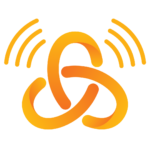
Episode Description
The first episode of The IDEMS Podcasts. Its title, “Maths Minds: Thinking hard about supporting a collaborative society”, represents some of what IDEMS does and how we intend to tell our stories. Dr Lily Clements interviews David Stern, a co-founder of IDEMS, to delve deep into elements of why IDEMS exists.
[00:00:00] Lily: Hello, and welcome to the items podcast. I’m Lily Clements, an impact activation fellow, and I’m here with David Stern, a founding director of IDEMS. Hello, David.
[00:00:11] David: Hi, Lily. Our first podcast. What are we doing?
[00:00:15] Lily: So today I wanted to talk about this kind of tagline that we have, this Maths Minds: Thinking Hard About Supporting a Collaborative Society.
This is our first podcast, and let’s start by a discussion on why this name, so I guess maybe starting with the Maths Minds?
[00:00:34] David: Yeah, and maybe just starting with why a podcast series anyway. So, I mean, this podcast has sort of come about because I can’t write, so I can’t do a blog, but more generally that we as a sort of Community now in IDEMS have got stories to tell, which we’re not very good at telling.
And, and so the idea is to actually get these stories out there, because when we tell them face to face, people want to hear these, and we’re not getting them out. And so,the idea is that as an organisation, as IDEMS, we want to get our stories out and share these with people in ways which we hope will be useful.
[00:01:18] Lily: And I also feel that a lot of the times when you speak, you say the stories in such a way that if I was to repeat them, it still wouldn’t come out the same way. You want to hear the stories from that person themselves.
[00:01:30] David: And, and unfortunately that’s why, at least initially, you’re going to be hearing me speaking quite a lot because I’ve got a lot of stories to tell, because we’ve been going quite a long time.
And over time, the intention is for the IDEMS podcast to, for other members of IDEMS to get comfortable telling their stories much more. So that’s the aim. You know, as we grow into this, this becomes a real mechanism for many people within IDEMS to tell their stories. But I apologise. You’ll be hearing quite a lot from me over the next few months.
[00:02:02] Lily: Well, we’ll see how that goes.
[00:02:05] David: People will get bored eventually. But, I think the important thing is, and this is where the subtitle or the sort of catchphrase is so important, it’s the fact that as an organisation, you know, we are mathematical minds at the heart of it. This was founded by mathematicians.
I was a maths lecturer. I, taught mathematics at a university. I was a mathematician before becoming a statistics educator, before becoming a data scientist, before becoming a research method specialist, but always within the mathematical sciences and applying that for the last 15 years that in international development, in social impact, in trying to actually move the dial on societal issues,not just doing this, as I did initially, for the beauty of the subject. I love mathematics, it’s beautiful. When you have no applications, it was great. But, you know, having the mathematical minds applied to things of social importance, I think, is really important. And that’s the mathematical minds part of the subtitle.
[00:03:15] Lily: But, but what about other minds? I mean, in IDEMS we’re not all mathematical scientists. We need, we need…
[00:03:21] David: No. And there’s this in society, there’s this big issue that actually mathematical minds are overvalued. They are underrepresented in areas: the charitable sector, international development, and so there’s areas where they’re underrepresented and one of the reasons they’re underrepresented is because they’re overvalued in finance, in business, and so on, and that’s why we have a society where it’s very expensive to get mathematical minds to work on social issues because you can earn a lot going into a bank with a maths PhD.
I mean, this is something where, you know, you’re in demand, you have skills which are really valued. And I think a big part of this is actually, if you’re not looking at, and I’m going to jump to the collaborative society bit, but if you’re not looking at a collaborative society, but you’re looking at a competitive society, math’s minds are really good at competition.
You know, actually figuring out logically what’s going on, understanding, you know, the theories behind it, getting rid of the noise, and just understanding, you know, how to make a profit. You know, this is the sort of things that maths minds do really well. In general, maths minds, because they’re good at reducing the complexity down to something they can solve, they don’t tend to deal with complexity well.
And this is one of the things which is so important in our society. We’re seeing all the negative impacts which come from as a society having single indicators of success. As countries, you know, everything being around GDP growth, well, there’s good evidence that that’s not what builds a good society.
You need to be thinking about society growth in a multidimensional way. The sustainable development goals, these are multidimensional. And there’s good reasons behind that. And one of the things we recognise as an organisation is that while we employ and while we bring in mathematical minds, from day one, when we bring people in, we help them to recognise that mathematical intelligence is not more important than the other intelligences.
There are many other intelligences more important than the mathematical intelligence.
