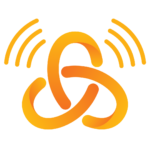
Description
Can AI-generated information be trusted? In this episode, Lily and David dive into the issue of AI-generated “hallucitations”, where generative AI models like ChatGPT provide ostensible citations referring to sources that do not exist. They discuss the implications of such misinformation, including defamation cases. They emphasize the importance of responsible AI systems and the challenges of funding and prioritizing research to ensure accuracy and reliability in AI outputs.
[00:00:00] Lily: Hello and welcome to the IDEMS podcast. I’m Lily Clements, a data scientist, and I’m here with David Stern, a founding director of IDEMS.
Hi David.
[00:00:14] David: Hi Lily. What are we discussing today?
[00:00:17] Lily: It’s a term dubbed Hallucitations.
[00:00:20] David: Yes. This is where let’s say an AI chat feature, such as chat GPT, is asked to provide citations and makes them up.
[00:00:31] Lily: Yes, and there’s been all sorts of problems out there and all sorts of scandals out there. One that I think it was around a couple of weeks ago, around May, that came out was of a law professor I think Harvard law professor, and his name came up from someone that was researching professors that have abused their students.
And it brought up his name and he said, I’ve never been to that school, I’ve never been on that trip. And he said initially he found it amusing, but then after a little while realised actually this is not funny.
[00:01:10] David: No.
[00:01:10] Lily: This is really quite, this is defamation.
[00:01:14] David: And it’s serious. This is the thing that actually recognising the limitations of what the AI systems can currently do and what they are doing is difficult. And people are using them now in ways where they haven’t really been trained in that way. I wouldn’t expect them to work that well in that way. It’s not that they couldn’t necessarily, but they might need to be designed differently to be able to do so.
[00:01:40] Lily: By this, do you mean the large language model, your generative AI, do you mean they’re not trained that way? Or do you mean the individuals using it?
[00:01:46] David: Oh, good question. I was referring to the live language models, but of course, there is an element about how you train people to use it effectively and responsibly. And I think that’s something which is going to be changing so fast as the capabilities of the models change.
I think one of the things to bear in mind is that the simple large language models, and that’s a rather silly thing to say because they’re not simple at all, but just using something like ChatGPT it’s trying to author something which you want to read, which looks like something you want to read, because that’s what it’s trained to do.
[00:02:20] Lily: Yes.
[00:02:20] David: To look like things you want to read, but it’s not understanding, there’s no depth of understanding on what it’s saying.
[00:02:29] Lily: Absolutely. I mean, this for me is where actually using stuff like ChatGPT or Gemini or whatnot, and seeing its imagery on it really shows to me that lack of depth.
[00:02:38] David: Yeah.
[00:02:39] Lily: Because, it’s so easy to see a bunch of text and to be blown away by it. But for me, it wasn’t until I started using it for images that I realized, okay, yeah, now you are not listening to me, or this looks so impressive, this image that you’ve made, this text that you’ve produced looks so impressive, but actually this doesn’t mean anything.
[00:02:58] David: Exactly. And this is the thing that actually to get that meaning, there are people who are working on solutions where that meaning is important. One of my favourite examples of this was people who were looking to build maths tutors using AI, where they found that if they tried to get the feedback directly using the AI it didn’t work very well. But if they tried to use the AI to get the results and then use the AI and the results to try and say, how should you communicate this results, they got much better results. They actually got better communication. So two layers. Now this is like actually, thinking before you speak.
[00:03:35] Lily: Yeah.
[00:03:36] David: A rather useful skill for a human, let alone an AI.
[00:03:39] Lily: And I, not on the same level, I’m not using it for maths tutoring or any tutoring. But for me, when I do use it, sometimes I do have to break down the problem, ’cause then it does give something a lot more coherent. I know many people that use it for getting information out there. And it is very convincing from how it looks. It lays things out so nicely, but it’s when you actually read those details that you don’t see that depth.
And if you even ask it for citations, which I know you can get citations on the paid version, I don’t know if you can on the free versions on chat GPT 3.5, but on GPT 4 you can, and actually it gives you convincing citations. It gives you an author name and paper, but if you go and look that up in Google Scholar or wherever, that paper does not exist.
