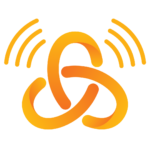
Description
In this follow-up episode, Lucie and David discuss data literacy from a more qualitative perspective, building on discussions between David and Lily in previous episodes. They explore themes like variability, uncertainty, and the value of different data types, highlighting the deep analysis of specific contexts versus the current rich data world. The conversation imagines a future where data literacy is as integral to society as traditional literacy.
[00:00:00] Lucie: Hi and welcome to the IDEMS podcast. My name is Lucie Hazelgrove Planel, I’m a Social Impact Scientist and I’m here today with David Stern, one of the founding directors of IDEMS. Hi David.
[00:00:18] David: Hi Lucie. You’ve requested this particular episode after listening to a previous episode.
[00:00:24] Lucie: Yes. So you had a discussion with Lily about data literacy.
[00:00:30] David: Yes.
[00:00:30] Lucie: And I love the use nowadays of literacy in these different contexts. I completely agree with it. I find it a useful concept. I really find it useful in terms of understanding someone’s ability to use something like data, someone’s ability to understand the data, to understand it in order to be able to use it. So I have to say, I find it a really important concept, and I was really interested to hear about it.
[00:00:55] David: However.
[00:00:57] Lucie: Yes!
[00:01:00] David: I see what you’re doing here! Go on, I look forward to the next bit.
[00:01:05] Lucie: What is data? What data were you talking about? What data is discussed when we talk about data literacy?
[00:01:11] David: As you know, I’m very big on not just quantitative data, but also qualitative data.
[00:01:17] Lucie: Yeah.
[00:01:17] David: And so my hope is always that when we talk about data, we should be touching on both. But I have to admit, sometimes I am guilty of not being enough of an anthropologist and recognizing when I do maybe think a bit narrowly. So I’m guessing that you caught elements where we didn’t represent more qualitative data as well as we should.
[00:01:38] Lucie: Yeah. And it’s also, I find it interesting that in anthropology when people are working more with people, more humanly with people, I would say, too, because medicine doesn’t always deal humanly with people, anthropologists don’t talk about data in the same way. They don’t talk about their data, they talk about their friends, or, they give the names of the people that they were working with, or pseudonyms in terms of anonymity. So I have a sort of question about how can we talk about data literacy, which includes these other attitudes towards data?
Also I just want..
[00:02:13] David: Can I just come in here on two things quickly? One, I couldn’t agree with you more, and I don’t know how to do this, so I’m really happy that you’ve picked this up and you’re challenging me on it.
[00:02:22] Lucie: I don’t mean to challenge, I want to have a conversation about it.
[00:02:25] David: I’m in the dark because I don’t understand what it is that was said that caught you wrong or that has led to this, or what was missing.
[00:02:33] Lucie: It’s not a challenge, it’s more a discussion, a follow up discussion, because there’s nothing in particular that was said, I’m not cross about it, but I find it interesting and I want to know more, yeah, I want to discuss how we can talk about data literacy in a way which is more open to different sorts of data.
[00:02:50] David: One of the things I think is really important is this different uses of data. You sometimes want data across a lot of people to know what is the general views on things. And other times, in the sort of cases you’re talking about, you want to go deep with individuals to be able to get hypotheses and to be able to draw out ideas, which have complexity within them.
And so both of these are valid forms of data, which is so valuable and so useful. We had a similar discussion on the CASAS work just recently, this is our modelling work, where the idea of actually building these models that represent the biological processes within the pests and diseases, there’s the question of how can you get useful data for that if you can’t get it at scale?
You have to do it with lab experiments. What you’re drawing on isn’t just important, and I think it’s really important to state this. This isn’t just an important discussion because of anthropologists wanting sort of individual data. This is also important in terms of the importance of lab studies, being able to get very precise data versus large scale data.
And, with the move towards big data, sometimes people forget the importance of precision and complexity. This is all important in data literacy and you’re absolutely right to draw it out in the context of qualitative data. But it is also there in other forms of data as well, the same need.
[00:04:20] Lucie: Yeah, but I do remember with Lily you discussed that we are in certain ways of putting it, we are in a sort of surplus of data, we live in a surplus of data. But I know other times you’ve mentioned exactly this, that while there’s richness in a huge quantity of data, there can also be huge richness in just one point of data, and that you can get many ideas from that or much understanding from that. And I didn’t feel that point came out in your discussion, which I think it should have done.
[00:04:49] David: And I’m so glad you’ve brought this up, and this is exactly where these episodes, I’m glad they are leading to this discussion. So yes, I think one of the things there which is so important is, and it rattles me a bit when I hear the experts in AI discussing full automation in different ways, where this importance of the individual data points, or data scenarios, in that diversity that’s created in different ways.
There are elements of this where this is very human in terms of societies, we actually want to build systems which can help to support the diversity of human experiences and individuals within the sort of idea of actually serving the masses rather than just making and treating everyone as the masses. That combination of individuality versus collectivity is so important and I find it very ironic that the people who are advocating for these big AI systems and so on are claiming to do so often in the case of individuality, when actually what they’re supporting is the collective voice.
