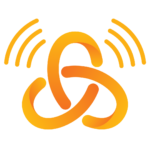
Description
Lily Clements and David Stern discuss the intersection of AI and international development, particularly in low-resource environments. David reflects on the critical, yet often overlooked, role AI could play in aiding smallholder farmers in regions like West Africa. They consider the potential of open-source AI, the ethical issues around commercially driven AI apps, and the significant yet underutilised impact of established AI technologies on international development.
[00:00:02] Lily: Hello, and welcome to the IDEMS podcast. I’m Lily Clements, a Data Scientist, and I’m here with David Stern, a founding director of IDEMS. Hi, David.
[00:00:14] David: Hi Lily, we’re discussing AI in international development and the implications.
[00:00:21] Lily: Something which I’m very interested in, of course, working in international development and being interested in AI, but I don’t know how the two cross over. So I’m really interested to hear your thoughts.
[00:00:33] David: I was in a forum with funders and the head of a foundation, we were there related to agroecology and working with smallholder farmers in very low resource environments.
[00:00:45] Lily: And when was this, sorry?
[00:00:47] David: A year and a bit ago.
[00:00:48] Lily: Okay, cool. So since the kind of…
[00:00:50] David: Since the explosion of AI, so this is relatively recent. And she was joking that, okay, everyone’s talking about AI, but I work in West Africa, Niger, Burkina Faso, Mali, with some of the poorest people in the world, farmers struggling to get by, and she said, you’re a farmer in a low resource environment, what do you care about AI? This isn’t important for you. We need to focus on what’s important for the farmers.
And nobody reacted. And so I reacted. And I said, just because you’re in a low resource environment, you know, you need AI as much as the next person to identify the pests that are coming on to your crops, to understand if your plant is diseased, you want to be able to have AI to identify it for you.
[00:01:38] Lily: How can AI identify that?
[00:01:41] David: You take a photo and it identifies the image. If you’ve got a bank of images, which have different diseases, and you have the learning algorithms to do this, this is well established. This isn’t new research in AI. These are standard AI processes in image processing which are used for identifying plants, diseases, insects, they’re well established. But they don’t work in low resource environments because people don’t have the data banks, the image banks to train them on.
And this is the thing, that the people who need it the most for whom actually being able to quickly identify a pest or a disease might really enable them to save their livelihood. It doesn’t work for them because it’s not trained, the images don’t exist to train it in their environments. And so, I sort of very quickly responded to say that, ‘No! Don’t forget about the value it’s bringing, things that we take for granted’. I’ve mentioned before that my aunt just leaves her phone out to listen to the birds sing to know which birds are in her garden.
It’s such a powerful tool now, in ways that are very specific. We’re not talking, or I’m not talking here necessarily about what I would consider as cutting edge AI advances. A lot of these things, yes, they’re improving all the time, but they’ve been around for a long time before the big AI boom happened. Or the latest AI boom happened, many of them.
But they are so powerful and they’re so important, especially in low resource environments for certain tasks that in my mind, it’s tragic that people aren’t doing the work to make them work. This is hard. It’s hard to get the contextualized image banks in lots and lots of low resource environments to be able to do the identification process and actually get those algorithms working well, where those farmers are.
They’re trained in environments, which are higher resource environments, generally. I have to say there are specific countries like India who are doing a lot. They are really doing a lot to create these image banks to be able to get these systems working. They’ve just got the human infrastructure and there’s lots and lots of instances of using the existing algorithms really well coming out of India right now.
But they’re often done in closed models and commercial models, which don’t adapt well to all other environments.
[00:04:44] Lily: With this example, though, the first thing that strikes me is who’s going to fund this? Who’s going to get the images? Or is that the kind of general question? So what’s in it for…
[00:04:56] David: Well, this is the point. At the moment, AI is seen as this huge commercial opportunity first and foremost. Whereas these instances, of course, if you take a farmer in Niger, then they’re not a sensible market. When you’re living in absolute poverty or less than 2 a day, there’s only so much you can extract from them.
There’s not that many of them. There’s a total population of tens of millions, 20 something million, you don’t have a big market. This is one of the lowest GDPs in the world. So there isn’t much to be made if you take it from a commercial perspective.
However, it is something where there’s an increasing number of students, of infrastructure, universities, and others, where the human infrastructure to contribute to these is actually there. And it wouldn’t be that expensive to tap into those in ways which are locally adapted.