[00:04:25] David: Sometimes it does.
[00:04:26] Lily: Sorry, sometimes it exists. I’ve been surprised as to how often it doesn’t.
[00:04:33] David: And I guess the key point is that it’s not impossible to design a system where it would always exist. But that’s not how these systems are being designed. This is the point. that there’s a whole lot of work which needs to be done to be able to ensure that.
So is that technically possible given where we are? Yes. Is that what the people developing it are prioritising? No. And this is part of what we need to worry about as a society. There are choices being made for which we as a society are bearing the consequences, where if we were to actually have objective choices, we might choose differently as a society.
And this is something where those power dynamics about who’s choosing what to prioritise in terms of a development, it’s such an interesting question. I don’t have any good answers to this. But I am pretty convinced that the sort of, equity model, where you’re asking for seven trillion dollars, which you’re then going to get a return on investment, that’s going to lead to a prioritization, which is about using AI to extract money from people who have it because they need to pay back that money, they’re getting an investment, they need to get a return on that investment.
And so therefore, elements of thinking about how do we want the AI development to really happen? Who do we want to be at the forefront of this? Where should that money be coming from? And how should it be coming? These are all really interesting and difficult questions.
[00:06:06] Lily: But out of interest though, we’ve got like these defamation cases, the one that I mentioned about the law professor, but there’s countless others, there’s a mayor in Australia who came up in an article that said in the 2000s I think it was that he went to jail for something and he never did. And, you know, knowing about these defamation cases, why is that not an incentive to then sort out the citation issue?
[00:06:28] David: It’s a matter of money. The point is, how big are those lawsuits going to be?
[00:06:32] Lily: Okay.
[00:06:33] David: They need to be enormous to make it a priority because it’s an expensive thing to do. If it’s in the tens of millions that they have to pay out for a few defamation cases. That’s probably not going to be enough. Start getting into the billions, and maybe it’s worth putting the investment in. You know, If those lawsuits were in the trillions, then suddenly, a trillion dollars would go a hell of a long way to actually making sure that these models are actually more responsible.
These are the sort of things where it’s insane to think that there should be that. But the idea of a class action lawsuit, which takes these false statements coming out and says it’s irresponsible to be doing this, maybe that’s the only way to actually get big tech to prioritise this. If the power balance is between big tech and, the law, then the law’s gonna have to get pretty big because big tech is getting huge amounts of money for this. And so they won’t prioritise it unless it hits them where it hurts, which is in the bank balance.
I’m not advocating for this.
[00:07:37] Lily: No.
[00:07:38] David: I want to be clear that I’m just trying to give a perspective of, it doesn’t make sense to prioritise it financially unless that cost of those defamation suits is enormous.
[00:07:50] Lily: Sure. So I guess I want to understand the systems a bit more. Maybe you don’t know the answer or maybe you have a theory as to why this is happening, but how does it make up citations? How is this happening? Because my understanding, or what I thought, was it would like trudge the internet, trudge all these cases, and so therefore it can flip through all of those cases that exist.
[00:08:08] David: You’ve made an important distinction. It is trudging the internet, it’s not trudging the cases. To trudge cases, and actually identify cases and just be limited to that data, that would be a different design. And that design, that’s a smaller dataset. Whereas actually, if you’re trudging a larger dataset, and you’re picking pieces from here and there and putting it together to give something which looks like something you want, where your reward system, incentive system, however your learning system is set up, is not about the individual sort of blocks.
What is a, what are you searching through? If you take the numbers of cases, then your data is automatically small.
[00:08:49] Lily: Sure.
[00:08:49] David: In comparison to if you’re actually just searching words across the internet or across the data banks you have. So all of this is about data and it’s actually a lot of it’s about multi level data. At what level do you want to be taking? Do you want to be taking a piece of a case here and a piece of case there and putting it together into something coherent? Sometimes that’s what we want AI to be doing, to be finding the best bits from all over and putting it together into a mismatch. You don’t want that in terms of a citation.
