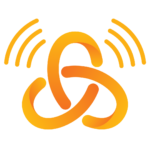
Description
Open access software tools like ODK (Open Data Kit) have been a game changer in enabling access to digital data collection. Lucie and David discuss what makes ODK so interesting, and compare its development and use to that of R-Studio, another open access software that has made waves in data analysis. What will be the next step change in data collection? Does ODK’s impact represent an alternative model to that of “big bets” as the route to bring about large-scale change?
[00:00:00] Lucie: Hi and welcome to the IDEMS podcast. My name is Lucie Hazelgrove Planel, I’m a social impact scientist and anthropologist at IDEMS, and I’m here today with David Stern, one of the founding directors of IDEMS.
Hi David.
[00:00:21] David: Hi Lucie, what’s the topic for today?
[00:00:23] Lucie: Today I’d be really interested to talk about data collection.
[00:00:28] David: Yes.
[00:00:29] Lucie: Because in the last 10 years, possibilities for data collection have changed completely. And I’m not talking about computers, the arrival of computers. I’m talking more about the widespread use and availability of smartphones, I think, or tablets as well, actually.
[00:00:43] David: I think you might even need to go back further than 10 years for that, but yes, there has been a real shift. And I think there is another shift which is emerging. So, yes, this is rather interesting.
[00:00:56] Lucie: I’m not sure about that other shift, I look forward to hearing about that. So in the past, I think historically, people have always done a lot of data collection by hand. So filling things out on paper, especially like questionnaires. So a lot of the researchers and research projects that we engage with, that’s what they started off doing, I think I’m correct in saying, but now a lot of them are realising that they can be a lot faster if they do it directly with online forms, well, digital forms, they don’t need to be connected to the internet at the immediate time.
And so some of the advantages are that you don’t need to input the data afterwards into a computer to then analyse it, because obviously everyone uses computers nowadays. And then you don’t need to lose forms, or you lower the risk of losing the paper forms, or them getting damaged.
[00:01:47] David: And in some sense, for many listeners, they might say, well, wait a second, this did, as you say, happen 10 years ago, or more. And that is in, if you want, high resource environments, this shift happened in the past. And actually some of the shift went directly to phone, it went to automated things and other ways.
And there’s been a whole set of things which happened in high resource environment, but most of them were paid services. And so in the low resource environments we work, it has been slower to happen. And some of the transformative technologies are the open technologies, where suddenly there isn’t a pay wall between you and doing this.
So in some sense, this is why this sort of process, actually has a very long history. You know, national stats offices, this history with them is rather interesting and very different again, and they’ve actually got tools which are more specific to them, which aren’t used so widely outside.
A lot of the researchers we work with, use things like Open Data Kit, ODK, or some derivative. Kobo Toolbox is one of the most popular because Harvard was able to get a grant which meant that researchers worldwide can have the hosting of this freely done in a very efficient way and so on.
But it’s all built on these open data kit technologies. And that’s been, I think, as you say, I’ve observed that over, I guess, a 15 year period when I’ve been working in low resource environments, this has been coming in. And 10 years ago I was training people on ODK, roughly 10 years ago now, when this was something which was really unusual.
And now what’s really nice to see is that actually a lot of people are just exposed to it. That there is a lot of it around and it’s being used by all sorts of different people. It is almost the norm.
[00:03:48] Lucie: So a lot of the interns that we currently have in Mali, research method support interns, a lot of them, or perhaps about half of them, already have, well, either experience or exposure to ODK, which is really interesting.
[00:04:00] David: Absolutely. And this is demonstrating what a powerful set of technologies can do in these environments. And what I think is so interesting is that, as I say, in high resource environments, 10 years ago when I was involved, this was already known and already done, and there were so many tools and there’s so much going on, and people have been doing this for years and years.
But, when you went to the low resource environments, almost none of them applied because they weren’t designed for it. And what ODK did, and what was such a game changer, it wasn’t just that it was open, it was actually somebody who wasn’t part of the core ODK team who took the next step, and this is one of our big learnings.
