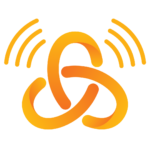
Description
Lily and David discuss the challenges of using AI to generate images, highlighting biases observed in the results – in particular, regarding race and gender. They discuss the importance of recognising and addressing these biases, proposing solutions such as creating specific guidelines for using AI tools ethically and advocating for diversity in AI development.
[00:00:00] Lily: Hello, and welcome to the IDEMS Responsible AI podcast, our special series of the IDEMS podcast. I’m Lily Clements, a data scientist, and I’m here with David Stern, a founding director of IDEMS. Hi, David.
[00:00:19] David: Hi, Lily. What’s the topic for today?
[00:00:23] Lily: Using AI to generate images.
[00:00:26] David: Oh, yes. That’s got lots of biases in at the moment, hasn’t it?
[00:00:30] Lily: Well, yeah, and I didn’t notice how much until I tried to use it to generate images. So, as you know, we create these different courses and the context is kind of farming in Africa or at least for one of them, one of these courses in particular, the context was farming in Africa. So I was generating these different icons for the course and I’d say, okay, generate for me a farmer or in fact, I think I was asking for an image of just some fertilizer and it gave a great image of a hand pouring out some fertilizer with a white hand. And I was like, okay, that’s perfect.
But actually I don’t want a white hand for this context. So I tried, I tried for a long time for at least an hour to get that image, but without a white hand of trying different requests, different ways of phrasing it. It would give a silhouette at points. It would give a huge background at points. In the end, I reached my limit my physical limit with ChatGPT. Not my mental limit, but my physical limit of asking too many questions. And it said, I have to wait another two or three hours to be able to ask again, at which point I just took that first image and manually changed the colour of the hand through my own ways.
[00:01:52] David: And I think one of the things which is so interesting in that is this element of, it was the first image that you took. I mean, I didn’t know that before you just told me, but it doesn’t surprise me that the first image was almost what you wanted, but it had biases which were not appropriate for the context within which you were aiming to work.
And this is, if I understand correctly, this is the courses we’re developing which are being used currently in Mali to train research methods support people in using data for agriculture. It’s that course, isn’t it?
[00:02:27] Lily: Yes, yeah, yeah, absolutely. It’s that course. And it was, the more I asked, the more specific I was with how I want this image, the worse it got, the less it gave me what I wanted. If I even just explicitly said, give me an African, an African farmer, it would then give a background with kind of flags and things. And it’s like, this is great, but I just want the hand, I want the aim to be on the hand, pouring out the fertilizer. If I asked for a black farmer, it gave a silhouette.
[00:02:56] David: And this is sort of, you know, racial discrimination baked in, unfortunately, to these AI models. And these aren’t any old AI models. These are the world leading AI models. And this is a really, really big problem. I want to take this just in another context, but it’s the same basic problem.
In the same region, we do work in Niger, Burkina Faso and Mali. This is a region which has come to prominence recently for a lot of the wrong reasons, but it’s a really challenging part of the world and one which often gets left out.
So another image issue is, there are all sorts of wonderful apps now around helping you identify pests and diseases on plants. And these exist and they’re great and they work fantastically in context on which images they’ve been trained. But if you’re in the Sahel, in Niger, Burkina Faso and Mali, nobody has the image bank on which to train the images so that their diseases and their pests can be identified so that they can benefit from these fantastic technologies.
It’s such a simple thing. What images are you training your models on? In your case, you’re looking at generative AI to generate images, but I’m looking at also contexts where you’re wanting to actually analyse images, and in both cases, it’s the heart of the training data set, which is so important.
And I can’t stress enough how impossible I feel it is within our current situations to avoid these biases. And I just do not know how we could remove the biases with the technologies in the way they are at the moment, to the extent where you could actually get the results you’re looking for and where you could have people in the regions like Niger, Burkina Faso, Mali, where they are not disadvantaged because they can’t access products that work as well because the training images don’t exist.
[00:05:10] Lily: To add to that, I found this kind of exercise as it were, as quite an eye opener to see how the AI is working and to see those biases in there. As a little game, you know, tell the robots to generate for you a maid or maybe not a maid cause that is inherently going to be a certain gender.
But if you tell it to generate for you a cleaner you see immediately these different biases, these gender biases and things, tell it to generate again and again and again, and have a look at like, okay, of those images generated, how many were men? How many were women? How many? You know, what was the cleaner holding when it was a man and when it was a woman? It tends to go, okay, a window washer. I’ll give you that cleaner, and it will give you one gender instead of another. And I’m saying this here as if there’s only these two options of genders as well. We don’t even, know how AI handles…
[00:06:04] David: Complexity. But this is the point, it doesn’t. The whole point of these sorts of systems is, it’s in some sense giving you something on average. And as soon as you take averages, it’s not actually representative of anything or anyone. My favourite example of this was, of course, they made a perfect seat for an aeroplane for a pilot where they used all the average measurements. And they tailor made the seat for the average measurements of pilots. And of course that meant that the seat fit nobody. Because there were so many dimensions. Across all the dimensions, there is no one who is average on every dimension. And so, by making something which is average across many dimensions, you make something which therefore suits no one.