And actually, it’s individuals, who are using their privilege of individuality, promoting something which is suppressing other people’s individuality in interesting ways. And it’s not suppressing it often because they’re willingly suppressing it, it’s suppressing it because they don’t understand well enough this balance and the difficulties between the different types of data, the importance of the small qualitative data, and how that needs to be treated and elevated at times, versus the big quantitative data at times. It’s not as simple as a, it’s never black and white on this.
[00:06:45] Lucie: And that ties into the other point I wanted to discuss, which is variability. Because, in working with statisticians, I have come to understand the last couple of years that one of the big problems is people not wanting to explore or not understanding that variability is an interesting thing and that we need to understand why there are different, I can’t think of an example.
[00:07:08] David: What the sources of variability are, how you can account for that variability.
[00:07:12] Lucie: Yeah and it’s something that isn’t talked about. It’s not talked about in the same way in qualitative research; it’s not in the ones that I’ve followed. I’m wondering if there’s something that can be improved in how qualitative researchers are trained in terms of thinking of it more in terms of variability?
For example, in working with people, you tend to want to try and find out if they’re saying it for a reason, or if they’re trying to manipulate the research or if there’s other reason why they’re saying it. Is the same idea of variability, is it just the context in which the person is saying it? I’m wondering if it’s useful even to talk about variability.
[00:07:50] David: But I think that what you are highlighting there is exactly that same search for the source of variability. If this person is saying it, what are the reasons behind why they’re saying it? Is it that everybody would be saying the same thing? Is it that they’re saying it and they’re saying it for a reason that you can understand? Or is it that they’re saying it because it’s what they believe and who they are, and they are different to who other people are?
[00:08:20] Lucie: Yeah.
[00:08:20] David: And that’s natural variability. What you’re describing is exactly how I would argue this deep data literacy around variability could help. And I want to be really clear. Variability is a hard concept for humans to understand. And I don’t think this is by chance. I think that there is something biological about how our brains work, where we’re looking for certainty.
There was this amazing event I went to a few years back by people who called themselves The Uncertainty Experts. And there’s a book on this and they run some trainings and the rest of it, but I think the essence behind what they said is that ability to actually be comfortable in uncertainty, this is a learned trait and actually a lot of successful people have it. That actually, it’s somehow very human to look for certainty. But the ability to be comfortable in the absence of certainty is like a superpower.
What do I mean by that? I don’t mean that you suddenly become Superman. It enables you to achieve more because actually we don’t live in a certain world in any shape or form. There’s always variability. Variability is all around us.
[00:09:44] Lucie: Yeah.
[00:09:45] David: But actually being able to understand, acknowledge that and work with that. So just as a very simple thing, let’s say you try something and it fails. Being able to determine, did it fail because you were unlucky, or did it fail because you did something wrong? That acceptance that it might just have been that, whatever, the timing wasn’t right, didn’t work. It was, try again and it will work, pick yourself up, dust yourself off and start all over again.
Or it could be that actually no, there’s a reason why it didn’t work. And that ability to recognise that, yeah, maybe you just need to pick yourself up, dust yourself off and start again, or maybe you need to actually reconsider how you do it. There could be different sources behind that and that ability to accept that uncertainty that it might not be that you did anything wrong or that the timing or it might just have been, you know, it didn’t work. Try again.
[00:10:43] Lucie: How does that relate to then the…? I think, if I remember correctly, in the data literacy episode, you’re also talking about how to encourage people to be more literate and understand how to work with data. It’s also about removing the sort of technical theory, but I don’t want to call them theories because the theories themselves are useful, but there’s some technicalities around the theories or the way they’re presented. I think you talked about significance testing, there we go, which I think a lot of people don’t really understand in its simplicity. So removing the sort of complexity of the name of it is something like, I can’t even remember, p tests or something.
[00:11:23] David: P values, yes.
[00:11:24] Lucie: There we go. So removing that sort of complexity and just thinking in terms of simple understandings of what it actually means. To me there was some sort of connection there again, in terms of this sort of certainty and uncertainty and then again, the sort of different types of data points and what you’re, what you’re trying to do. Not the different data points, sorry, the different types of data you might be working with.
[00:11:44] David: I think the example that I think you’re leading towards is we should be having people just having exposure to elements of qualitative data in such a way to build some of these instincts that you’re talking about, and then to be able to join the links between, okay if what I’m doing in these personal interviews is I’m getting that sort of deep, complex data, and that’s then when I need to question these different things.
Then you should be asking the same sort of questions of other data and vice versa. And somehow that sort of different types of data, data literacy should be about that complexity, that sort of substance behind it, not just one or the other.
[00:12:25] Lucie: Yeah, exactly. Exactly. I think there’s the same processes that go on in different types of data or different forms of data, but they’re explained differently in the different disciplines or something. And I think there’s a lot that can be improved there.
[00:12:38] David: I agree.
[00:12:39] Lucie: In both sides.
[00:12:41] David: And I love being taken to task in this way, because…
[00:12:44] Lucie: I don’t think I’m taking you to task.
