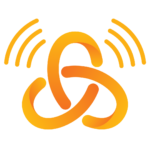
Description
David Stern and Dr Lily Clements dig deeper into the Dutch Childcare AI scandal, its causes and consequences. They analyse how this could have been avoided or mitigated and highlight the need for more work on the responsible use of AI, and particularly the importance of the human element in integrating AI to help in societal issues.
[00:00:00] Lily: Hello, and welcome to the IDEMS podcast. I’m Lily Clements, an Impact Activation Fellow, and I’m here with David Stern, a founding director of IDEMS. Hi, David.
[00:00:15] David: Hi, Lily. What’s the plan for today?
[00:00:17] Lily: So today I guess I wanted to continue on this Responsible AI series and maybe dig into some case studies of where things have gone wrong. I mean, I call them case studies, but just examples which we’ve come across, and to discuss what could they have done instead.
So one that springs to mind is the Dutch child care scandal. This was a situation where in the Netherlands they used an algorithm to determine, not detect, if someone is using childcare benefits fraudulently.
The result of this, and there’s some interesting articles on it as well, was that there were children taken away and put into care, a lot of financial problems for different families suicides, divorces… there’s horrific consequences out there.
[00:01:05] David: And this was the most shocking. We owe a debt of gratitude to our philosopher friends for introducing us to this, but I’m forever scarred by this particular case study. It is horrific and it’s something I carry with me. I don’t believe there was any ill intent.
[00:01:26] Lily: No.
[00:01:26] David: But the dangers of using our skills incorrectly or irresponsibly, are just phenomenal. As a society, we need to be afraid of this. And this is not an isolated incident. I mean, it’s in the news recently about the UK integrating these things into policing, into all sorts of other things.
[00:01:53] Lily: Yeah, into helping with going through people’s immigration documents and things like that.
[00:01:57] David: Exactly. And it blows my mind and it really frightens me that actually this particular case study demonstrates the problem is almost insurmountable at this point in time. We do not have the tools to ensure that we avoid these scandals in the future yet. The regulation that will come in may help, but it will not resolve this.
[00:02:35] Lily: Sure.
[00:02:35] David: So I’m genuinely afraid about this, but it’s important to also break it down to the things which were avoidable, and to understand little bits of why this is so serious.
[00:02:50] Lily: Yeah. Well, and so I guess that’s why, I said determine instead of detect and my understanding from other conversations with you, David, is that it should have more been highlighting this person’s at risk of this, this person’s at risk of fraudulently using child care benefits or claiming child care benefits. But this doesn’t mean that this person is doing it.
[00:03:13] David: Absolutely. So let’s mention very quickly two elements of this particular example that we’ve been able to understand from the literature that’s out there. Where I believe some problems could have been avoided. So I’m being slightly careful with my language here because it’s such a serious issue. The interpretation of the use of the algorithm can only ever be an estimation of risk. The nature of the data which is fed in, which is used to identify this, this is something which could be used to raise red flags. And it might not be an element in the question of what is the risk, is the risk of fraud, or is the risk of the situation those people are in. There’s different elements of risks. And so do you go in with somebody who’s looking to check or to police them on whether they are trying to commit fraud? Or do you go in with a care worker who’s looking to help and support them because they’re in a difficult situation?
There’s a human difference in terms of that, what risk you’re identifying? In this particular case, I don’t have those answers at all, and I don’t know enough about it to be able to offer any advice on that. But what I do know is, from what we understood, was that this was not interpreted as risk. And that is, in my mind, a cardinal sin.
If you’re using these sorts of algorithms, you’re using these sorts of AI based systems, you have to know what they can and what they can’t do. They cannot, and they will not be able to understand what the current situation is. They can predict the current situation based on the data they have from the past, based on any other information that you feed in, but they can’t know it.
So they can offer an estimation of risk. They can raise a red flag. But they can’t know. There’s a sort of an element of humanity to this. The best illustration of this is if you were to use a similar algorithm to predict crime, you would be able to identify people who are at risk of committing crime, and therefore you might want to observe them, but it would be unethical to put them in jail because they might not have committed a crime. You can’t put somebody in jail because they might commit a crime.