I come back to Niger, Burkina Faso and Mali and they are very interesting countries because they are looking for a lot of independence and sovereignty at the moment. So they’re wanting their own ways of doing this. We’re in touch with researchers there who would love to be able to figure out how to get their students to be building these image banks and so on.
And it’s something which, of course, it costs, somebody would need to pay for it. But it’s not necessarily something which, well, let me rephrase that. I think it’s something which is so eminently cost effective, because these tools, if they are built right, using open source approaches which are established, and they are in competition with some of the commercial models, but if it’s done well with open source tools, then there’s no reason why this is something which is tied to that context.
What you build for that context could have applications way beyond that context, which means that it could eventually become sustainable and independently sustainable, because it enters into competition eventually with established commercial models on this. And potentially, as we’ve seen in other cases, these open source solutions may outcompete.
But if we start by building some of these and thinking about them from this really low resource environment, then that might mean, yes, it needs grant funding to kick start, yes, it’s not going to have venture capital behind it because it’s not looking to rapidly get the return on investment. But if these things are built for and in those environments with a vision to go beyond, then I think that this could actually be an interesting way to gain momentum behind some of these open source AI tools.
There’s a big AI summit in France as we speak, which is broadly trying to say the EU needs an alternative model to be able to get in on this. And they’re very much looking at these societal good uses of AI. They’re looking at open source. They’re trying to think about how these models are going to work. There’s other discussions we can have about a recent Chinese open source AI model which is out competing the big tech on this and caused a bit of a crash in share prices.
[00:08:48] Lily: Yes.
[00:08:49] David: That’s a whole nother episode, but there’s a lot happening in the world where actually, if you step back from this and you say, okay, let’s look at the lowest resource environments, because there you don’t have a commercial model and let’s not try to find a commercial model because you don’t want to exploit the poorest people in the world. So let’s look at models to actually build solutions for them in cost effective ways using local talent, building in ways which are responsible and actually focusing on the social impact.
Maybe those approaches will then be useful and could be reused in other contexts. But because you don’t have the commercial aspect from the start, you can focus on the social impact, it means that you don’t get pulled in different directions. You can focus on actually building the tools that are really needed, cost effectively.
So that’s part of a hypothesis I have on this. And there’s a lot of danger, of course, of using AI irresponsibly in these environments, but there are certain things which it protects you from. Let me give you a couple of quick instances.
[00:10:20] Lily: Okay.
[00:10:22] David: So if you’re building for a high resource environment, then automatically you want the best. You often would want access to the service who can do the calculations, the big calculations on demand when you need it. If you’re looking at low resource environments and you want something which is cost effective, you want something which is functional, you focus on the functionality.
You might not have access to the internet, so you want something which can work offline. If you’re working offline, you can’t have big models behind it.
[00:10:55] Lily: Yeah.
[00:10:55] David: You could have big models behind it, which are used occasionally in the results of those models, and they’re sent down to smaller models, which can run on a device. And these are the sort of things where you can actually get a better interplay, which of course means you’re using less resources, so it’s more environmentally friendly.
You’re doing it in a way which it is good enough. If it’s not good enough, then it’s not good enough and you need to improve it. But you should be building things which are good enough to be functional. And if you can identify the pests and the diseases that you need but then you find something and it can’t identify it or you fear that it’s not identifying correctly and then you send that up to the model and it goes into a queue and it does that not on demand but it takes time and there’s actually a process behind it, maybe even a process which involves humans.
These are different approaches which I think could lead us to designing these AI tools differently. And my hope is that the design of those AI tools, because it’s focusing on them being impactful, them being good enough within the constraints of the environment, I believe that there’s real potential to re imagine the AI you’re building.
And what I want to come back to very simply is, I’m not necessarily talking about cutting edge AI at this point in time, because actually, yes, as a mathematician, I’m really excited by the latest advances, there’s interesting things happening at that cutting edge.
But actually, that’s not necessarily what’s needed. In fact, I don’t think that’s, I think the tools that already exist are enough to be able to build these powerful solutions. And they’ve been proven to work in certain contexts. But it’s the human infrastructure to build them across contexts. It’s the social structures to be able to support ongoing development and so on. That’s what we don’t know how to do at scale, I would argue.
[00:13:16] Lily: But, I mean, like, more and different kind of generative AI models and AI models coming out, having that diversity is allowing for, I guess, like optimisation in a way. For example, you mentioned DeepSeek briefly, my understanding is that that one’s a lot cheaper to run.
