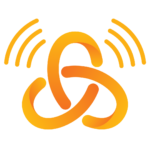
Description
We’re used to participatory research with farmers, but what does scalable participatory farmer-focused research look like? Lucie and David discuss this principled approach to agroecological research, which has the potential to not only create great benefits for researchers and farmers alike, but to also shake up research systems and power dynamics between researchers and the community.
[00:00:00] Lucie: Hi, and welcome to the IDEMS podcast. I’m Lucie Hazelgrove Planel, an anthropologist and social impact scientist, and I’m here with David Stern, a founding director of IDEMS.
Hi David.
[00:00:18] David: Hi Lucie. Looking forward to a discussion. What are we on today?
[00:00:22] Lucie: Today I’d like to discuss how a lot of the projects we work with actually do research, because there’s a concept that we all use and I know at IDEMS we’re really motivated in and believe in, which is Farmer Research Networks. So could you just sort of say a bit about what these farmer research networks are?
[00:00:43] David: This is not central to all of our work, of course, because we don’t just work in agroecology. But within the agroecology work we do, this has emerged as a topic which is really exciting, I find. This has come out of the work we do with the McKnight Foundation on the Global Collaboration for Resilient Food Systems, CRFS. And our role there is to support research. We offer research method support for grantees of all different sort of types who are doing research in agroecology.
And over the last, almost 10 years now, this idea of farmer research networks has emerged, in that context, where a lot of the research programs are trying to be more farmer focused and so they’re integrating elements of farmer research which is well established beyond farmer research networks. They’re bringing in elements of participatory action research, sometimes called PAR.
[00:01:52] Lucie: Right, so when you say farmer focused, it’s not just that it’s research for the farmers.
[00:01:56] David: Absolutely.
[00:01:57] Lucie: It’s research involving farmers then too.
[00:01:59] David: Absolutely, and this is sort of learning from sort of PAR approaches. And some people would feel there’s no real reason for farmer research networks as a tool, because actually this is just an instance of participatory action research. I wouldn’t necessarily disagree with that…
[00:02:16] Lucie: But what is the difference then?
[00:02:19] David: I would argue that the principles… There have been articulated principles for farmer research networks, and those aren’t the principles of participative action research, which is much broader. It’s a more narrow application of that to farmer research in a particular set of contexts. But I don’t think the principles even capture the ideas of farmer research network as it’s emerged, and what’s really interesting is this is, in my mind, one of the few examples I have where we went through a process of actually defining principles, but I’m not happy with the outcome. You know, we never got to coherence. We never got to agreement.
[00:03:05] Lucie: We should just say that you can find a list of the farmer research network principles on the McKnight’s CCRP website, and I say CCRP because the web address is CCRP.
[00:03:15] David: That’s right, it used to be the Collaborative Crops Research Programme, and was recently renamed to the Global Collaboration for Resilient Food Systems.
[00:03:24] Lucie: So I’d be really interested though to know how do you define farmer research networks, let alone how other people do it, how do you do it?
[00:03:31] David: Well, let me just keep going on this principle because I think there was an interesting element of controversy around that, not controversy really, but discussion where there was a principle which is not there, which some of us associate to farmer research networks as being essential. And that was an idea called embedded scaling. And one of the reasons that this didn’t make it into the farmer research network principles for the program as a whole is that it wasn’t clear that this was appropriate in all regions or in all contexts, whereas there were elements of the participatory action research approach which were clearly applicable everywhere.
And so, a more general term was useful to get the cross learning that I think is valuable. But not having that principle of embedded scaling, to me, removed the uniqueness of the farmer research networks. And so, when I really think of farmer research networks, I include embedded scaling as a principle. And then the principles that are there, plus embedded scaling, roughly do it for me.
Then I feel I’m comfortable, I know what I’m talking about. And so maybe I should just talk about why this embedded scaling part of the farmer research network approach, and how this has played out in a couple of different contexts, and why that’s so powerful.
[00:05:02] Lucie: So most participatory research doesn’t need to be scalable.
[00:05:05] David: No.
[00:05:06] Lucie: This is the main distinction, is it?