But how’s the model supposed to know when you do and you don’t want that? Because it’s designed to take this mishmash from here and there and everywhere and bring that together as data, these relatively small pieces, and put them together. That’s what it’s designed to do.
And so you’d need to build in layers where you’re actually treating data as multi level data, where you’re saying, okay, these cases can’t be broken down. You can’t take bits and pieces from one and another. So this is the sort of thing where that’s a hard data problem. The irony of course is, don’t get me wrong, what ChatGPT and these large language models are doing is amazing. But of course they’re doing the easy bit, in some sense.
The really hard bit of understanding the difference between fact and fiction, that’s almost impossible. That’s so much harder. How would they get that classification right? That distinction between what is true and what’s just being put out there. Those need to be done and they need to be regulated.
And understanding that we don’t have the structures yet. This is in its infancy. Do I believe we’ll get there? I think so. I think the technology actually isn’t that far off. But I don’t believe that the research is pushing in that direction enough of how to do this. I very rarely hear people in AI context worrying deeply about the multi level nature of their data and the difference between the confidence levels and different elements of their data, their data sources.
The complexities of the data sources, that in its own right is a huge area of study where, what are you feeding into the models and how do these relate? I don’t hear that in the sort of AI discussions as much as I should. There are a few people discussing these things, it’s not that they don’t exist, but they’re a minority. If we wanted to have really responsible AI being developed, then the funding needs to shift so that the funding is primarily on building methods that can be responsible, not just building methods which are bigger and faster and able to appear to be effective.
Effectiveness is not the limiting factor right now. Our models are pretty effective, but they’re not that responsible and that’s not the fault at this point, I would argue, of the models. But I would argue, as a society, we need to make choices about where we want these tools to develop. And if we want them to develop in ways which are responsible or to society’s benefit, we probably need to think, are the funding structures we’re putting in place encouraging that or not? And at the moment, I’m afraid I don’t think they are.
[00:12:01] Lily: Interesting. That’s very interesting, but obviously incredibly worrying. I mean, I just hope that having these stories that come out, these articles that come out of the law professor, of the Australian mayor of this, that and the other, help give awareness. And maybe if, people are using this at the university level or in education and they are using, fake citations that hopefully their teacher or lecturer will pick up on it and then from there they will learn, okay, it’s making things up.
[00:12:31] David: But think about how important this is in so many different ways. And the people you’re citing are people who are already in positions of power. What about people who aren’t in positions of power, who get caught up in this, but have no power to actually demonstrate this is false? This is the sort of thing, the consequences on our society, we have no idea right now.
I’m not trying to make it even worse or even scarier. But it is something where at the moment, we’re playing catch up to try and be responsible. As you say, these instances are coming out which are highlighting of course, this is ridiculous and this has got out of hand. This was going to happen. There’s a question of should we have been more responsible before it happened? Or should we be responsive to when it happens? And if we’re only being responsive to when it happens, what about the times when it’s happening and nobody sees the consequences because somebody is not deemed important enough to actually make a fuss.
This is where I come back to these sorts of, if you go back in history, particularly in the US, these class action lawsuits, this is what may be needed, for people who aren’t a Harvard professor, a regular person in the street getting caught up in something like this. I suppose in some sense, I don’t quite know how that’s going to be, how it’s going to turn out. Because it is something where the negative implications for society, for a regular person, I’m more scared of that than I am killer robots, which is what they ended up talking about, at the safety summit.
But the actual harm to people’s lives due to misinformation, this is so much more important. Without AI, pre AI, there were already big misinformation issues, and it took years for the lawsuits to actually have an impact. I just read recently that the Sandy Hook shooting and the sort of misinformation around that, finally, after many years, the law seems to have caught up. The consequences are being felt, but it took a long time. And it’s going to get worse with the AI sort of superpower in this. And will you be able to hold someone accountable? If so, who? I don’t know. This is serious stuff.
[00:14:41] Lily: Yes. And I suppose maybe with the more responsible AI, like ethical AI laws and whatnot coming through. I don’t mean laws. I’m trying to think of the word that I mean.