So a lot of our technologies are built from the insights we gained of watching what happened with ODK, where there was this Excel authoring process for ODK. Now, what this did, was two things. One, it met people in a technology where they work, you know, most people were using a spreadsheet.
[00:05:17] Lucie: Exactly. Everyone loves to hate Excel.
[00:05:20] David: Exactly. You know, people are just familiar in that. And that meant that it was something where you were meeting people in a tool that they were familiar and used to using, rather than them having to learn how to use a new tool. Even if it’s easier to use that new tool than the tool they’re familiar with, there is still a barrier for some people.
And the second thing, which was so incredibly powerful about this, and this is maybe my deepest insight, was that if you had a website with a nice visual front end, people couldn’t collaborate on it in the same way. Whereas collaborating on a spreadsheet, people were used to doing that. They were used to sharing it, even if they were offline, they could work on it and they would then share it with others. And then people could do different columns because sometimes you have things like translation. And so they would do their column, but they’d be working and sharing and collaborating on the spreadsheet, which they are used to doing.
Whereas actually any new system which was built, either it was really built from the perspective of one person working on it or when it’s actually quite complex and it really is quite complex to manage collaboration well on a web tool with a nice visual user interface. So then there was a high barrier to learning it.
[00:06:54] Lucie: And we’re seeing that a bit with some of the partners that we work with, I’m not sure whether they’re partners in terms of the strict sense of the term, but who have been trying to develop things individually, and it has caused problems later down the line because then it’s harder to collaborate with others, exactly as you were saying.
[00:07:11] David: Exactly, yes, it’s exactly that element, and I don’t know what a strict sense of the word partners is, we work with so many different collaborators and I do consider them all partners in different ways.
[00:07:20] Lucie: Okay.
[00:07:21] David: But yes, in many cases, this element of people building technology or using technology and doing things themselves, which work for them, then the barrier to then getting collaboration on that can be huge.
[00:07:35] Lucie: And then that sort of lowers the sustainability of the technology as well.
[00:07:39] David: Exactly. And this is what, in a high resource environment, this sort of doesn’t matter because people just pay for things. You then buy the commercial versions and so on. But in low resource environments, these efficiencies are so important, and actually building towards them.
I mean, the digital data collection piece on its own is also really important. But in some sense, this is old news that we are talking about. It’s important old news, but it’s been bubbling along for many years.
[00:08:07] Lucie: Okay, am I correct though in, like you say, making this distinction between high resource and low resource environments, that it is still relatively recent in West Africa?
[00:08:16] David: Well, in a lot of the lower resource environments, not just West Africa, it has only been really coming in over the last 10 years, whereas in high resource environments it dates back a lot further than that. And it really is, from what I’ve observed, the single importance of ODK and its derivatives. Because many people don’t even know they’re using ODK because they use things like Kobo Toolbox, and so they say, no, I’m not using ODK, I use Kobo. Yes, that means you’re using ODK behind the scenes.
And this is what’s so fantastic about open source. It’s not a brand, it’s a technology which has been developed and which is used and which is then further developed by others to become more powerful. I should at least mention Honor in this because they are another organization and my understanding is it is the founders of Honor who actually developed the XLS form format.
[00:09:10] Lucie: Interesting.
[00:09:10] David: And then they sort of developed a company which then has now their own use of this in a different way, and they’ve taken it in a different direction. All building from these open source principles and open source core, which was ODK. And ODK, I believe, it is a real centrepiece in the transformation that I’ve been observing and learning from. And I think this is what’s so important.
And what’s really interesting here is that there’s some other thinking about this in different ways, which is highlighted by a book which has recently come out by some excellent philanthropists doing fantastic works. And they talk about big bets.
[00:09:48] Lucie: Okay.
[00:09:48] David: And how real change happens through big bets. I don’t think ODK was ever a big bet and yet it is one of the most meaningful changes I’ve seen, gradually impacting so many areas of work and so, so much substance behind it. You know, it’s like R. R was never a big bet, but the influence of R and the way it’s grown, and you take these two together and you now have actually elements to collect data and elements to analyse data.