[00:05:47] Lily: So why, so in this case, you’re saying we’re focusing on the maths minds. And so what is it that the maths minds can bring? Because you said that they can’t, they aren’t good at dealing with complexity.
So they’re clearly not bringing much there.
[00:06:00] David: So, so no, I didn’t say they’re not good at dealing with complexity. This is, this is one of the contradictions. You know, what they’re really good at is getting to simplicity.
[00:06:07] Lily: Okay.
[00:06:08 David: So, so they often avoid complexity. They just sort of get rid of the noise, the complexity, and just say, well, okay, this is simple. This is just this. And that’s what they’re really good at. And, and, you know, it’s one of those things that as, as a mathematician, you know, what you tend to do is you tend to make sure that what you’re working on is extremely well defined and precise. And you can logically follow the argument. Yeah, that’s what you’re trained to do.
This is what we do really well. And so we’re really good at logic. We’re really good at precision. We’re really good at actually getting a precise context and working it through to understand what the implications will be and what would logically follow. But, for example, an intelligence which I value immensely and have had to work at because I don’t have it naturally is an emotional intelligence.
You know, actually understanding the value, for example, one of my favourite examples of this is you know, if you treat all people the same. You know, many mathematical models, you sort of work with differential equations. So if you have differential equations, you assume a homogeneous population.
A homogeneous population means everyone is the same.
[00:07:33] Lily: Okay.
[00:07:34] David: Not my experience. Everyone’s different. So accepting that there are differences between people means that you need to accept there’s different motivations. You need an emotional intelligence to understand how to motivate other people.
[00:07:52] Lily: well, and this is, I guess later in the podcast series, we’ll have the likes of Lucie, who’s an anthropologist.
[00:07:58] David: Absolutely.
[00:07:58] Lily: I mean, and she, she deals with, with these kind of more complex ideas.
[00:08:06] David: And this is where mathematicians are really good with complexity, as long as it’s well defined. Whereas anthropologists, they work brilliantly when things aren’t well defined, because they can’t, they know you can’t define these well, there’s all this complexity you cannot define, you can’t figure them out.
So it’s a different nature, and valuing that human element is so important.
[00:08:31] Lily: Okay. Okay. So should we, are you ready to move on to the thinking hard?
[00:08:33] David: Yes.
[00:08:34] Lily: Okay. So maths minds, thinking hard. So why not, why not thinking?
[00:08:42] David: Well, I mean, maths minds tend to think that’s what we do, but most of the time we tend to try and simplify.
[00:08:50] Lily: And also just the statistician in me at this point wants to say maths minds being mathematical scientists.
[00:08:56] David: Absolutely.
[00:08:57] Lily: not that I mind very much about being called a mathematician. I don’t mind what you call me, but I know some
[00:09:03] David: Many statisticians do. Many statisticians are very clear that they are not mathematicians and they think differently.
And actually many statisticians are, you know, they’re used to sort of being on this boundary where things are less well defined. They deal with variability. I love the fact that, you know, people make this big difference between mathematicians and statisticians in all sorts of different ways. But if you come down to the modelling, you know, thanks to Bayesian statistics, the difference between mathematical models and statistical models is just an error term that in stats you worry about the variability and in maths you assume that everything works on average. So obviously statistical models are better.
[00:09:46] Lily: Thank you!
[00:09:47] David: Mathematical models are missing out on the most important aspect; as in the variability which exists within everything we do.
But at the heart of all of this, then, is sort of, well, where does variability exist? And so, so, you know, statisticians have it much harder, if you want. And this is where your mathematical sciences… There’s so many different layers to it, you know, there’s your computer scientists where they’re, they’re sort of much more on the end of sort of getting towards building things, understanding algorithms, you know, understanding how to work with big data.
I love the fact that computer scientists have captured data science from statisticians. So, you know, just like mathematicians are horrified if you call, sorry, statisticians are horrified if you call them a mathematician, data scientists would be horrified if you called them a statistician.
[00:10:34] Lily: Oh, yeah!
[00:10:35] David: They’re not a statistician. No, they’re a data scientist. They don’t work with statistical methods. No, no, no. They’ve liberated themselves from that using computing. And, and so they now work on big data, and they use machine learning and artificial intelligence. And so, and we’re going to go into that in, in…
[00:10:55] Lily: Yes, yeah, I was going to say, I think the variability and all of this and responsible AI…
[00:11:00] David: This is going to come out beautifully in the future. But I think… I shouldn’t leave out theoretical physicists, of course, you know, there’s an important distinction in the mathematical scientists, you know, an experimental physicist would not be considered a mathematical scientist because they need a lab, they need to work with experiments, whereas a theoretical physicist would be considered as part of the mathematical scientists. And they’re brilliant because… They bring in the real world. You know, mathematicians, you know, we can abstract out many things, whereas theoretical physicists, you know, despite the fact that they’re theoretical, they are trying to understand the real world using the same tools. I mean, you know, so the mathematical sciences as a whole is really important. But to get back to this thinking hard.