[00:14:52] David: Regulation.
[00:14:52] Lily: Regulation. Thank you. Presumably that’s something that the regulation will touch?
[00:14:56] David: Yes and no. The regulatory frameworks are, especially the European one, but also in China and the US and elsewhere, they are looking at these issues. And it is possible that they will put in place regulation, which will mean that the law will be stronger. But it’s really not clear and I also feel for the, developer side, if your business model is at stake here, you can’t afford to try and be responsible because you’re under so much pressure.
We need to have less equity funding going into AI and more research funding. That balance between those two, having research funding pushing the boundaries is much, much safer. Having equity funding where you have to make a profit pretty quickly to get back. That’s a bad combination in my mind. It’s a dangerous societal combination. It’s the reality of the world we live in right now. But it is a dangerous one.
I wish there were more people actually putting research funding in, or funding where it’s not about maximizing profit. I don’t think there’s a problem with aiming to make a profit of course, we have to do that as well. But it’s the maximization of profit combined with this very young technology where there is significant risk of harm. There’s real danger here. This is a combination which I think is worrying, to me anyway.
[00:16:24] Lily: But with every worry comes a, or with every challenge comes an opportunity. So what’s our opportunity for this one?
[00:16:31] David: You’re just trying to get me to finish on a positive.
[00:16:33] Lily: Yeah.
[00:16:33] David: Which I think normally I’d be really happy with you for trying to do this. But I think, let’s come back to actually what this topic was about, which is about these citations. I believe fundamentally, if that is the problem with the current technologies as they are, it is a solvable problem.
I don’t believe we need huge new advances in mathematics or in data science to be able to get systems which can ethically and responsibly use AI systems to provide citations reliably. I just think we need to design those systems differently and I think the research needs to go into that. This is a topic I’d love to be able to put time into. It’s a hard topic. I don’t think it’s an easy one. Maybe the upcoming versions will have cracked that nut because it’s an obvious one where there are issues around this which is very visible. And it is one which is solvable.
And I come back to the fact that I think that one of the ways to solve this is to make it a two layer problem. That when you’re actually dealing with citations, you’re actually using a different model to extract the citations. It’s a separate process. So you’re separating out the two processes. You’re doing a multi level process. Most data we work with in the world is multi level data. It’s absolutely obvious to me that if we want to do responsible AI, we need to be having multi level models, which are much more common than they currently are. It’s not just about them being multi level, but it’s you know, this idea of actually within a response, it containing components which are coming from different sources and some of those sources being constrained and some of them being less constrained.
That approach to building up the models is, and I’m not suggesting something new, I’m not saying that the current models aren’t bringing elements of this in. What I am suggesting is that If we’re wanting to have these responsible systems, almost certainly that’s going to be part of it. And that’s going to be a really important component. And I haven’t heard enough academic discussion on this, that a lot of it is actually on the algorithm side, more than on these sorts of data, the data side and the source of the data and the complexities in the data.
Because the point is that actually that’s using existing technology. And for many researchers, particularly in the mathematical sciences, you’re not pushing the boundaries. So I’d love there to be this research which focuses on using the current technologies to be responsible, that sort of thing. And actually not just research, this is something which is coming in to some of these models, and I believe it will.
There’s a question of when, and there’s a question which I’m really worried about incentives for it to come in. And I really hope that there are better incentives than lawsuits, but I don’t know if there will be.
[00:19:22] Lily: Very interesting. And just to pick up there, because you’ve mentioned a few times about this research, and the research about how this will help it, but what do you see the research doing? What are we researching?
[00:19:32] David: Well, there’s a lot of people doing research into ethical AI in all sorts of different dimensions, in all sorts of different ways. I’m not going to say they’re missing the mark at all. We’ve worked with philosophers who are doing work which we feel is outstanding.
What I do feel is that if you look at the amount of money which is going in to the research, if you want, into building new systems, and you compare that to the amount of money which is going in to research into how to build responsible systems.
[00:20:07] Lily: I see.