[00:10:19] Lucie: Can I just ask about the comparisons then between R and ODK? They’re both open source then?
[00:10:24] David: Yep, they’re both open
[00:10:25] Lucie: And the open source bit is this second movement or something that you were talking about at the beginning, is it?
[00:10:30] David: No, the open source, that’s the first part in some sense. So there was a whole movement before that, and there are other things which are sort of, it’s not worth naming lots of things, so I won’t keep going on the names, but I will say that ODK in terms of this digital data collection, I think that is what led to what I’ve seen as this revolution over the last 10 years in so many ways, in so much impact. The way it’s changed what people can do has been fantastic.
I believe that there is a need and an emerging element which is coming out. And I suppose this relates to AI in certain ways and what’s happening in the artificial intelligence sphere. But ODK is, ODK is primarily related to statistics, in terms of data. Why? It is about designing. You do a good design and part of that design involves designing the data you collect and then you analyse. And the analyse part of that, that’s what R, which is, if you want, the biggest open source statistics project…
That’s why I brought those two together almost as a pairing. Because really from a statistics standpoint, I would argue that ODK and R in terms of open source, they have shown the power of open source.
And I’m again, going to put this in contrast to these big bets, which is a fantastic philosophy about how change happens in the world, and it talks about things like how people have managed to eradicate polio and this sort of thing. And my claim is that the thing that they’re missing is that a lot of those big bets actually rely on these underlying advances.
And what I’ve seen is how these underlying advances actually have enabled some of those big bets to happen. And so I think that what I’ve observed on the ground, working in many places, is that it’s the slow progress of some of these underlying technologies, which have actually enabled a lot of the other things to suddenly work and become cost efficient and cost effective.
If you just try to do the big bets without doing the slow hard work, then suddenly your progress sort of stalls. And in my mind, this underlying infrastructure, and it’s a digital infrastructure, but R and ODK, I would argue, have been part of what’s, you know, scaled and enabled things that would not have been possible without them.
And that’s part of where, coming back to ODK, it wasn’t the real innovator. And I think this is important. I’m not trying to take away from ODK, I’m a huge fan.
[00:13:18] Lucie: We’ve seen.
[00:13:19] David: Yes. But there was a whole history before them of people who had done digital data collection in different ways. And broadly what ODK did was it learned from what others were doing and it basically said, we’re not after this as a commercial venture, we’re after this as an academic venture. We want to get the best learning out of how to do this well and we want to make it openly available.
[00:13:48] Lucie: Okay.
[00:13:48] David: And that’s what they did. I was an academic. I love academics. I work with academics all the time. But most good academics, they are learned rather than innovators. And there’s a distinction between those two. And being learned means they knew what everybody was doing. They understood everything and they put it together and they were able to say, well, from what everybody else has done, this is what’s known, this is where our knowledge is. And maybe they push the boundaries of that knowledge to put it together in a way that hadn’t been done before.
But many innovators that I know, they innovate almost in a vacuum. And that might mean that they do something which is totally out of the box new, or it might mean that they’re reinventing something that somebody else already knew.
[00:14:39] Lucie: Yeah, that’s really interesting.
[00:14:41] David: That’s the innovation process. Your academics are very rarely brave enough to be totally out of the box thinkers, because they know what they know, and they know what they don’t know.
[00:14:53] Lucie: Exactly. And the whole point is to work, to build on the knowledge base.
[00:14:56] David: Exactly. This is not a criticism of academics or of innovators. This is just an element of trying to understand the different roles they play. And I would argue that ODK, as R these are academic pursuits, in the best possible sense of that world. And that they are building from the best current knowledge. And I really wish society valued that more.
I think there is an issue where actually a society at the moment tends to love your rogue innovator who doesn’t really know what they’re doing, but he’s able to talk a good game. I’m not going to name any names on this, but people might infer who their favourite person is who talks a good game.