[00:12:47] David: You should be doing so more. I feel I’m being taken to task.
[00:12:49] Lucie: I’m sorry.
[00:12:51] David: But no, let me be clear here. I’m sensitive to this, because I know that I come from a world of more quantitative than qualitative, and I really appreciate the importance of qualitative, both anthropological approaches, but also sociological approaches and so on, which are more qualitative in nature. And I’ve seen the value that they bring. But I do recognise that my language, I’m not coming from that world. I’m sensitive to that world, I’m impressed by many elements of that world, but I know that’s not where I’m coming from.
And so, it doesn’t surprise me that you as an anthropologist listening to the episode that I did with Lily says, um um, and it bristled a bit. And I cannot think back to what it would have been that would have done that or not. But it’s something that I’m sensitive I always have to be careful with because it doesn’t come naturally to me. It’s something which is a learnt trait rather than an inherent trait. I’m not a natural anthropologist. It’s something I admire, but I’ve had to learn.
[00:13:59] Lucie: No, but you have a lot of the skills, definitely.
[00:14:03] David: I might have mentioned this in previous episodes or not, but my sister studied anthropology and everything I learnt in terms of social skills I learnt from observing her.
[00:14:13] Lucie: Which is what an anthropologist would do though too.
Okay, so another sort of final question is also, you know, in terms of if we’re thinking of literacy in understanding data or in working with data, then I also just have a question in terms of the impacts or the potential impacts or the implications of findings. An awareness of that, can that also be included in the concept of literacy? Does that make sense?
[00:14:44] David: I think so, but I’d like you to repeat it for my own… it’d probably be useful for the listener as well, but I want to just make sure that basically I’ve understood. Go on, repeat it.
[00:14:55] Lucie: Okay, I’ll take a step back. If we’re thinking about literacy in the normal sense, in terms of reading and writing, actually I’m not sure, does it include writing? I can’t remember, it does. Then a very basic or simple level of literacy is to be able to read something and to perhaps be able to understand what you’re reading. I don’t know if it means that you have to understand the implications of what you’ve just read.
[00:15:20] David: Yes, I hear what you’re saying, and I hear the parallel for data literacy.
[00:15:25] Lucie: Here I’m talking about a higher level of literacy.
[00:15:28] David: But already the fact that within literacy we would implicitly include understanding, and understanding does imply, in the context of data at least, understanding of implications around, validity and this sort of thing. So I think the fact that you use the word understanding what you read…
[00:15:46] Lucie: Yep.
[00:15:46] David: …which I think nobody would dispute is an element of literacy. And I’ve heard people talk about data literacy in many different ways, and most people I know who think about this quite deeply, don’t consider this as a bar you jump over. They consider it as a journey you are on.
[00:16:03] Lucie: Absolutely.
[00:16:04] David: And I still remember, if I think about my own actual literacy, I was very fortunate I was in the French system at school and very near the end of school I finally understood why literature was interesting. If I’d gone through the British system, I’d have done double maths, physics, chemistry at A level, and I wouldn’t have had the same breadth.
But studying what was essentially French literature at the equivalent of A level, I suppose it was the equivalent of AS level because it wasn’t my major subject, that’s when it suddenly clicked to me as to why this is important and why this is interesting. That ability to understand, not just to read, but to understand what’s written and what’s not written.
[00:16:49] Lucie: Exactly, and that comes in, you know, so you were, up until sort of, you’re 15 or something, you were literate, but you didn’t have that additional understanding.
[00:16:57] David: And I do like the way of thinking about this, it’s not just understanding what is there, but also being able to read between the lines a little bit.
[00:17:06] Lucie: Yeah.
[00:17:06] David: And the same is true of data. High levels of data literacy, you’ve got to be able to read between the lines of what’s not there, what data isn’t there, what might be missing, whose voice might not be being heard because of the nature of the data collection or something like this.
That to me is definitely part of what I would consider data literacy. And I would argue there is a parallel with understanding literature, and understanding the value of literature to be able to expose not just by what is there, but what isn’t there and what’s implicit or missing or needs to be read between the lines, the symbolism and so on.
[00:17:44] David: So I think there is a parallel there. And I think, as you say, the concept of literacy and data literacy is a very powerful concept. I wish I could spend more of my time on it, to be honest. It’s one of those things that what would the word look like if we had a high, you know, if we think about the transition from a population which is broadly illiterate to a population which is broadly literate?
And we now think about the fact broadly, our populations are data illiterate. We have a few exceptions who are really immersed in data in different ways, but as a population level we have very low levels of data literacy. What would our societies look like if we actually had really high levels of data literacy, if we took data literacy as seriously as we took literacy? Oh, I don’t know. But it’s a nice thought experiment.
[00:18:45] Lucie: It’s a good place to end too.
[00:18:48] David: And I should be absolutely clear that, of course, in that data literacy, I am including qualitative data as well as quantitative so that I don’t get into trouble again.
Thank you for this.
[00:19:01] Lucie: Thank you, David. It’s been interesting.