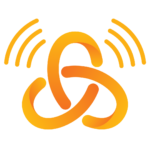
Description
Lily Clements and David Stern discuss their recent collaboration with a PhD student on complex data modelling. They highlight the challenges of accurate statistical interpretation, the importance of responsible research practices, and the need for more accessible modelling tools. Their conversation underscores the significance of improving data skills to enhance the reliability of research and AI applications.
[00:00:00] Lily: Hello and welcome to the IDEMS podcast. I’m Lily Clements, a data scientist, and I’m here today with David Stern, a founding director of IDEMS.
Hi David.
[00:00:16] David: Hi Lily, looking forward to another discussion today.
[00:00:19] Lily: Yeah, me too. I thought that today we could discuss about some work that we’d recently done with a PhD student in kind of supporting her.
[00:00:27] David: Absolutely, it wasn’t a big piece of work for either of us, but it was something where I learned a lot from this.
[00:00:34] Lily: Me too, absolutely. And I think, just to start it off, maybe we’ll just give a brief background. This was a student, she did a kind of experimental design and was writing a paper on the results.
[00:00:45] David: I think we should start by stating this is an extremely strong student who has been writing her own R code and doing really impressive modelling and you’ve been particularly involved, I’ve been a little bit involved on the periphery, and she’s had other support from the statisticians supporting that project, if you want. You know I’ve supported lots of PhD students and researchers all over the field in many different ways. And as a non statistic specialist, she’s about as strong as I’ve ever had. So this is, this has been a really fun project to support.
[00:01:22] Lily: Absolutely. And as we were reviewing the paper it was actually our colleague, Esmee, who is a mathematician, and she’s a very good mathematician but she doesn’t know much on the statistics side because she focuses more on that mathematical side, and she noticed, okay, how come this number here isn’t the same as that number there?
[00:01:42] David: Broadly, yes. The interpretation of that number there, I thought, would correspond to this number up here, but they don’t seem to correspond. So she picked up on that. And I should clarify that the study that had been done was a really rather complex study with something like a thousand people, 600 people I think it was. But you know, it’s a sort of large complex study. And so that meant that there was complexity in the model.
[00:02:09] Lily: But when she flagged this, she then had a meeting with me and you and we were like, okay, actually, I think we should discuss this with the PhD student. And we had a very interesting discussion there and then dug into it quite a bit over the course of a few hours and eventually found that ultimately the problem was that something was being timesed by two.
[00:02:29] David: Well, there were a few little bits different, but yes, it was a trivial thing where there was a slight misinterpretation somewhere deep in the modeling which had led to the problem. And it wasn’t that the end result was timesed by two, but there had been a process in the middle where there was a problem. So full credit to Esmee who identified that indeed these numbers should have lined up in a way where they would have made sense to her and they didn’t, and she picked that out.
And this is not uncommon. I mean, let’s be clear, even if this had got published. There was a nature paper a few years ago, which I’ll let you describe.
[00:03:06] Lily: Well, yeah, so my understanding of the paper a few years ago was that it was at least 50 percent, I can’t remember the exact number, but at least half of papers published in top, top journals, have their statistics as kind of misinterpreted or incorrect. I can’t remember which phrase they used for it there either. And I’m sure that we could find it. But either way, quite a scary statistic, I suppose for me, when I was first told that statistic, it was very scary. And now as I’ve kind of progressed over the last few years, you know, finishing a PhD and into statistics, and now into data science, it’s kind of not surprising.
[00:03:42] David: Yes.
[00:03:43] Lily: Or not as surprising as it should be.
[00:03:45] David: Well, it is not surprising at all. It’s part of what’s so interesting and difficult about this. And I think this particular case is a really interesting one in point. Because, okay, the end conclusion of the paper didn’t change with this slight misinterpretation. But the extent that that particular value that was being reported on did. And so that specific result, or there were specific results where the values actually changed in the way they were. Now the interpretation of the results were exactly the same.
But what that meant was, this was exactly the sort of thing that was being picked up in over 50 percent of papers, where there was, in this case, a very competent researcher having to use a really difficult and complicated modelling process because of the nature of her research and then getting tripped up along the way and it being very hard, despite the extensive amount of support she had, to actually notice that this has happened. The fact that she was tripped up was, in my opinion, not her fault at all. This was just, sort of, the tools aren’t really there to help this, and therefore the expectation, the bar, is set so high.
This is happening very regularly, and that’s exactly what the paper in Nature sort of pointed out. So, the point which I suppose is so important in this, and the real reflection and learning for me, is, well, if this is happening here, in this context where there is so much support, and so much skill already, what chance do we have of enabling research to be happening responsibly at scale?