[00:05:08] David: I saw originally, no, I was not the originator of these ideas, a lot of this actually originated from people like Ric Coe, who were really one of the thought leaders in this area. And when he first presented some of these ideas more broadly, it was all about what he was talking about at that point, which was large N trials and how farmer research networks could be used as a way to really create not just large N trials, but participatory large N trials.
[00:05:44] Lucie: And large N is when there’s lots of people involved, quite simply, N for number.
[00:05:47] David: Absolutely, N is the number, and it’s a large number. So, most traditional participatory research is on a small number. And so really the distinction that I’ve kept and that I have in my head, when I think about what I really believe in as a really interesting new approach, is this idea of being participatory at scale, and actually being able to do participatory research at scale. And that’s, I think, a very useful way of formulating and framing what I understand as farmer research networks.
So let me just take a couple of instances of this which I found inspiring for me. I’ll start in Niger because I love Niger. I grew up in Niger.
[00:06:36] Lucie: Everything starts in Niger.
[00:06:38] David: For me it does anyway. It is where my heart still lies. And there’s this amazing farmer federation in Maradi, which is a region of Niger, sort of central south. And it’s the federation called Fuma Gaskia. They were the first farmer organisation to be awarded a research grant by the McKnight Foundation. And this was a big deal, and this happened back in 2012, over 10 years ago.
[00:07:16] Lucie: Yep.
[00:07:16] David: This even predates me in terms of this, I only joined a year or two later! So they’d already had their first sort of trials gone out, and I joined them when they were struggling to analyse that data because they didn’t choose their treatments perfectly, and so their treatments weren’t really comparable.
But they’d done some amazing work already, identifying human urine as a potential and its potential for women’s fields in their region. And what they’ve done over the 10 years since is just incredible. But when they were doing this, they were doing this on-farm experiments, which has been around for a long time.
And they were working with farmers who were trying out a number of different treatments. And what we did actually over the next few years is we helped them simplify the treatments a bit so that they could have better comparisons. And that then led to them actually getting good evidence that, yes, human urine, as they were able to use it locally, was able to have a positive impact on the yield in these low resource environments on farmers fields. And so they got some interesting scientific evidence of that.
But I suppose the most important thing that happened was that as they went through this, they were really struggling to manage their data. And they were really struggling with the research process. It actually took years to get that paper published. And in our role, we supported that a bit. And I was very grateful to be part of such an interesting piece of work. But it wasn’t really until their next phase, they’d gone through a sort of first phase of funding and as part of the next phase they came to me and they said we need to take the data management seriously, because this is how we’re going to be able to do better trials and actually engage the farmers in a different way.
So they ended up employing a computer scientist. Again, I was very privileged to be part of the interview process and they had a very bright candidate who they recruited. And he wasn’t just a very bright candidate, he was also someone who wanted to go back to the rural areas and work in the rural areas. He had personal motivation which aligned with this. And he did some exceptional work.
Most notably, what he enabled them to do was to have the data which was collected through the mechanisms of the Farmer Federation using software which he developed to do trials with thousands of farmers. So they went literally over a few years from tens of farmers to thousands of farmers because of the support that that gave.
And I was privileged to accompany them in this process, and there were some wonderful moments, really formative for me in terms of thinking about why this approach of farmer research networks seen from this idea of let’s say a farmer federation actually owning the research.
This ownership is key here. They quite early on had a number of collaborators from the local university who were supporting them, and some of them had engaged extremely well and some of them were trying to impose their methods on the federation. And my role was quite often to try and help navigate and find a way to support the federation to do the things that were meaningful for them with the researchers.
So I remember sitting in a meeting where they were trying to do a farmer typology to help understand some of the results they were getting. Different farmers have different results in different ways.
[00:11:07] Lucie: And farmer typology is sort of, if it’s women, if it’s men, how old they are, is it, how much income?
[00:11:13] David: Well, this is exactly the point. I mean, when you take it as a sort of standard process, this can be really detailed. You can get a lot of data, which you then use. You can do farmer typologies in many different ways. And you have the social scientists debating the different ways of doing it. And the main thing that they got onto debating was, you know, how big should your sample be? And one of the colleagues who was there, Aminou, looked at me and I looked at him and I knew what he was thinking and so I said, Aminou, how many of your farmers are you wanting to have do the typology?