[00:15:35] Lucie: I think I can guess…
[00:15:38] David: But it’s not about criticising innovators, society needs innovators as well. But I do feel that the balance of power and money given to academics and innovators, I would much prefer a society where that was a little more balanced than it currently is. And I think part of what we’d see there is initiatives like ODK, which provide the groundwork for really meaningful change at scale.
I’m not saying Big Bets don’t, that’s the innovator story in some sense. But I feel that’s really important.
[00:16:12] Lucie: Well, it’s more exciting, isn’t it? I think people catch on to sort of a big idea.
[00:16:18] David: Big Bets. This is exciting. This is fun. We can have a lot of fun and try something new and be very clever in the process. The really clever people, the real academics, they know that it’s about actually building the framework slowly and steadily. And not instead of innovation, they also value innovators and they try to learn from them and they try to do that.
And so to me, these are both needed. And if you want your highest intellects, by and large, they tend to be the slow and steady. And so, recognising that, and you’ve got to recognise that here I’m also putting myself down because many people would not consider me slow and steady. That demonstrates how much value I give to people who actually have the patience to do that. And my patience is sometimes limited on this. I want to get things done. And that does mean that my, if you want, my intellect probably suffers from that.
[00:17:12] Lucie: But this is where things being open source and community built is interesting.
[00:17:17] David: There’s a really important distinction in what you’ve said there. Open source is not the same as community built.
[00:17:24] Lucie: Yes, no, I agree.
[00:17:26] David: In fact, to be very precise, ODK and R are not really community built. I can explain this much better with R than I can with ODK because ODK’s different. But R is very simple on this. R made the explicit decision, the core team of R is very small and it’s very exclusive. And they then built a system where contributors could contribute, but not to the core.
[00:17:57] Lucie: Okay.
[00:17:57] David: And they could contribute in a way that would be recognized to be part of an outer circle. In the R world, this is CRAN, and broadly that’s just a way of approving certain packages built by other people as satisfying an agreed upon community set of standards with saying nothing about what actually they do. And so this is sort of this two levels. So they have the core, which is not community built, and then they have the CRAN universe, if you want, which is community built, and within that there are teams who are driving forward their own agendas, and the key example of that is now called Posit, but was R-Studio. And they built the Tidyverse.
[00:18:37] Lucie: Okay.
[00:18:38] David: Which is a whole universe built on R, which is what most people, I’m afraid, myself included, it’s what we would tend to use within R, we would go beyond R core. And there’s good reasons for that, it’s not a criticism of R core, what our core is doing is incredible, but that’s an exclusive group and they’re very good at what they do. Whereas, the extended community is a layer outside.
Now, there’s questions in terms of business models and all the rest of it as well. How do you sustain that? Who should sustain that? The community that should sustain the core. But does the community value the core? And so on. There’s all sorts of complications there. But your really insightful statement was this sort of…
[00:19:23] Lucie: Whether it’s community built. Because I guess I, with ODK I’m aware of the community where people can sort of support each other in using ODK and make suggestions as to what is missing, but absolutely it’s just making suggestions as to what they would like as opposed to actually being able to build it and integrate it within the system.
[00:19:40] David: Well they can because it’s open source, so if you have the skills to do so.
[00:19:44] Lucie: Integrate it within the original systems for everyone else to use.
[00:19:47] David: You can because it’s an open source system and unlike R, actually the barriers to contributing to ODK are lower. But it is still a relatively small group, as I understand it, who are the core developers of ODK itself. And what’s interesting is that it really has a community of users, much more than a community of developers. And there’s a really fundamental difference in the models between ODK and R in this in the sense that ODK, the community of users are not really overlapping with the community of developers because it’s totally different skill sets.
If you’re a computer scientist, you’re unlikely to be going out in the field and collecting data.
[00:20:35] Lucie: Exactly.
[00:20:36] David: Whereas, R, the core, is really good statisticians. And really good statisticians do analyse data themselves.
[00:20:44] Lucie: But I’ve got to say, I just saw, I think as part of the ODK forum, sort of, newsletter or something last week, one of the learning points for them was that perhaps some of the developers should go out to the field more and see how ODK is used.