[00:06:59] Lily: I hadn’t heard that story and I love that. That is, that is great. But then, that also makes me want to ask, you said across all pilots, but what population of pilots? Was it all pilots in the world or was it pilots in the West?
[00:07:13] David: My understanding is that this was a specific country doing this for their air force. That was my understanding.
[00:07:20] Lily: Sure.
[00:07:20] David: But I don’t know this and I don’t want to dig into that and I don’t want to get stuck into that detail because in some sense the point, which is so much more important, is we’re kind of accepting this convergence to the mean, getting this average because we are relying on AI.
AI is, in some sense, dragging us back to the average. Whereas we’ve, for many years, until recently, a lot of what society has been moving towards is accepting diversity, encouraging diversity, embracing diversity. And now AI suddenly is coming in and saying, whoop, nope, we don’t want diversity, this is what I’m giving you.
[00:08:03] Lily: One thing I wonder is about the future and how we go from here as we continue to use tools like AI. Are we going to start to accidentally, or however, not be able to progress or become thinking more in that average mindset rather than outside of it?
[00:08:25] David: I honestly don’t know. And this is something where there are views and visions for the future which I find truly terrifying. And I’m an eternal optimist, I kind of believe that despite some of the current general tendencies not going in directions that I’m particularly happy with, that society as a whole, it’s gone a long way towards accepting diversity, to embracing diversity, to recognising that, you know, we don’t want everyone to be the same.
And so I believe that that belief in our societies is strong enough that we will eventually build tools that support that, rather than fighting it. And so my hope is that we will eventually build tools that support that. But my worry is that that’s not in the interest of big companies who are driven primarily by profit.
That’s in the interest of governments, of international agencies and so on. And so part of this might be a power dynamic battle about the pressures of who is driving the development of the technologies. And if it is only for profit companies, well I’m afraid for them, of course. It’s very cost efficient to just ignore the margins and focus on the main market.
You can get more than enough money from that. Most of your money is created by just having things which are good for many people. And so there is a concern around that. And this is something we’re of course deeply involved in ourselves because we end up building a lot of tech for the margins. That’s where we tend to be working. And we’re working with universities, with UN agencies, with charitable foundations, who are the ones supporting that work. But, as you can imagine, that the money in that is not competitive with the money in just selling things to the majority.
And so we’re not a dominant force in this at all. The dominant forces building tech are doing so for big markets. That’s how you build good big tech. These are challenging issues. And of course, AI is right at the heart of this right now. And it’s not AI itself, AI can serve either purpose. It depends how you implement it.
[00:11:03] Lily: Yeah, because we’ve spoken before, you gave a story before about I want to say it was Harvard University, but I might be completely wrong. On a university and generating new ideas or having a competition with new ideas and AI was kind of eight of those top 10 or something. Eight of those top 10 were AI generated. And, that shows that AI can be creative, you know, it doesn’t have to fit with this average and with everything we’ve done.
[00:11:30] David: This is a really interesting point. The question of whether AI is itself creative or whether AI can be used to enhance creativity. I would certainly agree with the latter. And there’s evidence which is emerging for that. The question of whether AI itself can be creative is really a question on the definition of what is creative, what does creative actually mean. And that I don’t really want to enter into that philosophical space, because it’s great for discussion, but it isn’t something which I feel qualified. We spent enough time with philosophers who are thinking about these things really hard and really well to recognize that our thoughts on this are maybe informed, but they’re not fully formed.
[00:12:19] Lily: Yeah, sure. So I just want to link that back with the average bit though. So to me, and this might be where I might have drawn the wrong connection here, but to me, we’re talking about things being average, and AI giving the average. And to me, that if we kind of give the average, we’re giving things as they are. And so how can we kind of create, use that to create?
[00:12:41] David: So I would frame that slightly differently. It’s about AI and its role related to diversity. Is AI supporting diversity or is AI suppressing diversity? And I would argue that AI is totally neutral. It is how you implement AI which determines whether you support or whether you suppress diversity.
And so the question shouldn’t be, what does AI do for diversity? The question is, what does certain implementations of AI do for diversity? And you started this with this wonderful example where in the context of you trying to use AI tools to generate images, you have found that those tools have suppressed diversity.