And that is what’s happening. There’s research which is happening at scale, and we often talk about responsible AI, but we should also be thinking about responsible research. And I suppose this also comes down to, for you, you have moved to much more working in the responsible AI space, data skills, but actually thinking about responsible research is also important. And enabling that is hard.
[00:06:03] Lily: Absolutely. And I think we’ve worked on various courses and writing various courses on data skills when it comes to things unrelated to modelling. But when it comes to modelling, we’ve got some courses that we’ve worked on, but not as much. And that’s I guess because there’s a lot there to cover, but even the basics are still the same.
[00:06:22] David: I think the point which I’m really loving is that I think that there is possibility to work on this, but it’s hard. And I’m hoping you might get tempted to do some of this. You know, people haven’t been working on this for long. I know papers from 1973, where they started thinking about these things. And I think that wasn’t the first set of people. You know, it’s only 50 or so years that people have been thinking about this, but I think you could actually make an impact on this.
[00:06:49] Lily: Well, and I’ll be honest here, when we came up, when we found this problem with the PhD student and we volunteered me to have a look at it.
[00:06:59] David: I like that phrasing. I think you were volunteered rather than you volunteered and I apologise for that.
[00:07:06] Lily: Well this is why I’ll be honest, that was not something I wanted to do, because it is hard, because it’s something which is out of my comfort zone, it’s something I don’t understand as well. And I know that it’s something I don’t understand as well. As someone with a PhD in statistics, I don’t want people to know I don’t know that. I mean, maybe I should be saying that on a podcast.
[00:07:24] David: Nobody’s listening to it anyway, so I wouldn’t worry.
[00:07:27] Lily: No, but I also don’t mind saying those sort of things. But you know, the kind of instinctive reaction is always, oh, I don’t want people to know that I don’t know that. But actually, when you then think about it, it’s like, oh, I don’t mind people not knowing I don’t know that.
But when this task was given, and when they found this problem was identified, it was, gosh, how? What am I meant to look at here? How am I meant to do this? Modelling is hard and there’s so many different complexities there, there’s so many different things and I think that there’s definitely a a cause, we would really want modelling to become more approachable somehow.
[00:08:00] David: And I think the point that I’ve often had, and I’ve had this discussion with statisticians all the time, when we’re working on projects which try to make it easier for people to use models or do things, and they say, I don’t know if we really want to make it easier, because then they won’t understand what they’re doing. You know, modelling is hard.
[00:08:17] Lily: I suppose, well, okay, so you don’t want to make it easier?
[00:08:22] David: Well, you know, particularly it’s discussions which revolve around, well, should you be having front ends to make it easier versus should you be having to write code? And one of the arguments is that actually the barrier that writing code provides is actually maybe healthy because it stops people thinking they know how to do it when they don’t. And so it’s putting a barrier in there which could be useful.
Now I personally disagree with that. I want to make these things more accessible because I think the barrier of writing code is not the same as the barrier of actually comprehending and being responsible in what you do.
But the truth in what they say is, well, actually, if you want it to be just press a button and it works, I’m afraid that’s not, it is hard, it does need sort of help, and it’s not the sort of help which I think is going to go away with AI anytime soon.
[00:09:14] Lily: That’s true, yeah, that’s true. And I suppose that, you know, you could fit a very simplistic model, or you could fit a more complicated model more tailored to your situation, but either way, most of the time, or a lot of the time the model will still fit. It’s not going to tell you, okay, this is fit, but by the way, here’s a big error. You know, that’s up to you to then find.
[00:09:37] David: Well, and maybe, maybe AI could help with that in the future. Maybe there are elements where we could develop AI to help people be more responsible in their modelling and their research. I think this interesting sort of thinking about uses of AI, which could be different. One of the things which I feel that you’ve been exposed to now in some sense, moving more towards data science as you have you’ve sort of been able to look back at statistics with a little bit of a lens of an outsider again, rather than, you know, as a statistician. And I think that’s been useful in this. Do you agree with that?
[00:10:14] Lily: I do agree in that. Yeah, I do. I do definitely agree with that. It’s been nice to look at those bits of statistics and be like, okay, good, yeah, good luck. Or maybe not good luck, but, but seeing the kind of… yeah, the, the, the things there and… Then with this problem here being dragged back into models, I thought I didn’t want to be in this, I mean, of course, data scientists also need to know modeling and stuff.
[00:10:37] David: But these details, you know, the point is that at the end of the day on this, this particular result there’s the element, very simply, that using the correct, not the correct model, using an appropriate model compared to a less appropriate model…
[00:10:55] Lily: Not the correct model because there is no correct model.