[00:11:50] Lily: Yeah, sorry. Sorry for diverting us.
[00:11:54] David: Diversions are good. To get back to the thinking hard. It doesn’t matter where you are in that space. By and large, our general approach is to be lazy. You know, any good mathematical scientist tends to want to be lazy and to do the simplest thing possible. In mathematical modelling, there’s this whole thing about actually doing the simplest model possible to answer the questions. You know, this is an unnatural instinct. So thinking, we do very well. But naturally, as a good mathematician or a good mathematical scientist, we want to be lazy and think as simply as possible.
But we’re saying, no, we don’t want to just think. We want to think hard. We want to think deeply. We want to go beyond the easy solution to look at complexity. Because that’s the nature of how we can serve more broadly.
[00:12:50] Lily: And thinking hard doesn’t mean thinking longer. It doesn’t mean thinking you’re sitting on something. It means, I assume, just from experience in my PhD, it means you’re digging deeper and deeper into the problem, which might mean spending longer on it, but it doesn’t necessarily mean…
[00:13:11] David: Yes, I think that that’s absolutely right, that it definitely it’s, well, it does relate to this Nobel Prize on thinking fast, thinking slow.
We are not talking about thinking fast, we’re not talking about sort of being able to have your good instincts. That’s what thinking fast does. We’re talking about thinking slow. We’re talking about reflecting deeply.
[00:13:39] Lily: Sure.
[00:13:39] David: We’re talking about considering complexity.
[00:13:42] Lily: Yes. And is that the Thinking Hard? Shall we move on to the…
[00:13:47] David: That’s the Thinking Hard. Let’s go to the collaborative society?
[00:13:50] Lily: The Collaborative Society then. So okay. Maths Minds: Thinking Hard about Supporting a Collaborative Society. Let’s go on to Collaborative Society.
[00:14:00] David: So the collaborative society is really important because at the moment a lot of our society is built around competition. Companies compete you know, for resources. Countries compete for resources. But even within competition, it has been shown that collaboration can outcompete competitiveness.
There’s some really wonderful biological research going back a long time, you know The Selfish Gene (by Richard Dawkins) is a really nice popular expression of this, which is really old now. It shows how old I am. But, you know, this is, this is a very simple way where in biology they used a bit of the maths to then demonstrate why actually being collaborative can outcompete in a number of contexts.
So, you know, it actually showed, you know, in their context they had a nice chapter called Good Guys Finish First, which when I was young and growing up, this appealed to me in many different ways. And so this idea that actually by being collaborative, you can outcompete. Now you can’t outcompete in all contexts, and actually the society we have created at the moment doesn’t really support collaboration as much as I would like.
It sort of is assuming that there is a limited resource, in, in different ways. And we do live in a world where there are limited resources. And of course, when there are limited resources, competition for those resources is natural, but there are other ways in which our resources aren’t limited.
And when you’re in that context, then collaboration can actually perform better than competition in certain ways.
[00:15:48] Lily: So how can we, if we have competition in there, how can we have collaborative working effectively if you’ve got people that are competing in the mix?
[00:15:59] David: Well, all you need for that to happen in evolutionary terms, is that the people who are collaborating outperform the people who are competing.
It’s as simple as that. Mathematically, that’s all it corresponds to. It’s a question of, within this, you know, who performs better. And actually there’s more and more evidence around that people who collaborate well do tend to perform better even in a competitive environment. And so understanding how to collaborate and when to collaborate.
Now that doesn’t mean that, you know competitive practices won’t win out in certain cases. McDonald’s is one of my favourite examples of a really sneaky competitive practice where some people thought they were building a burger company, whereas other people realised they were actually building a property company.
[00:16:52] Lily: Okay.
[00:16:53] David: And then stole the whole concept. So the original McDonald was totally screwed out of McDonald’s and couldn’t even use his own name for his restaurant because people realised that the value in the company was in the property and not the burgers and therefore… anyway that’s a whole different story.
There are concrete examples of where competition in these ways has won out against sort of a more collaborative approach. But there are plenty of other examples where the collaborative approach has achieved more. And so we believe in these collaborative approaches and, and in enabling a society which supports them.
And that’s where we think that, in the sort of crises we face at the moment, collaboration is essential to us actually building the society we want, that we’ll be able to rise to the challenges of the future. It doesn’t matter whether this is the economic challenges which we’re facing across the world, it doesn’t matter whether this is the future health challenges which COVID 19 has sort of shown will be coming. Or whether this is the climate crisis. There’s so many different crises our society are facing at the moment.