And you were looking for specific elements, because you had a specific context, and you were struggling to meet those needs. That brought up the more serious issue. If you didn’t really care about the context, then, well, you’d just have taken the first images that came along. And therefore, everybody would do that, and that would reduce the diversity, and this would therefore take us back many years as a society. And so, as a society embracing AI tools, if the AI tools are naturally suppressing diversity, then that’s a really worrying sign.
Now, building an AI tool differently so that diversity is baked in, and that you actually get elements of this where some elements of diversity are recognized and you always have the option of either choosing or adapting, it bakes in ideas of diversity.
It’s relatively easily programmatically to imagine how that could be done. It is not, as I understand it, the pressures that the AI companies are facing at this point in time. Maybe they will have to face that if the lawsuits start coming in. Now, generally, I’m not a big fan of going through legal systems, but I would absolutely encourage groups who support ethnic diversity, gender equality, and so on, to take big tech to task over the lack of diversity and what the outcomes of their models are doing.
Because the argument that it is not me, it’s the algorithm, is totally false. It is an implementation choice. Whether the big tech recognises that or not, it is. You can build these solutions to encourage, to embrace, to support diversity if you put the time, the effort, and of course, from their perspective, the money in.
And so the choice not to do so, is a choice about getting the product out faster, being first to market, and therefore getting the money coming in. Spending that money to then actually make the tools better, I would argue that should be their legal responsibility. And therefore, I would not be surprised if in the next 10 years, there were a whole host of lawsuits which came out.
And where, actually, I would hope that the arguments would be made correctly that, yes, these were implementation choices that were made where the developers, and therefore the companies developing them, should be held responsible for suppressing diversity, if that’s what it is actually doing. And it’s not as if this is new, you can go back tens of years, at least, maybe more, to find instances of AI algorithms, which had problems with ethnic diversity. Talk about facial recognition, talk about, you know, there’s so many instances of this, where you have these gender and these ethnic issues in AI algorithms and where a lot of work has happened to be able to state how you could find solutions to this.
Now, I would argue quite simply that holding the algorithms in their first iteration responsible is unreasonable.
[00:17:18] Lily: Sure.
[00:17:18] David: By the time you get to chat GPT 4, is that, what was it, 5, we’re currently on?
[00:17:23] Lily: Chat GPT 4 is where I’m generating the images from, yes.
[00:17:26] David: So by the time you’ve got to chat GPT 4, well, I mean, now the money’s coming in, is it really responsible now? Have they done what they need to do to make this work? My guess is no. Now I would argue they could and they should possibly be held to account. I don’t know, maybe it’s coming out in chat GPT 5 or 6, I don’t know. Maybe they are putting that work in now and maybe if the argument is that they are doing the work but it’s hard, that I believe, then they could be held to account to a lesser extent because they are doing that. But if they are not putting that work in, I would argue that it is essential that our legal systems uphold the rights of diverse communities, the inclusion in society and, and take them to task.
If I had to guess, it’s going to be in the EU where this is going to happen. But these are big deals and I don’t see any real incentives other than the fact that there can and there should be lawsuits which hold them to account because their market incentives aren’t there. And therefore the only other structures we have in place in our society are legal structures where, you know, inclusion is enshrined into most laws.
[00:18:51] Lily: So this is something now though about what the companies can do and their responsibility here, but then what can we do? So when I’m generating images, I knew, okay, I know the context I want, so I kind of had to fight hard for it to give me that context.
Sometimes if I wanted to generate a scientist, it would give a man by default. I didn’t actually notice that, funnily enough. I should have noticed that, but I didn’t really click that it kept giving me a certain gender until I was talking to Danny about it, who’s also a director of IDEMS. And he asked me, because I was talking to him about this more ethnic diversity side, and he said, okay, and what about gender?
I was like, you’re right. It’s also not generating female scientists as often as male scientists. Obviously, if I ask ChapGPT, okay, generate me a female scientist, it will give me one. But that’s not the solution.
[00:19:42] David: Well, it is. I mean, it is the intermediary solution.
[00:19:46] Lily: Sure.
[00:19:46] David: It’s not a long term solution, it’s not a societal solution. But I’m afraid we can’t be the ones to drive lawsuits against this because they’re not giving people scientists, and I, you know, this is not our role in society, I’m afraid. I’m afraid there are other groups who are much better qualified and set up to do that.
We need to just be aware of this. We need to be making sure that when we use these tools, we use them in ways which are ethically responsible and we notice, we pay attention to the details. So just noticing alone is the first step.
[00:20:26] Lily: And some of the others that use these tools to do this, we would kind of recommend that noticing and making sure that you may have to add specifics for what you want in your image.