[00:20:57] David: Of course I approve that, but the important part is the other way on as well. Your random user of ODK is unlikely to become a developer.
[00:21:09] Lucie: Yes.
[00:21:10] David: And this is the thing, whereas a user of R actually could say, well, wait a second, I need to do this. Well, I could write the code to do that. I make it into a package. I now get that experience building packages in R and I want to go deeper. And then they could evolve into the core team and be contributing in that way because it’s a continuum of skills.
[00:21:31] Lucie: Why is it so different than with ODK? I mean, is there code behind ODK that you need to know in order to develop it?
[00:21:38] David: Yes, because ODK is deliberately, basically code free, and so there’s no continuum here. ODK uses divide design forms, whereas ODK developers write code.
[00:21:49] Lucie: Yeah. Okay.
[00:21:50] David: There’s totally different, whereas with R, you could have a core that is just software developers, but that’s not what it is. They’re statisticians who write code.
[00:21:59] Lucie: And so I’m definitely one of those ODK users who don’t understand and hasn’t seen the code behind it all, which makes it all sort of connect the Excel workbooks or whatever kind of workbook with the actual thing that you see afterwards, the online form.
[00:22:15] David: And I think the point there is, and of course it’s not just ODK, there’s so many other things related to digital data collection in all sorts of ways, I don’t want to try and list them, but what we are seeing and what I hope comes out of this is this revolution that I’ve observed on the ground happening, I believe, attributed primarily to ODK about digital data collection in low resource environments. I feel that, that process is actually one of these real changes.
I mentioned the second change, the one that I think is emerging.
[00:22:52] Lucie: Yeah.
[00:22:54] David: And for us, the example to understand this that we have is our collaboration with FUMA Gaskiya.
[00:23:02] Lucie: The farmer federation in Niger.
[00:23:05] David: Exactly, the farmer federation in Niger, who has built an app. They found for various different reasons, they didn’t want data surveys at individual points in time. It wasn’t just that they wanted longitudinal studies, which I’m really happy to say, things like ODK are now able to do much better and there’s sort of real advances in that direction. They wanted something which was serving the farmers, and also was collecting data.
Now, in many ways, again, if you go to the high resource environment to develop word, to Big Tech, they’ve been doing this for years. This is exactly what they do. They harvest data out of what you’re using all the time.
[00:23:50] Lucie: With a slight perhaps change in the fact of who is the data servicing?
[00:23:56] David: Exactly. Exactly. And that’s the key point and that’s what I observe could be happening now and into the future. There is this growing awareness of the value of that data and the fact that actually that data can and could serve communities.
[00:24:14] Lucie: And should.
[00:24:16] David: I absolutely agree with you. I wasn’t brave enough to go that far. But yes, I believe it should. I believe that data should be serving communities. It should not be extracted out for profit. But it is extremely profitable at the moment and therefore actually that view that it should be serving communities instead of enriching tech companies is a slightly controversial one.
And it is a question of, you know, well, how could that work, and so on. There’s a philosophical question there, which I’m happy to sort of skip over. And I would have skipped over more if you hadn’t brought it up.
[00:24:51] Lucie: To me it’s not even a philosophical question, it’s just black and white, that even.
[00:24:56] David: I think it’s philosophy, isn’t it? Anyway, no, let’s skip over it. We’ll not get into it.
[00:25:04] Lucie: So it is one of the aspects though, which is really exciting about our work in, well, especially in West Africa, but it’s a lot of what IDEMS does is trying to change these, or exploring if it’s possible to change these dynamics.
[00:25:17] David: Exactly. It’s exploring if community based tech could be using data and making data available at scale in different ways to the communities it’s serving.
[00:25:27] Lucie: And again, it’s not just community based tech. It’s accessible, accessibly developed community based tech. So like we’ve just been talking about…
[00:25:36] David: I’ve never heard that term. Accessibly developed? No. What, how did you have that?
[00:25:40] Lucie: I think that’s what I said. But it’s not only that it’s developed accessibly.