Actually, these are, I believe, times where collaboration can really win out and can really outcompete competitive models. And so we’re looking to support that collaborative society. And this is, you know, about supporting.
[00:18:34] Lily: Yeah.
[00:18:35] David: We cannot achieve that. Mathematical minds are ill placed to conceive what a collaborative society of the future will look like. You need emotional intelligence in the bucket loads to do that. You need all sorts of things we don’t have necessarily. I’m very conscious that when I walk into almost every room,whatever the context, I am, by some measure, both the smartest and the dumbest person in that room. And so, recognizing that collaborative society requires intelligences I don’t have, but that my intelligence can contribute to it, I think that my role is to support the people who are trying to build that world, who are trying to sort of create that future.
And, and so our role is… You know, we’re good at competition. This is what comes naturally to us, you know. I’ve been, since I was young, I liked to win. Like, so I feel that’s a bit of a confession, really. I sort of, I got quite good at winning in a lot of different cases. I used to play volleyball before, when I was a little younger, and I loved it, and I actually got quite good at it.
And I used to find, I had my one, one season of great success. Yeah, locally, you know, I played more seriously. I played in the National League in England and so on, which is a really low standard if anyone internationally knows about volleyball, but, I was coaching a team in a local league, and we were not the strongest team in the league, and we lost two games, one in 3-0 and the other one 3-1.
Every other game that season, went to five sets and we won all of them because I was really, really good at the psychology of winning and actually how to get the sort of substitutions to do the coaching right, so that even though we didn’t have the best team, if we went the distance, we had the psychological advantage at the end that we won.
Yeah. And we won the league. Now I’m quite proud of that, but it’s, it was not because we had the best team. It was because as a coach, I was able to get the best out of my players at the right point in time, and have momentum with me to win the match in that crucial fifth set for all but two games of the season.
[???] Lily: Yeah.
[???] David: And in the two games of the season we didn’t get to the fifth set. But if we had, we’d probably have won it. Because I got the psychology. So, it was, you know, this is, you know, I’m, I like competition, I’m good at this. This is natural, the mathematical mind of thinking about these things, being able to take complexity and turn it into the sort of simple thing of actually…
Well, how can I make substitutions which will increase our team’s motivation while you’re motivating the other team and all of this? That was something I was quite good at. And naturally, mathematical minds, we can be pretty ruthless, you know. We can be really good at competition. And so our role is not to build that collaborative society because that’s not how our minds think.
Our role is to support others, to recognize the value of that society, to understand how to build it in such a way that it can outcompete others. Because I believe it can. And that’s one of the things which has been shown in time and time again in biology and in other areas, that actually collaboration done well, with the right depth of thought behind it, can outcompete.
And that’s what we’re about. I’ll just give one last example of that, which is my favourite, is my inspiration. Okay. You know, if the guys behind this, ever listened to this, you know, I admire what you did. Red Hat.
[00:22:33] Lily: I knew it was going to be Red Hat.
[00:22:34] David: You knew it was going to be Red Hat. These guys are amazing. They decided when Microsoft was the biggest, the richest company in the world, making its money out of proprietary operating systems, they decided that it would be a good idea to build a company around offering a free operating system and they succeeded. They over, roughly a 20 year period, they built a company which turned over a billion a quarter based on an open source free operating system, Linux, and then, you know, it was incredible, not just the way they, not just what they did, but the way they did it.
And then, of course, they got bought out by IBM, they all became rich, and all the innovation they’d built was, was lost, but that’s a separate story. But the fact that they demonstrated that this collaborative, you know, everybody knew about open source way back when they started and people liked it in theory.
[???] Lily: Yeah.
[00:23:46] David: But after they’d done that, people saw, no, this out competes proprietary.
[00:23:46] Lily: Okay.
[00:23:46] David: As a way to develop software. And now, you know, all the big software companies develop a lot of their software as open source because it’s better for business. And that’s a really beautiful example of collaboration winning out if it’s done right.
So, hats off to you, Red Hat and that’s part of our inspiration.
[00:24:08] Lily: Interesting. Okay, great. So could you just summarize in one or two sentences Maths Minds Thinking Hard About Supporting a Collaborative Society?
[00:24:20] David: This really underlines what IDEMS is. This is about the fact that, as mathematical scientists, we should be in service of the society that we want to see.
If we want to be the change we want to see in the world, we need to recognize that it’s not about us. It’s about the mathematical mind serving others.
[00:24:48] Lily: Wonderful. Well, thank you very much, David, and I really look forward to continuing conversations in this series with you.
[00:24:55] David: Thank you. I look forward to it, too.