[00:20:37] David: I’m going to get more concrete in this and give you some work. I haven’t done this on a podcast before, but, you know, I think you should create a simple document, which is what you use to try and make sure that you carefully avoid these biases when you’re using these tools at the moment in their current shape. And then the key point is, if that’s a small, relatively simple document, what I would do is then when we talk to others about how they should use this, because we have our partners who are using these tools all the time, we should then make sure that they have or they use a similar document. It can be an open resource, which they can then take and they can adapt and they can use themselves. That is important. This is essential that we take this and we bring this into our working practices.
And this isn’t something which existed. I’m sure some already exist and are out there. We’re not going to be the first people to be doing this. But I do think that there is value from the perspectives where we’re coming from of us actually providing such a document and not just relying on the document of others. Because the thing that we have, which enables you to look at this in, I think, a way which is maybe different to many others, is this idea of actually understanding the algorithms that are behind it, maybe adapting it as the new versions come out, understanding how those algorithms have changed, what they’ve done related to diversity, actually dig into that. As the technology is going to change, and it’s going to change, and my hope is, I’m an optimist, my hope is that these will get better.
This is, it’s in its infancy, the generative AI is still very, very young. So it will change and it will improve over the next few years. How it changes is going to be something which will be influenced by social pressure, whatever that means. And so getting people to actually fight, good fight for that, to make sure that these change in ways which are aligned with societal beliefs for diversity, equity, inclusion, that sort of thing, is important.
We are not best placed to do that, but what we are well placed to do is to actually be the interface between people looking to serve that and people who understand the technologies deeply. And that’s where we could contribute. So, find a place we can contribute, and contribute. That tends to be my approach to these things in general.
[00:23:17] Lily: Nope. That’s true, that’s true. And I shouldn’t be surprised that I’ve been given work from this!
[00:23:22] David: [Laughs] Yes.
[00:23:24] Lily: But no, it sounds like interesting work and not a difficult document to make either that other people can kind of add to from their experiences. I know, certainly after the incident of my 40 requests to get an image of changing the colour of a hand, I literally, I put that into different slides on a PowerPoint just to kind of have that there for myself of this is hilarious, of quite an interesting example of…
[00:23:51] David: I mean, it’s the sort of thing that, we’re academics as well, we were academics before. This is the sort of thing we’re actually writing this up properly, actually maybe even publishing this, actually once you’ve got that document which actually goes through and describes this, actually saying this is what happened, this is what led to the document, giving that as a presentation at the conference. I think you could do this, there’s a number of conferences which are easy to attend and sensible where I think you’d find traction in getting support to then build up these processes.
It’s a problem which everybody’s aware of.
[00:24:26] Lily: Hopefully.
[00:24:28] David: Well, no you’re right.
[00:24:28] Lily: Because if you’re not aware of it then you might not be…
[00:24:32] David: You’re absolutely right. I really got that one wrong. Everyone is not, I wish everyone was aware of it. Lots of people are aware of it. But what proportion of people that is, is actually quite scary. My guess is that it’s much lower than I hoped. We are not the only people to be aware of this and thinking about this. That’s sort of what I should have said. There’s lots of other people who are trying to deal with these issues, tackling them, but there are so many people who are maybe not aware and not thinking about this, that this is exactly a concern that we need to have and we need to bring.
[00:25:15] Lily: And unfortunately for me, the only problem here with these kind of images coming out this way. For me, the only problem is that, okay, I have to change it a little bit. I have to be aware of it. I have to make sure I give the correct prompts to kind of allow for this diversity in the images that come out. But actually going back to the start of this podcast, when you were talking about the farmers, that’s where the kind of real problems come in.
[00:25:40] David: Absolutely. I mean, you’re absolutely spot on. There is no automation in what you’re doing. And there’s a human in the loop who is able to actually take responsibility for the end result. And in that context, I have full confidence in you for making good ethical decisions. And if you get it wrong, which you will occasionally, then other people can pick you up on it and can challenge you. You know, you’re in a team which will. So it’s something where this is likely to come back and, you know, yes, I didn’t think of that.
But in the context where things just get automated, it’s scary. In the context where this then affects people’s lives and there’s nothing they can do, they’re powerless with it. This is really, really difficult. And so it’s a problem and it’s a problem which is solvable. That’s one of the things which I think we’ve got to remember, but we need to put in place structures to be able to solve these problems, and it’s not going to happen on its own. This is where this is going to be huge efforts by large numbers of people to actually build the societal structures which enable these problems to be solved.
It is not a given that these problems will be solved in the future. We are at a fork, if you want, into what the possible futures could be, where some of these issues get resolved sensibly and we build good technology which supports diversity, equity, inclusion, or we build technology which suppresses it.
I think that’s probably a good place to end.
[00:27:19] Lily: Yeah, yeah. Well, thank you very much, David. It’s been a very interesting conversation, as always.
[00:27:26] David: Thank you for bringing it to the table.
[00:27:28] Lily: No, and thank you for the work. I’ll go get on with that. Thank you.