[00:25:43] David: Accessibly developed. No, I mean, that is really interesting because that resonates with me, and I don’t think I’ve ever heard it or said it, but it does. It’s this fact that the barriers to becoming part of the development have been lowered and therefore it is more accessible to become part of the development processes. That is the centre of a lot of what we’re trying to do. I mean, it’s hard. We certainly are not doing it fully as I would like at this point in time, and we won’t for a number of years. But it’s definitely the direction that we’re trying to explore.
[00:26:18] Lucie: Yeah.
[00:26:19] David: And I think the point there is we’re not the only people trying to explore these particular things and these ideas of getting data which is not designed. And this is the thing, it’s been a bit of a windy road, but at some point I was saying that, you know, ODK and R, this is sort of broadly statistics.
It is designing the data collection process, and then doing the analysis of that designed data. So you have the whole research process, or it could even be a census or whatever it is, but it’s all designed and it’s happening in that way.
And that’s broadly the statistical processes. Of course, what’s so exciting with data science, is really that data science and machine learning and AI is built out of using data that wasn’t collected for that purpose. It is data which exists. The big generative AI models, they are built on the internet. The internet was not designed to collect data to serve AI. It just so happens that the data that is available on the internet can serve AI. And this is, if you want, to me, a valid distinction between statistics and data science in the way people are currently looking at it. Where data science is accepting that it’s using data where there wasn’t a designed process in the same way.
There are other people who have other ways of describing this. I’m not saying that that is a black and white, nobody should be quoting me as this is the difference, but that is one way to interpret the differences. And it’s useful in this sense because I would argue that just like ODK transformed data collection to be able to scale out elements where statistical approaches could be used more effectively…
I think that there is an opportunity, and what we’re really trying to work in the space of, is that place of actually saying well where could the data to be able to ethically do these AIs really responsibly through having sensible data sources with data streaming in, in interesting ways…What is that innovation? What’s that open innovation?
Which could mean that we maybe remove some of the biases. If you look at the internet, well, actually, whose voice are you hearing? There are certain places which are more active online in terms of the data you can get than others, and therefore those are the voices you’ll hear.
Whereas actually if we had other mechanisms, you could actually have algorithms designed to be using data which was more appropriate for the context they were serving. And this is pie in the sky thinking, in some sense, at this point in time. But it’s actually happening, you know, the groundwork is being laid.
ODK, 10 years ago, already had a lot of groundwork behind it. 10 years ago, it was at that place when I was teaching using it, trying to get people to use it and take it up. I still remember one professor from Maradi in Niger, suddenly, you know, he was so engaged, his postdocs, his PhDs, he has a whole empire, if you want, of people who are normally doing the sort of detailed work for him, and he sits back.
[00:29:40] Lucie: This is Professor Baoua, I think.
[00:29:42] David: This is Professor Baoua. But for ODK, he was so engaged, because he saw immediately the first time it was introduced to him, he saw this is going to change everything. And it did, he came back the following years, and he was able to say how he was now able to follow his students, and the student who was under the tree filling in questionnaires instead of actually doing the proper work, he saw that the same night and gave him a talking to.
I’ve said him that because almost certainly those bad students were almost all male. Of course, that’s why I was using him.
[00:30:17] Lucie: I’m fine with that, I’m fine with that. [Laughs].
[00:30:26] David: But the reality there, watching that happen, and watching again how someone who is at the top of their field was able to identify, this is a game changer for me, and be able to seize it and put the time in himself personally, in a way for other things, he wouldn’t because this wasn’t worth his time. He was such a great judge of that. But this was important to him. And this was almost 10 years ago now.
[00:30:56] Lucie: And we’re still actually supporting his project in using ODK and in exploring their data and things like that.
[00:31:03] David: Well, what’s of course interesting is his project is across three countries.
[00:31:08] Lucie: Yeah.
[00:31:09] David: And we are not supporting the Niger team to use ODK at all. But his influence, and this is where you also see his influence, is incredible. It is across the three countries. But the ODK skills influence has diminished by the time you get to Mali. And that’s where we are still supporting the Mali team.