[00:05:51] Lily: You say that but then we have the US case study of Compass, which is where they used an algorithm to determine people that have been arrested, if they’re at risk of re-offending, to determine their bail and things. I’d say a more kind of appropriate one is more in the healthcare or…
[00:06:09] David: Well, no, but that example’s different. Let me be really clear, so let me come on to that Compass example, because it’s another one, but the key point is that is a re-offending. There is again a question about whether you can or you should use it for that, and that’s a whole different question. And I won’t dig into that now, that might be another podcast, but the key point is that by the time you’re looking at re offending, they’ve already committed a crime.
So that’s not the same point. The point is, you have a totally innocent person, who because of their demographic, because of whatever it is that you have a data about them, you feel is at risk that they could commit a crime. Well, they may even have in their mind an intention that I might commit a crime. Even if you could read their mind and they’re thinking about committing a crime, you still can’t jail them for that. You can’t jail them until they’ve committed the crime. Your thoughts are your own. So even if there is a choice, you know, this is important in ethics, that human choice, they could go right up to that point where they’re about to commit the crime and decide no, I’m not going to make that. And if they don’t commit the crime, then they haven’t committed the crime.
[00:07:21] Lily: Sure.
[00:07:22] David: Because they have that choice up to that point. So if you think of that in that context, there is no prediction you can do if you believe that there is an element of free will and of choice. Until something’s happened.
[00:07:36] Lily: Even then, I’m sorry to go back to it, but even in the Compass example, just because someone’s committed one crime doesn’t mean they’re going to commit another.
[00:07:45] David: Well, but the question is how lenient should you be with them? How much of a danger do they place on society? So then at that point you’re deciding, do they serve their full sentence or is it responsible to release them early?
[00:08:00] Lily: Okay.
[00:08:02] David: Yeah? So if that’s the decision you’re making, that’s different. I think we can dig into the compass example, but it is a different example, which I think is important. And I think one of the things which is really important if we come back now to the specific case of this Dutch child care.
[00:08:23] Lily: Yes, yeah.
[00:08:24] David: That confusion of risk with reality, that is very simple. That’s something we can teach people not to do. And that’s something which has to happen. More than that, we have to put regulations in place so it is illegal to confuse them. That’s very simple. That will come in in these regulations. It’s not me thinking this, there’s a number of other people in the ethics world, in the data science world, in a number of different worlds who understand this. I am pretty confident that will make its way into regulation. But that wasn’t the only problem. The other problem we were able to identify, and this relates as well to the Compass example, is that there were biases in the data.
[00:09:05] Lily: Yes.
[00:09:06] David: Because of the data and the nature of the data that was used, there were actually particular populations where this risk was misidentifying people in ways which it shouldn’t. Again, we could have another podcast about this around gender or about race because there are some very important examples which dig into these issues around biases.
But in this particular case, there is a question about the fact that actually could or should the algorithm have been built better to avoid some of those biases. So even if you changed the interpretation from actually thinking that fraud was happening to them being an investigation because that there was a potential for fraud. So even if you did make that change, there would still have been biases against certain minority groups who would have been overchecked on this. So you need to be able to either correct the algorithms, and there are ways to do that and it’s hard, it’s hard enough that Amazon gave up when they had inbuilt gender biases into their recruitment algorithms. It can be a really hard problem to remove biases. But there are ways to improve the algorithms to do that. But there’s also ways to build systems so that actually these racial biases or these minorities who might suffer in that way don’t lead to negative outcomes.
So this is now, let’s say, what’s your response? Do you send in an investigator or do you send in social care?
[00:11:01] Lily: Sure.
[00:11:02] David: If you send in an investigator, now the people who are being over investigated, that’s, going to lead to problems. If you’re sending in a good, skilled social care person, who is actually there first and foremost to support, then maybe you’re giving more support to a minority group than you would have done otherwise. But that’s not a bad thing. So you’ve got to also think through how your interpretation and how the biases, the potential biases in your algorithms play out. Is it acceptable to over investigate certain ethnic minority groups?