And he said, all of them. And the researcher said, no, no, you can’t do that, we need to take your sample. And I said, well, you can, that’s just a census of his farmers. So it’s just a slightly different method. Oh, you’ve never done a farmer typology as a census before. And so, actually that idea of thinking about what it means and why you’re doing it.
Now, of course, for the federation, this information for all of the farmers is useful because it helps the farmers themselves. They’re not collecting this information for the researchers, they’re collecting it for themselves. And once you started recognising that the main aim, in some sense, was to have data that would be useful for the farmers, and the fact that you have a farmer typology to help differentiate your different types of farmers, is primarily to serve those farmers, but also useful for research, then you start to understand the change in thinking when you change the ownership of the data, when you change the ownership of actually the research approach.
By giving that ownership to the farmer federation and having the researchers engaged, then not only do you get different data, but I would argue you get better data. Because the typology now, should be, if it’s done well, and if the Federation does it well, it should be in each farmer’s interest to be classified correctly, because that will mean they get the right help and support that they need, at the right times when they need it.
And so, you’re actually getting better quality data, because the incentives for the data are correct. The federation is there to support them. And the better data it has to understand their situation, the better it should be able to support them. And that’s exactly how this was framed. And similarly, when you actually got down to it, the researchers get better data at the out as well.
That they could actually get data, not just on more people, but also over time. They could be seeing how things change over time because of course it’s in everybody’s interest for their information to be kept up to date. And so once you change the nature of the data and the data that’s collected, you actually get a much better data source.
Now of course that collaboration needs to be established. What are the researchers going to use that data for? How are they going to use it? Is it going to serve the farmers? Well that now lies as a negotiated process where a lot of the power is with the farmer federation. So in this particular case this idea of a farmer research network basically played out as the federation providing the networking between farmers, between farmers and researchers, and between other actors with the farmers in different ways. The federation was the central sort of actor helping to facilitate this with negotiated protocols, with negotiated research agendas.
And what’s so powerful is over the years, this research has now been institutionalized. It’s no longer a project which the Federation does. It is part of the Federation activities.
[00:15:10] Lucie: Yeah, yeah.
[00:15:11] David: And projects come and go to supplement the research they do. But the research process, this sort of ability to work with its farmers and to do research is now embedded in the Federation structures. It’s an incredible achievement.
And that is so inspiring to me in terms of just recognizing that, well, as soon as you start thinking that of the research collaboration like that, the researchers had to adapt, because once you are asking all the tens of thousands of farmers, you couldn’t have a long survey, which took three hours, you know, you can’t do that. You had to rethink the data you would use and how you would use it.
And so they actually got it down that the initial data they collected was just 10 questions. And they tried to use that to help sort of do an initial typology. But the beauty is, they could then add different data over time.
So you start with those 10 questions, but…
[00:16:10] Lucie: You get people hooked.
[00:16:11] David: You get people hooked. And then, you know, if people are interested, then over time, if there’s more information, which is needed, because you’re not differentiating between certain sets of farmers, which you need to differentiate between because it would be useful to differentiate between them, then you actually can get more information over time. But you’re never doing a big long questionnaire. You’re always just having that continued interaction with the farmers because the data is theirs and it is useful to them.
That change in thinking is so important. And I suppose this relates a little bit to the study types we were discussing the other day. The point is, why do you need to do sampling? You need to do sampling because you’re doing a survey. You’re doing a survey because you want to represent a population.
[00:16:59] Lucie: Yeah.
[00:16:59] David: Now we’re talking about an opportunistic study.
[00:17:02] Lucie: Exactly, it becomes opportunistic, yeah. Because the data is being routinely collected, and so it is there, and it just needs someone to actually be interested in it, to analyse it, and see what it has to say.
[00:17:13] David: Exactly. But what’s really exciting, of course, is you’re combining that with experimentation. And now your experimentation, you can do experimentation at scale, because you can reduce the needs of this. And so they’re doing these experiments, very simple experiments, often just literally comparing two things, or three things. And they’re doing it at scale, in a way which is so interesting, and what’s fascinating, of course, is this has totally transformed the interactions.
All the other research projects in that part of Niger, who are part of the same community of practice, now work with them to do the farmer experimentation because of the data that they have and the way they can do it at scale and they can now do variety trials in a way you couldn’t have compared varieties so easily before.