[00:31:26] Lucie: Exactly
[00:31:26] David: To be able to sort of see through what he started 10 years ago and recognize and build from this. Because it’s hard, it is really hard to get these things working at the scale people like him are working, the quality of that, of their work is incredible. And I would argue that a lot of the work they’re working on is sort of things like, I come back to these big bets. They’re sort of doing, in some sense, some elements which would be akin to that.
[00:31:53] Lucie: That’s a really interesting view.
[00:31:56] David: It’s that acknowledgement that if you want to make that big change, you’ve got to get the details right. That’s why I love Baoua. And some of those details, the one that I particularly get is ODK. He just grabbed.
[00:32:11] Lucie: Are there any closing comments you would like to make, David, about that? To me, that’s a really nice place to end, the practicalities of it and, an example of someone who’s made the most of it, I guess, and he’s been able to develop their empire. And I call it an empire.
[00:32:24] David: I should apologize to Baoua. If he’s listening, you know, we mean it in the best possible sense.
[00:32:29] Lucie: Absolutely. There’s huge respect.
[00:32:32] David: Respect for how he has built these really powerful structures, which have had so much impact.
I guess my last comment really is to come back to, I think, the starting point. And this is this element that I suppose you came in really with this observation that helping people to take up this digital data collection.
[00:32:56] Lucie: We should mention that some of the projects we are still supporting have up until recently been still using paper.
[00:33:02] David: And with relatively good reason. It’s not always bad reason, but it sort of can be with good reason. And what I would like to clarify here is that there is always a cost to pay for everything. Moving to digital data collection is not a silver bullet solution.
[00:33:17] Lucie: Yep.
[00:33:17] David: You still need to design good data collection forms and those data collection forms change in what you can collect and what you can’t collect and how you collect it. What can you do on paper versus what can you do using digital data collection. And you can’t easily scribble in the margins.
[00:33:32] Lucie: No, exactly, I was just thinking that. It’s less enticing, I guess, there’s a small box of where you’re meant to type all of the text of the answer or something.
[00:33:41] David: And this is where good digital data collection is really, really hard. And we’ve seen many people actually go backwards by moving to digital data collection, where they underestimate it, they expect it to now solve all their problems, but it doesn’t. Because most of the problems are scientific in nature, they’re really about the nature of this.
We’ve just had this with a sort of recent study which we were doing with Oxford, which is on actually the other level, it’s on the data collected not from ODK, but within a systemic data collection, where the problem was the interpretation of a variable. And we actually ended up going backwards and forwards quite a lot because the variable they were analysing and using in their model, when we read the interpretation in the paper, we said, hmm, that doesn’t quite match with what that particular variable corresponds to and measures.
And of course, because you’re getting so much data, which is just coming back systemically from inside an app or something like this, then there were lots of different possible variables they could use, the analysis becomes much harder and much more complicated. Now which variables should they be looking at and why, and what are they learning from the different variables? That becomes a whole different set of problems, once you get to these other types of data.
And so, open data; it is not that by suddenly having an advance in the type of data you’re getting or the tools you’re using, that suddenly this resolves all problems. It is the fact that it advances you in one direction and you now need a whole other set of skills to take advantage of that. It’s complex. We do live in complexity.
[00:35:21] Lucie: Which is what makes it interesting.
[00:35:23] David: Absolutely, and what makes me slightly nervous about some of the ideas around the Big Bets philosophy. Because, actually, the Big Bets philosophy is to say, well, okay, if we really put our efforts into doing this, we can do this.
[00:35:39] Lucie: Is this your sort of silver bullet solution? Um, does it?
[00:35:42] David: No, no, no. Big bets are not silver bullets. They’re fantastic. No, don’t get me wrong. I am a fan of the big bets approach. This has come out of, you know, places like Rockefeller Foundation, Ford Foundation. This is a lot of the big foundations trying to see how real change happens in the world. And one of the examples which has come out of the Gates Foundation on this was eradicating polio. That was the sort of big bet, which, to sort of say, we should do this worldwide and we should eradicate polio, that’s something we should just put the resources on and we should do. And it’s a well documented success case of a Big Bet where polio is a terrible, terrible disease.