We know this from the stop and search issues around policing. This is not acceptable to have racial biasing or any other biasing within policing. Whereas, is it acceptable to offer additional support to minority groups because your algorithms may be biased towards them? Well, maybe that is acceptable. The cost to society is small because it’s a minority group. So actually, this does have a cost to society, but it’s a small cost to society. So maybe that small minority group is now, because of the biases in the system, getting more support than they maybe should.
But maybe the biases in the system are because historically they’ve had less support than they maybe should. And so, maybe this is a way, if we use this well, that this leads us to a fairer, better society, if we understand how to build responsible systems.
[00:12:46] Lily: Yeah.
[00:12:47] David: I don’t have the answers on this at all, but I do know that there’s deep thought needed on individual cases as we’re going through these. There’s no simple answers, it’s not as black and white as using AI algorithms in the Compass example. Very good instance there. It is not as black and white as working on that is bad. No, it is about understanding how if you’re going to work on that, how do you do it responsibly so that these systems are built to serve society?
And that’s the hard part. It’s really hard to do so. Almost certainly, there will be elements of getting it wrong before you get it right, but being able to actually have the intuitive learning processes in place to catch these issues, to be able to be responsible to avoid the serious negative outcomes that we heard from the Dutch example. To be doing so cautiously, to be integrating them. And you can’t integrate them slowly, this is part of the problem with the way our society is changing.
[00:14:04] Lily: Well, yes. But if we go back to that initial demystifying data science.
[00:14:10] David: Yes.
[00:14:11] Lily: And you said with the post office example, it was initially 90 percent of the time, right? But what was the consequence? The consequence was that oh my post went to the wrong person so I might have to wait a few extra days to get my post because they have to send it back. Or I might have to ask for my post again. Can be problematic in some situations. But the problem there is my post. Now we’re talking about the problem being people getting their children taken away if it goes wrong. So I feel like we don’t have time to have that teething phase while we get things right.
[00:14:46] David: Absolutely. So let’s take the post office example and say, okay, imagine that it was life or death to get the post to the right place.
What do you do? Well, you don’t take away the original systems. You leave the original systems in place first, and you put the sorting algorithm, which gets you right 90 percent of the time, on the back of it. So there’s an additional expense.
[00:15:09] Lily: So you have 2?
[00:15:10] David: For a big period of time, you have your two layers, you’re not replacing your other component, which in that case would have been manual hand sorting. Now the manual hand sorting would be more efficient because 90 percent of the time their job would be done. They’d stand there and say, okay this is all looking good, oh that one’s in the wrong one, and so on.
[00:15:28] Lily: I see.
[00:15:28] David: So you’re actually making your manual job more efficient, by doing both. Now of course you’re not yet building the efficiency from the system and there’s been some really interesting studies I think recently where I think they had this thing about sort of checking and if you were checking another human, humans checked more carefully than if they were checking an AI. But they did this experiment recently, I believe. I don’t know those results well, so I just heard about this. And I need to look up that research to understand, do I actually believe that’s what’s happening? But I know, I can understand that there are issues about how you fit AI into a system will affect the system.
But I do believe you can do this in stages, where you don’t get rid of the human component, they work alongside, or you gradually reduce it, and you’re never wanting to get rid of it. Even in the post office example, you’re never going to get rid of the humans, there are still postmen. And when there are postmen, and they’re replaced by drones, because you can do that automatically, at the end of it you’d still have a human receiving the letter.
Now, okay, once you actually get robots receiving the letters and reading them for you, that’s a whole different story, but probably consuming the information at some point there’s going to be a human in that loop.
[00:16:46] Lily: Yeah.
[00:16:46] David: And that’s what we need to be always thinking about. Where are the humans in the loop. To be responsible, that’s critical.
[00:16:54] Lily: That’s a very good point. Thank you. And I think that that’s probably a very good place to end it on.
