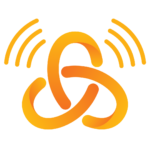
Description
As society embraces AI, interpreting its results can be a matter of life and death. Lily and David consider how we can be misled by data in general, including the results of AI models. They discuss how misinterpreting the output of data often comes down to misunderstanding the limits of what data can tell you.
References:
Simpson’s Paradox: https://en.wikipedia.org/wiki/Simpson%27s_paradox
How to lie with Statistics by Daryl Huff
[00:00:00] Lily: Hello and welcome to the IDEMS Responsible AI podcast, our special series of the IDEMS podcast. I’m Lily Clements, an Impact Activation Fellow, and I’m here with David Stern, a founding director of IDEMS. Hi, David.
[00:00:19] David: Hi, Lily. What are we discussing today?
[00:00:22] Lily: I thought today we could discuss How data lies.
[00:00:24] David: Oh yes. Go on. I like this one.
[00:00:27] Lily: Well, I suppose we’ve got these different case studies that we’ve looked at and through that have come across instances where people have been misled and the AI algorithms that the output from the algorithms that have come out have said something completely different to what is I guess, acceptable.
[00:00:53] David: It’s not only what is acceptable, it’s what is actually happening. And the point is that this is very common when trying to analyse data. Analysing data is really hard. And this is where there’s lots of… You know, one of my favourites of course is the Simpsons Paradox. Where, if you look at the data without taking into account a categorization, you actually could get the opposite result, in terms of a trend, from actually when you take into account a categorization.
[00:01:23] Lily: Yes, I think Simpson’s Paradox is one which… It’s seeing this visualisation.
[00:01:29] David: It doesn’t matter how I talk about it, without seeing it, it’s hard, but it’s beautiful. Wikipedia has nice visualisations of it, it’s all over, everybody talks about this, this is not, this has nothing to do with us, but it’s sort of really common.
I do have a really concrete example I love to give.
[00:01:46] Lily: Sure.
[00:01:47] David: Which explains how this actually can lead to the wrong conclusions. So, for example, if you did a study, which was done over a number of years, of basically digital literacy, and you plotted essentially, digital literacy by age, you tend to find that digital literacy decreases with age.
[00:02:16] Lily: Yes, absolutely. I can expect that because…
[00:02:18] David: Yeah, a lot of young people are very good with digital literacy. Depends how you measure it and so on. Depends at what point. But there’s a lot of studies have happened which have done that. And so, as you get older you are obviously less and less digitally literate.
[00:02:34] Lily: Sure. I mean, I’m waiting…
[00:02:38] David: But you’ve fallen into the trap, of course not.
[00:02:39] Lily: Well, no, no, no. I’m behind the trap.
[00:02:43] David: The point is if you were to now categorise people by age, and of course you now have a time series, and you see how people actually in those categories, what their digital literacy happens over time, well at all age groups, your digital literacy increases over time. So as you get older, you are more and more digitally literate. You’re just digitally literate at a lower level than people who are younger than you.
[00:03:09] Lily: Why? So you’re saying for age categories, let’s say we take 15 to 25 year olds, 26 to 35 year olds. That’s terrible.
[00:03:20] David: Doesn’t matter. Doesn’t matter how you categorise them. But if you just look at them, you know, all together, you’ll see the slope will be decreasing. As people get older, they are, they do less well on whatever digital literacy measure you have.
[00:03:37] Lily: Okay, sure. Digital literacy decreases. Okay.
[00:03:40] David: Older people tend to be less digitally literate than younger people, depending on how you measure digital literacy. However, if you take within any given age category, how individuals change over time, they all increase. On average, they get more and more digitally literate over time. It’s just that people who are older are starting at a lower level of digital literacy with that particular technology, whereas people who are younger are starting with a higher level of digital literacy. But as individuals, we all tend to increase and become more digitally literate over time.
Now this is really important because this actually says something very significant in terms of society, in terms of actually what you could do, we have people we met recently who do some fantastic work in the north of Scotland, I think it’s Red Chair Highlands or something, and they’re actually doing digital literacy training and bringing devices to elderly people in particular or vulnerable people in general in remote areas of the Highlands. And what’s so important is that actually this has become urgent because so much of society has gone digital that they are now being excluded. They can’t park. They can’t drive into town and park anymore because you need to be able to use a device to be able to pay for the parking.
[00:05:09] Lily: I mean, I find that frustrating.