[00:36:21] Lucie: Absolutely.
[00:36:22] David: Which has been, to the best of my knowledge, at this point, broadly eradicated through this Big Bet and actually saying, we can do this.
But there are elements of cost on that when you look at the details where you say, actually, maybe if we had done this systemically, rather than just as a big bet, maybe it would have been better. And I’ll just take one example, which I’ve heard quite a bit about in different contexts, which is that actually sometimes to recruit the nurses and the people to give the polio vaccines, they were recruited out of jobs where they were serving more people and having more impact, because there was a skills shortage in certain contexts.
Now this didn’t happen everywhere of course, because not everywhere had a skills shortage. But in certain places it has been documented that that big push towards polio eradication came at a very high human cost because of the skills shortages, which meant that the human effort to do it came at the cost of the human effort that was actually happening elsewhere.
And that to me is the danger. This isn’t silver bullets at all. But this is the danger of big bets that actually, if you’re not looking at the systems correctly…
[00:37:37] Lucie: Yeah.
[00:37:37] David: …your big bet can achieve what you set out to achieve, but at a cost which you didn’t imagine because of the knock on effects.
I don’t have the answers to this, but I do believe in really systems approaches where you’re actually recognizing, in my mind, you know, in my mind, I got to be careful how I frame this, but you can really change the world with a big bet. But how is it going to change? What are the knock on effects? And so on.
[00:38:10] Lucie: To me it sounds like a tension between local and sort of global there that, it’s working together for the greater good, but ignoring the sort of smaller, I don’t say evils, but, smaller problems or issues.
[00:38:24] David: I think there is an element of this and I would argue that the Big Bets is a very much a global approach and you need it to be done globally and that’s good. But not to recognise the value of local and to not try and build that in, and we’re really all about the local, we really believe that the local approaches and local change bubble up and actually scale out in much more powerful ways. And actually there are things to be done to stimulate and to enable that.
So if your big bets are happening at the cost of local, then suddenly the impact may be more negative than you could imagine for reasons which are just, if you want, consequential of details of the approach you take, which could have been changed. You know, you could have made different decisions and that would have led to very different changes on the ground.
And so this is where I feel that combination of local and global is so important. And Big Bets to me is great as a global thought process and a global approach, but it really needs to be embedded into approaches which are more systemic. And I would argue that ODK for digital data collection, they weren’t a big bet.
[00:39:39] Lucie: No, exactly. So did it start smaller then? I mean, it started more locally?
[00:39:43] David: Well, it started solving specific things in a particular way which enabled scaling, which was different to viral scaling. And it removed barriers, and this is the key thing, it removed barriers to people getting on board in ways which were so meaningful.
And what I think is also important is some of those barriers weren’t removed by the core team. This is where Honor came in, it wasn’t Honor at the time, but the people behind Honor came in and built the XLS form, which removed another barrier. And in my mind, that was a barrier which then changed the game.
And it can be those little details, which aren’t big bets. But they’re getting the details right. The importance of actually having communities working together, getting details right, innovating alongside the big bets. And big bets with good intellects behind them is great. It’s a wonderful intellectual exercise.
As I mentioned before, maybe my intellect isn’t what it was, which is maybe one of the reasons that I’ve left academia and I like to get my hands dirty and try to actually be more of an innovator. Even though I probably have more respect for the academics. I have less respect for myself than I do for academics, in that sort of sense, but it’s, it’s complicated.
Maybe we’ll end up cutting off this end bit, because it was a really nice end bit to do with Baoua. We’ll see what others decide. If you’re listening to this listeners, then they didn’t decide to get rid of the end bit, and I’m sorry you’re not finishing on a happy note. Let’s see what happens in the editing.
[00:41:21] Lucie: Yeah, thank you David for an interesting discussion.
[00:41:24] David: Thank you.