And if you wanted to do variety trials at scale, they either had to be very structured or they had to be very small, it was hard. Whereas now they have real power at being able to do research at scale within this framework that they’ve built, and where they have very interesting contextual information about the farmers, which doesn’t need to be collected every time.
And so it’s a much more respectful interaction that actually the research doesn’t just help the researcher, it also helps the farmer federation and that’s negotiated. The power dynamic change is fantastic. So I’ve gone on and on about this example and sorry I’ve just talked and talked here.
But, you know, this has been inspirational for me and when somebody says FRN to me I think of Fuma, first and foremost.
[00:18:54] Lucie: Okay.
[00:18:54] David: What they’ve done and what they’re doing. And there’s many ways in which they’re still evolving. It’s only very recently that they’ve got to negotiate the treatments with the farmers in ways which are more participatory, because they were first set up to look at one thing. Whereas that sort of part of the principles of FRN is that all steps are discussed with the farmers. And so it’s taken a long time to get to where it is, but that process and that embedding in a farmer institution, this is what I’m inspired by and where I see that data at scale, potentially enabling farmer experimentation and real research knowledge to be built, while also serving the community that is the network of farmers and so on. That’s what I see.
In practice, in most other contexts, farm research networks, even within the McKnight’s projects, have taken many different forms. And the others are also very interesting. There’s been some really interesting work on East Africa with a group that’s done amazing community restoration in incredible ways.
[00:20:08] Lucie: The fields, community restoration of fields.
[00:20:12] David: Well, sort of.
[00:20:13] Lucie: Or the community itself.
[00:20:14] David: More than that, the whole water balance of the community was changed because they did things with dams, they actually did incredible work restoring landscapes. This is the drylands project in East Africa, incredible work that they’ve done. And they would consider their approach as being very much an FRN approach.
They sort of worked with the principles, they were trying to do this and they were thinking about the problems in a very collaborative way with the community. They don’t have the same ownership of the data, which, I must admit, I really like in the Fuma case.
[00:20:45] Lucie: Exactly. So, from your explanations of Fuma Gaskia and what excites you about the way they work as a farmer organisation that does research, to me, there were several things. So one was I mean, data ownership, who owns data, and therefore who has power. I think that’s sort of starting to be a lot more recognised in academia, that data has power.
[00:21:09] David: Yep.
[00:21:11] Lucie: And that often its providing the data, who own it. The evidence too, that word came up a few times. And I think it’s making me think about research and what makes good quality research. So, I guess I have a question as to can farmers do good quality research?
[00:21:29] David: Farmers generally don’t care about academic publication. So in the sense of traditional research, their research is not considered good research in that context. But the question is, if we think of research more broadly, could you get academic publications from a very farmer led process? I believe yes. I believe you can get really high quality publications and evidence.
And so part of the question is, what’s the new knowledge? So in the Fuma case, is it surprising that human urine improves crop yields in low resource environments? No. There’s good research which predates this by a long way, which means that yes, this was almost certainly going to give a good result.
[00:22:20] Lucie: Yep.
[00:22:22] David: Could you have known beforehand that there was sort of a relatively constant result, whether you were a low fertility farmer or a relatively high fertility farmer?
[00:22:38] Lucie: In terms of the soil fertility?
[00:22:40] David: Yeah, in terms of the soil fertility. If you have, relatively poor soil fertility, and so you don’t tend to get much yield, or you have a higher soil fertility. What they seem to show with some of the data, which I saw in the past, was that actually, the amount the additional top dressing sort of human urine gave, seemed to be relatively constant. There seemed to be a relatively similar absolute amount which was increased.
Now, of course, that means that if you’re in a low fertility environment, relatively speaking, you’re doing much, much better. This could be a 50 percent increase in yield. Whereas in a higher fertility environment, it might be less than 5%. You know, the importance of that might not matter so much if you already have high fertility. And so those are the sort of questions where I don’t know of the scientific literature, and not just me, I mean I don’t know anything about how plants grow… I know a little bit now, I’ve learned a bit, but, but really it’s not my area of expertise, it’s not surprising I don’t know.