[00:10:58] David: Of course. Yes, I picked up my mistake and thank you for helping the listener to understand why I picked up on that as being a mistake. But yes, these are elements that an appropriate, a more appropriate model, the p value, which is what you use to determine whether or not the results are statistically significant and hence trustworthy became less significant. And so therefore you are less confident that it was trustworthy. And therefore if you’d used the simple model then you might have believed you have more evidence than you actually have with this particular trial.
There was quite a big difference. It was still statistically significant and hence trustworthy, but much less so than using the simpler models. And so, one of the things there, which I think is so important to take away, is that you don’t use more complicated models necessarily, in most contexts where people are using modelling.
The more complicated models don’t show there to be more evidence for what you’re doing, they often show that there’s less evidence because you’re not making assumptions which are incorrect. This is the sort of tricky thing. I think that there could be a case where if we turned around how people use modelling, and this is what they’ve tried to do in New Zealand with the schooling system there, then you could start with models which are less significant because they have less assumptions.
And then you would not need to use other things if there was enough evidence there. But if you then use more complicated models, you’d be doing so because you are saying, well, I believe I can make these assumptions. And that’s a really different approach, but our tools are not designed that way.
It would be amazing to design the statistical analysis tools in such a way that the default modeling processes were ones which were, which had less assumptions, not more assumptions.
Am I even making sense?
[00:13:10] Lily: Well, you’re making sense to me. So you’re saying that the tools are not designed that way. Do you think that that’s a matter of time before the tools are designed differently?
[00:13:19] David: Well, statisticians also don’t tend to think that way, because historically, the tools to have less assumptions were developed later. So we tend to teach in almost a chronological order of how the methods have been developed. Actually, the methods to have less assumptions, those are more recent methods in a sense, some of them are not that recent, but you couldn’t have applied them so easily in a general context.
So I think, I don’t know of anyone really trying to build those systems, other than maybe me, but I’m not actually actively working on it. I do have a few other people who are sort of thinking about it. But the New Zealand group, as I say, they have thought about this. And this is where, when I first heard what they’d done with the curriculum, I said, no, that’s silly. Why would you do that to the curriculum? And I’m now a total convert.
So I’m certainly not the first person to think like this, whereas they’ve integrated it into statistics teaching and so on. And so in some sense, their tools, and there are tools built around that, are developed in that way. But these aren’t the big tools which people use.
So I don’t know. I think there is a possibility that there could be people who could just pick this up and run with it, and I’d love that, and I’d love to hear what they’re doing, especially if it’s open source. But yes, it’s not obvious to me and it’s certainly in education, I don’t know many other examples where they’d be brave enough to try that.
[00:14:55] Lily: Interesting, interesting. And is that… Is that a bravery thing? So why is that not kind of integrated in other places? We presumably wouldn’t integrate it as much into courses that we write because the software doesn’t follow.
[00:15:10] David: It’s one of the hesitations I’ve had in actually really developing the modelling parts of our courses and sort of building that out because I would love to do it. But I’m slightly scared of how big a job it is. Whereas, you know, other things I’m confident that we can do and we can do well in a short period of time, this is something where this could be a huge job. But I want to be optimistic to finish. I think the reason people haven’t done it isn’t that they’re not brave, it’s that in some sense many people don’t see the importance.
But my hypothesis is that actually with the importance of AI and the recognition of the need to be responsible with AI. I reckon that this could then trickle down to actually people recognizing that actually, if we want to be responsible with AI, we need to be responsible with data. And if we want to be responsible with data, we need to have tools which should enable people to be responsible using data in all sorts of different ways.
And so there is a hope for me that people could really latch on to this area of responsible data analysis or data skills to be able to enable modeling, which is done more responsibly, which would also then feed into more responsible AI. So maybe this is something where the responsible AI movement could push in this direction.
I think maybe I’m being over optimistic about that, but you never know.
[00:16:40] Lily: Well, there’s a lot of, yeah, there’s a lot of areas, I guess, that we want responsible AI to change things for the better.
[00:16:46] David: And I think there was a real question here that responsible AI doesn’t exist yet in my mind. I don’t know anyone who can do responsible AI fully, because the tools aren’t there and actually, you know, there’s so much complexity around this. There were people working on this and I love some of the work coming out of the Turing Institute on this. But it’s a hard problem.
But because it’s such a hard problem, and people recognize its importance, I think there’s an opportunity that this could actually really provide the, I guess the investment is the right word, it’s not just in sort of financial investment, but in terms of human effort, the investment of human effort into all the different aspects which relate to this.
And part of that has to be responsible data skills. So, I’m going to leave on that optimistic note before you convince me that there’s another angle to look at this and be less optimistic.
[00:17:40] Lily: No, let’s leave it on the optimistic note. Thank you very much, David.
[00:17:44] David: Thank you.