[00:13:32] David: Absolutely, and I don’t want to get distracted by that, yes.
[00:13:36] Lily: Sorry.
[00:13:37] David: But yes, DeepSeek is an incredibly interesting advance. That’s a whole other discussion.
[00:13:42] Lily: Yes. But should we focus on the models that we do have?
[00:13:46] David: No, I’m saying different people can do different things.
[00:13:49] Lily: Okay.
[00:13:50] David: What I’m saying is, if we’re looking for AI applications for international development, we don’t need to be at the cutting edge. There’s so many applications which can use the existing tools. Even before generative AI, the sort of identification, classification of species or plants or diseases or this sort of thing, which I keep coming back to, these are old fashioned AI models now, which is great to see. But they’re still not being used as they need to.
So I’ll give you one very explicit example, what I considered the best app to identify pests and diseases was brought up by a pesticide company.
[00:14:42] Lily: Okay.
[00:14:42] David: Because the business model is that once you’ve identified the pest or disease, they sell you the pesticide.
[00:14:48] Lily: Okay. Yep.
[00:14:50] David: And that’s highly profitable for them. But in an environment where you don’t have access to pesticides, where there’s no real market, if you go to the context where that’s not really feasible, of course, that is not their priority. And so it isn’t being improved or developed in these really low resource environments because they’re not a market.
Now, more than that, actually, we’re involved in agroecology and there is an ethical issue for me with the technology of identifying pests and diseases to be tied in with a pesticide company when there’s actually a lot of evidence that pesticides are actually not necessarily the best way to treat pests and diseases in a lot of different cases.
There’s been some of the modelling work we’ve been involved in have shown that they saved I’ve forgotten exactly what it was, but it was billions of dollars in the U. S. when they demonstrated that they were using pesticide unnecessarily because the pest they were trying to treat for, didn’t actually reduce yield. And actually letting the pest eat a few buds, there were more than enough buds to go around. The plant grew healthily anyway.
And so you didn’t need to treat for that pest. And so they were able to save billions of dollars, reduce the damage to the environment, and not compromise on yield or the healthiness of the plants. And so there are big issues which are recognised with the use of pesticides in ways which are not always needed.
Now I’m not saying pesticides are never useful or never needed, but what I am saying is that you have a conflict of interest if the app identifying the pests and diseases is owned by the pesticide company. That to me is a conflict of interest, you know, because of course they will want to point you towards the fact, oh, you should use our products. And that’s just fundamentally not good for society.
I would argue that we should have structures, be they academic structures, be they social structures, that hold and use the technology to identify the pests and diseases and gives a whole range of different things and the latest knowledge, and maybe there can be ongoing research on the best things to do.
And so that discussion of what feedback you should get when you identify a pest or disease, that’s probably an academic problem. This is about experts. This is about knowledge. So really, we want something which is tied in with some of our academic systems, our knowledge creation, which can give a balanced view and where you can have different opinions coming in and giving whatever the latest state of knowledge is about that.
Now the systems to build such a system, I would argue in Europe or the UK, these could happen, but it’s gonna be hard because it’s so expensive to go up against those who have a vested commercial interest. Whereas you do this in an environment where they have a flourishing academic community, which is starting to sort of come out, I come back to Niger, one of my favourite examples, but when I was there not so long ago, there was one university in the country.
Now every region has a university and, you know, academics, flourishing in all sorts of areas. You’ve got a really strong set of students coming through in different ways who can build careers actually doing this. So you could actually build them into the system of actually what the AI would be feeding into, no, it’s not what the AI is feeding into, because the AI identification, that needs the images and so on, but it’s actually then what happens once you’ve identified. They can be taking responsibility for that. And we have academic partners in Niger, Burkina Faso and Mali, who are building that knowledge in really powerful ways.
So thinking about this is something where those structures have built out and tested in an environment where the commercial companies are not wanting to compete because there is no market, I think could give the opportunity to think about alternative models to building socially impactful AI.
That’s what’s so exciting. It’s not that in low resource environments, the need for AI is any different really from the need for AI internationally. It’s that the incentives behind AI are totally different because they don’t have the same commercial markets. And that’s one of the ways to see it. Anyway.
[00:19:57] Lily: No, and that’s a very exciting way to see it as well that actually there’s this huge opportunity. But I guess my immediate thoughts is that AI is moving fast and it’s moving really fast. And so what’s to say that in a few years time, or like you said, suddenly there’s all these universities popping up in Niger, so what’s to say why now? Maybe in a few years time it will be easier to implement these things when…
[00:20:21] David: Is AI moving fast?