What was interesting to me is that experts didn’t know. They didn’t know what to expect, would you expect this to be proportional or would you expect it to be a constant gain? And, you know, experts weren’t sure, they could say, oh, I don’t actually know, in this sort of environment, I can’t figure it out, you know, I don’t know. So there are hypotheses which are emerging.
Now, of course, I want to be really clear here. It is only a hypothesis which emerges because it’s an opportunistic study, even if it is experimental in that sort of sense. It’s in a highly variable environment in different ways. If you really wanted to be certain of this, and to understand the processes, then you might need a more laboratory based experiment to then back up that evidence. But fantastic to know that this is meaningful.
[00:24:33] Lucie: Just a second then. Because I’m thinking of our previous discussion about study types and experiments were the only way of identifying causality.
[00:24:43] David: Yes.
[00:24:44] Lucie: So you’re saying that farmer research networks can’t identify causality?
[00:24:48] David: No, I’m not saying that, but I’m saying there are questions or hypotheses which can emerge which are not related to the comparison in the same way. So, for example, whether there is a positive effect due to human urine, yes, the experiment is set up to check that and it can determine that there’s a causal effect, that yes, there is a positive effect due to the addition of human urine as a fertilizer. But that was already known. So that’s not a surprise. So the fact that the experiment confirms what was already know, isn’t necessarily interesting.
What is really interesting is that by doing the experiment through this sort of large thousands strong farmer research network, you now emerge with new hypotheses. The hypothesis that the human urine addition top dress fertilizer is actually adding a constant amount across different levels of soil fertility.
That’s not a hypothesis that we had before. Now, to get evidence that that is actually the case, probably a farmer research network isn’t the right tool for it to do that. That’s the sort of study which you might want to do in the lab to understand the process of why it is a relatively constant amount.
It might not be, but if that’s the hypothesis, then that experimentation is not the right tool to really get evidence for that hypothesis, you’d want to go into causation of it and you’d want to therefore understand what the differences are which you’re observing and why, you know. It doesn’t actually make sense, it is slightly counterintuitive to me, and I don’t know that this is the case but it’s what seems to be emerging relatively consistently, I haven’t seen the latest data, maybe it’s no longer emerging consistently, it just emerged from the data that I observed.
But what’s really interesting about this approach is that now, because of the scale you’re at, as well as being able to actually determine causation for a process because of the experimentation, that might not be the most interesting results. Your secondary results can be much more interesting, actually identifying, let’s say, maybe, and I didn’t see this in this case, but we’ve seen this in other cases, groups of farmers, where they get a different behaviour.
So for example, in East Africa, with a farmer research network there, there was this fantastic work on sorghum varieties, where then there was this problem because in certain regions, they weren’t getting the results that they were expecting, and they figured out that this was because of aluminium toxicity in their soils.
And so they then went back to the lab and they actually understood, and they did some experiments in the lab, which helped them to recognize the difference between different varieties and their resilience to aluminium toxicity in the soil. And so actually, you know, just because you’re doing these things at scale, it’s generating hypotheses as well as answering certain hypotheses.
But the real power is if you want the observation part of it, you know, the opportunistic part, then actually once you’re having that larger data set with these additional components, you should be now generating interesting new hypotheses by treating this in some sense, not just as answering the experimental question, but as using this as a way to then do a opportunistic study and identify new, better questions to ask, or other interesting questions to ask.
And that’s where this becomes so powerful as a research approach. Now, coming back to how does this relate to high quality, good international research? Well, it’s very different to what we work on with our Oxford University partners and our partners in sort of very prestigious international universities who tend to have research trials which follow a very fixed format, coming out of particularly if you want the medical research, you know, these randomized control trials.
There was a Nobel Prize for economics to Esther Duflo for applying this methodology to development. And I’m not saying that this is wrong, but I’m saying that it is just part of what is needed. And actually the opportunity that I think FRNs present is demonstrating that actually, if we think about not just participatory action research, but how to do that at scale, farmer research networks potentially provide a roadmap, not of what this is, because there’s no coherence across them, it’s just learning. But it does provide a roadmap of, wow, if we do consider research differently in certain ways, we might be able to get to different types of results.