[00:20:07] David: My claim is that balance is rotten, that we need to get a higher proportion of the effort of people who are at their intellectual capacity, putting in the effort to try and understand the responsible element for societal benefit. And that’s a hard thing to do because that does not necessarily bring a return on investment in the same way. Now doing so responsibly, maybe it can bring a return. It can’t bring an exponential return in the same way that people are wanting from equity funding.
I’m not against investment as an approach to get this because that is where you get orders of magnitude of money which are bigger. What I’m worried about is that combination of equity investment looking to maximize profit with the fact that almost inherently excludes the responsibility angle. It’s that money is not going into the responsibility and therefore it’s disproportionately moving technologies ahead without balancing it out with people who are thinking deeply, who are engaged intellectually with trying to ensure that responsibility.
Now I want to be absolutely clear here. Many corporates are interested and engaged in the responsibility, the ethical AI space. So this is a big space and I’m not trying to diminish those efforts at all. I’m just trying to say that in my observation, there is an imbalance. From a mathematics standpoint, the underlying research that’s going on.
What’s ironic, of course, is that those researchers are not researching the problems that really relate to the necessities about responsibility. Partly because it’s not breaking new ground. It’s often using the tools that are already available. And from a, if you want, big tech perspective, of course, you’ve got to get the new models out there because it’s equity funding and all the rest of it, and so therefore, that’s not prioritizing it as well.
So although there are a lot of people who are concerned about this, who are putting time and effort into it, they somehow are falling into the middle, where you’re not necessarily getting everybody agreeing that this is the right way to pursue. And I don’t know how you could, but I do believe that there could be a more conscious understanding of how to get this responsible approaches into the research, which is leading to the development, which is happening both within big tech, but also in academia.
[00:22:45] Lily: Great, that’s very interesting. Do you have any final bits you want to say before we round off?
[00:22:50] David: The final bits is, I really look forward to the day when the citations you get from an AI system you can rely on. I think that this is possible. I think it will happen. When it will happen, I don’t know.
But this is the sort of thing where there will be a time when you can, and just think about the power of that in so many different ways to help us in our work, in our research, in all areas of actually being able to trust. Now of course there is an element, where just because you’ve got a citation onto something, that doesn’t mean that it is correct.
And so I’m looking forward to the day when we can go a little bit further. When the AI can also help to be able to give levels of uncertainty or levels of confidence in different sort of things. To be able to give a more critical perspective than we can do by just saying, oh, somebody said it was true, therefore here’s a citation.
[00:23:46] Lily: That’s fantastic. Yeah.
[00:23:48] David: And I believe that’s possible with AI, but we’re a long, long way from getting there. If only we were using AI to enhance our ability to get truthful information, rather than at the moment to, as a dangerous tool, which is creating misinformation. Wow, that would be so powerful. And I believe technologically, with the tools we have today, it is possible to design such tools if we choose to do so. It would be expensive. And that’s the thing where it would have to be the likes of ChatGPT who decides this is a priority. We’re going to stick a stake in the ground and say we are on the side of truth and information and not misinformation. And I don’t believe they will do that.
[00:24:35] Lily: And to put a, I guess, positive on the current system, which is that at the moment, these citations are often made up or whatever, or they might be misinterpreted by AI at the moment. Maybe for now is a good thing, because it means if you are aware that the citations being made up, you’re then going to read that article to check that it does support what you’re trying to say.
[00:24:54] David: So, I don’t think that necessarily, I wouldn’t qualify it as a good thing, but I do hear what you’re saying, that if you as a user today are using this and recognize that the citations may be made up or may be misrepresented, and they may be representing something truthful or not. If you recognize that, then maybe it’ll help you to be critical in the way you use it, and that is nothing but a good thing. Humans’ roles in this should be the decision makers, you should be the critique of whatever is coming out, and that role of having humans in the loop playing that critical role, playing that thought processing role, being able to take responsibility for what’s there, that’s exactly what we need. And this is part of what may come in some of these regulations, how humans are in the loop and the roles they play. But we shall see. The future is unknown.
[00:25:49] Lily: Perfect. Well, Thank you very much, David.
[00:25:51] David: Thank you.