[00:17:03] David: I think almost. I guess the one thing I do want to come back to the Dutch child care example. This will be for me, a haunting example for the rest of my life. It will ground me, it will keep me humble in the sense that we as data scientists, as people with the skills to build these systems, we are affecting lives in ways that we may not understand at the time. And this is something where there are two sides to that. There’s one, and I think this comes to this sort of mantra, do no harm. You know, there’s, do no harm purposefully. So, do not deliberately do harm. And that I feel I could do, and I think in that particular case, I would have been able to discuss and think about how the AI system would have been integrated into other systems to ensure that actually it was done responsibly.
That’s the sort of discussions where, in terms of the do no harm purposefully, I’d have been able to say, look, if you’re going to use it like that, then this is the wrong, I don’t want to be involved in that. I would have said, I don’t want to be involved in that job because it’s the wrong thing to do.
So actually purposefully doing no harm is something which is within my control. But what scares me about this case is that that’s not enough. That actually, even if we’re trying to do good, even if we’re trying to do things right, even if we’re trying to do things to the best of our abilities, even if we do things really well, there are elements of the consequences which are beyond our control as people who are building these systems.
And to me, that’s what scares me in a sense. I love being a pure mathematician because I didn’t have to engage with the consequences of my work. They weren’t going to happen for hundreds of years and actually this is an element of where we’re on the front line. Our work, our efforts, have consequences. I’ve always had utmost respect for doctors who hold people’s lives in their hands and have to be responsible for others in that way. And so much of society, that’s so important.
We are getting to that place where how we use these AI systems for societal function, which is the case we’re talking about in the Netherlands, has direct consequences. And so actually, thinking through how to train people so that they can be responsible in that, that’s I think, so important now. It’s not part of data science training. There’s a little bit on ethics here and there, which is coming into a lot of programs, but the actual skills to be able to think about this, not just in what you’re developing, but in how things are used over time, how you’re monitoring it, when it goes into use. The role of the data science through the life cycle of the AI that they’re involved in.
Now, I’ve not heard those discussions happening, I’ve not heard of training happening in that, but it’s so central to building responsible AI systems. And if I take that particular example, this wasn’t a difficult task for data scientists, it’s getting easier and easier. So you get a recent graduate who’s just come out with a few skills and who can build a system, who gets now employed and put something together and they’re really proud they’ve done what they needed to do. They’ve helped the department to do so much better and to be so much more efficient. Aren’t they helping society? And look at the consequences.
[00:21:11] Lily: Yeah.
[00:21:12] David: This is where we need that training at so many different levels because there was no ill intent in my understanding and yet this is going to happen more and more and getting people to have the skills to avoid it is hard. And it’s not about the data scientists on their own, it’s about their managers, it’s about the people collaborating, defining the tasks, using the systems.
We need people to be AI literates. They need to understand what AI systems can and can’t do for them, and therefore to interact with them in the right way. Even if you can’t build the system for yourself. Understanding what AI systems can do is going to be so important. Thinking about using AI responsibly, this is a skill we need at a population level, and I don’t see how that’s going to happen.
In this moment, this is on the top of my mind, and I don’t see this being addressed. That’s one of the things I’m worried about. I’m sure we’ll have other podcasts where we dig into this, but this example will haunt me, because we haven’t put structures in place which will avoid this in the future. This is not the last scandal like this we will see.
Sorry to finish with a bit of a down note.
[00:22:39] Lily: Well, that’s okay. It’s about responsible AI and sometimes we need to remember the kind of consequences that can happen until we get, and even when we have systems in place, implementing the systems.
[00:22:58] David: Absolutely. And maybe just to that point, I do not believe we have the skills or the tools yet to be able to avoid these problems in the future. There is so much need for work on that. There is knowledge, there are people who are working in these areas who are working towards this, but not enough and certainly not at a scale which is needed to lead us to do this, given the speed at which these systems are being built and integrated into society. Okay.
[00:23:37] Lily: Thank you very much.
[00:23:38] David: Thank you.