And that’s something I’d be so excited about. And the key to this embedded scaling, and the reason I’m so insistent that for me that’s central to the FRN process, is that the embedded scaling is exactly what breaks down the barrier between research and development. Or implementation, implementation is a better word.
So if you think about embedded scaling, what that is telling you is that actually as you scale it, the research should scale as well, so the data should scale as well. So think about now, you do a trial in Kenya about an education initiative with a particular technology, and then you get the technology out at scale, the research shouldn’t stop.
Actually, as you implement, as you get out at scale, that’s now the research network just expanding and you’re finding ways to enable people who are involved, maybe the teachers involved, to be part of that research network. This is the sort of concept which I think has come out for me as a learning of embedded scaling within FRNs as an opportunity for changing research more generally, to be more impactful, because you can really embed the research in implementation, and you can embed implementation in the research.
This is the thing, so the research at scale that Fuma are doing, or that this group in Kenya who are doing the sorghum varieties are doing. That’s already a dissemination activity. By doing research at scale, you’re actually already exposing people in those communities to the ideas.
[00:32:14] Lucie: Well exactly, that was going to be one of my questions. What actually do these farmers get out of the research?
[00:32:20] David: The reason they’re interested in it is because it serves them! They want to know the answers.
[00:32:27] Lucie: Okay.
[00:32:27] David: So they want to know, you know, is this new variety which someone’s proposing to me better than the varieties I’m already growing? Or are my varieties better? And so they’re answering that question for themselves. And the researcher is getting the data on that. And that’s the whole point of this embedded scaling. The normal approach is: you determine which variety works well, and then you vote… the French word is vulgarisation, which I really like. I like that much better.
[00:32:57] Lucie: You vulgarise it, you make it vulgar.
[00:33:00] David: You make it vulgar. I love that. It’s a fantastic term. But, you know, that is the normal process that you then disseminate and people adopt your learnings. That’s the traditional research approach. And it’s broken, it doesn’t work well. What you want to do is you want to be going out and adapting as you go. People talk about this, you shouldn’t be having adoption, you should be having adaptation. But if you’ve got adaptation, you need to embed the research in that process.
And so that you’re learning as you go, because you want to be able to learn from the adaptations that happen. So this whole discussion in development in general about adoption versus adaptation is all tied in with this. And I believe there are concrete learnings which are coming out from these FRN, farmer research networks experiments, as I would put them.
I shouldn’t be calling it experiments, but it’s sort of what the learnings which are coming out from these experiences, experiences is a better word, that are happening. It’s not that FRN is necessarily something which is going to become big and lots of other people are going to adopt.
But I do think that the thing which is going to become big, and a lot of people will adopt is research processes which were not about adoption, but are about adaptation. And I hope they don’t just adopt it, I hope they adapt it.
[00:34:21] Lucie: Yes. Because, as you said, there are many different ways of doing farmer research networks or being a farmer research network.
[00:34:30] David: And as I say, I’m not happy with the farmer research network principles because it doesn’t have embedded scaling. I would be happier with it if it had embedded scaling. This is just, you know, my personal opinion. The point which is so important is that I do like the fact it’s principle based. And so, the fact that there are principles means I can explain my disagreement. So, you know, arguably, the difference I would like to see the FRN approach, it would be FRN plus embedded scaling with the current principles.
And that’s exactly why a principle based approach is so powerful. That you can actually voice and argue about the differences and discrepancies. Now don’t get me wrong, I absolutely understand why within the program, the McKnight CRFS program, I understand why it is what it is, because embedded scaling isn’t right for the learning they’re wanting to get. It’s just what I believe in personally. It’s what I’m excited about.
And I do think that, as an entity, it makes sense if you add embedded scaling. It might just be that that’s what I’m passionate about, but I do think that there’s experiences coming out which are worth learning for which relate to this.
[00:35:46] Lucie: And I look forward to getting more people on this podcast series who can debate those ideas with you about farmer research networks, what it means and whether they’re good for something or not.
[00:35:57] David: I have to confess the people I have in mind who will probably be on sooner rather than later, they agree with me about embedded scaling. So it’s not going to be the debate that would be most fun. I should get some of the people who don’t agree with me on that to have some good debates. But anyway, that’ll come later, I’m sure.
[00:36:15] Lucie: Okay, thanks David.
[00:36:17] David: Thanks