[00:20:25] Lily: It feels like it is.
[00:20:26] David: Ah, that I agree. It feels like AI is moving fast. I claim that the AI advances that I think are really needed, all happened in the 90s. It’s taken more than 30 years for them to actually come to fruition in a way where now we could think about using them at scale in low resource environments and so on.
There’s a lot of hype about AI moving fast at the moment, and it is moving extremely fast at very specific things. The generative AI and the advances in generative AI on language, on image generation, on these sorts of things, deep fakes were in the news again, because the French president actually used deep fakes as part of the advertising for this AI summit.
These technologies, they are changing really rapidly and the applications of these generative AI technologies are coming out all the time and that does give them feeling of movement. So there are lots of things in our societies where it is true our societies are reacting and having to react to AI fast now because there was a tipping point.
And that tipping point broadly was, there’s different ways to see it, but it’s when the ability of generative AI to feel creative, that was a tipping point in how people could use it. And when you can’t tell the difference easily between something which is AI generated and human generated, that was a sort of tipping point, the Turing test, but anyway. So yes, that was a tipping point. But that’s not all that AI is.
[00:22:13] Lily: Yes.
[00:22:13] David: And the technologies behind AI have been around for a long time, they’re now pretty well established, they’re stable, almost. Actually using some of those, rather than necessarily jumping onto the sort of generative AI stuff, which is advancing, I don’t dispute that, but it’s not necessarily what is most useful in these low resource environments, especially because it’s language dependent.
And if you’re in a context where your language is a minority language, there’s still a long way to go.
[00:22:50] Lily: A very good point as well. I guess it’s very easy to conflict or to jump to generative AI and forget about all the other existences of AI, or other uses of AI.
[00:23:02] David: Yeah.
[00:23:03] Lily: Other AIs.
[00:23:05] David: Absolutely. And that’s the narrative which has taken over. But it’s not necessarily what’s needed. If we’re really looking at how we could use AI to positively influence society, then actually, stepping back, understanding what are the problems which are easily solvable with AI now, and how do we solve them?
This is the thing. We know identification is something that it does brilliantly. So what are the identification problems that we’ve only partially solved?
[00:23:42] Lily: Yes. Yeah. No, absolutely. Okay. I’m convinced [laughs]. And so AI in international development, you’re referring to these sorts of things where actually, as you said, it’s stuff that was established back in the nineties. All those big advancements were around 30 years ago and it’s very well established now. And we can make those changes, applications, those enhancements in the kind of international development.
[00:24:13] David: I think there’s a lot of problems that people are facing in international development in different ways, there are ways in which AI can come in and help more than I think I fully appreciated until somebody said, of course, AI is of no use to people in low resource environments. That’s obviously false.
But actually thinking about well, okay, what are the ways, if we forget about the hype and we come back to things which are eminently doable now, how do we build solutions that really take advantage of this and use that? And it’s not easy, this is the thing, good technology, building good technological solutions is never easy, and cheap, that’s another point. But it is something where these are solvable problems, this is a step change, it doesn’t solve the bigger problem of pests and diseases. But it does say that the identification of the pest as a problem, that is solvable.
We can have systems everywhere in the world which can identify pests and diseases. That is a solvable problem. The Gates Foundation years ago wanted to eradicate polio. It was a solvable problem. It’s not an easy problem to solve, but it was a solvable problem. Taking a single disease and actually eradicating it, that was a solvable problem. And I feel it’s the same, we can look differently at how we use technology to just solve components of a problem. Anyway.
[00:25:48] Lily: No, incredibly interesting. And thank you very much, David. Did you have any final thoughts?
[00:25:51] David: I guess we will be having further discussions about DeepSeek and all sorts of other exciting advances coming along. And this is an ongoing, I guess it’s an ongoing discussion. I think we might even want to do another episode where we look at this more specifically for education.
[00:26:09] Lily: Yeah.
[00:26:10] David: Because that’s a really exciting area, where I think there’s a lot coming, but there’s actually dangers. We haven’t gone into the dangers so much on this occasion.
[00:26:26] Lily: It’s hard to in half an hour, but thank you very much.
[00:26:28] David: This discussion will continue.
[00:26:30] Lily: Yes. Thank you.
[00:26:32] David: Thanks.