[00:05:13] David: This is the sort of thing which is happening where that level of digital literacy which is needed to function in society is increasing and there are certain things which are required. If you take the first analysis, which is just, older people tend to be less digitally literate and you misinterpret it to say that as people get older they become less digitally literate, then the conclusion you reach is wrong. There’s no point training people because they get less digitally literate over time.
[00:05:45] Lily: I see, okay.
[00:05:47] David: But that’s not true. The truth is if, within an age category, you look at how people change in digital literacy over time, they increase in digital literacy over time. Therefore, trainings in digital literacy for the elderly and for all categories in different ways are sensible and useful and add value.
Just because young people pick it up much faster doesn’t mean you shouldn’t be training more elderly sections of society to engage. On the contrary, what the Red Chair Highland’s are doing, I’m picking this as an example because I, I heard about their work and I love it, what they’re doing is important. This is really valuable.
But if you misinterpret the data and you don’t include that categorization, you come to the wrong interpretation. The interpretation that as you age, you get less and less digitally literate, which is false. And that’s what we talk about, about how data lies. You can arrive at a false conclusion because you have not considered all the factors which are needed in the analysis of your data. This is Simpson’s paradox.
[00:07:03] Lily: So then how does this link through with, I guess, responsible AI? I suppose my understanding would be… we need to interpret the data correctly when it’s fed into the algorithm, otherwise the output of the algorithm will interpret it…
[00:07:18] David: Well, our favourite, no, not favourite, our most scary case study in some sense that we both find, I think, really terrifying is this Dutch case related to child care.
[00:07:32] Lily: Well, no, I was going to say scary so far.
[00:07:37] David: Yeah, there may be worse ones. But just say a little bit about that, just to remind the listeners.
[00:07:42] Lily: Yeah, sure. So my understanding is that this was to do with child benefits in the Netherlands. They were finding a way to detect people that were using childcare benefits fraudulently. And so they created this algorithm, they got the output from the algorithm and the output said, okay, these are the people which are using it fraudulently.
[00:08:03] David: No. It’s a key point.
[00:08:07] Lily: Well… so, these are the people at risk. These are your people at risk. And then the interpretation of that was, these are the people that are.
[00:08:17] David: Exactly. That’s the key point. It’s this point of interpretation. So this is a wonderful example where the AI algorithm could never know who is.
It doesn’t have the information it needs to do that. So the fact that people interpreted it as saying, these are the people who are using it, fraudulently, is a total misunderstanding of what can possibly come out of that data.
[00:08:45] Lily: And then in this instance, it’s not that, okay, you get a letter in the post and it says that you’ve used it fraudulently and you go down to the office and you clear it up.
No, no, no. In this case, what happened was people were fighting it for years and years and there’s stories and articles about divorces, about having children, thousands of children taken away from their parents, about…
[00:09:07] David: Suicides?
[00:09:09] Lily: Suicide. Yeah, absolutely, it’s…
[00:09:10] David: It’s horrific and it’s such a simple element of, actually it’s the same thing that we just discussed before, of misinterpreting the outputs of the data.
There were also problems with the analysis of the data where actually there were elements of bias within this related to racial discrimination in different ways. And those could have been fixed and the mechanisms could have been better. But the point is not making that algorithm better. That is important and I’m not saying that’s not important, but I’m saying the fundamental thing which should never have happened is this misinterpretation of the output.
Not understanding what the output can tell you and what it can’t tell you. If you interpret the output as saying that this person is fraudulent, then you’re misunderstanding the data that’s been fed in and what the data can tell you. This is just saying that there is an analysis of the data, which means that there is an identifiable risk that this person could be fraudulent.
And that’s all the data could ever tell you. And so you have to build your human systems around the correct interpretation of the output, which is coming from any models, be they a machine learning AI model or a very simple statistical model. It doesn’t really matter. And Simpson’s Paradox is really a beautiful illustration of why you have to do that and you have to recognize that just because the data says something, doesn’t mean that that is the absolute truth. It cannot know the truth. It can only tell you what a given analysis of the data is potentially telling you.
And so, let’s say we got a, an algorithm which then came out and said people get less digitally literate over time and actually that was what the algorithm was explaining, was coming out as a conclusion. Then if we’d just accepted that then our policies would be all wrong because it would just be a question of that analytic tool actually being misled by the data. This is Simpson’s paradox. The fact that you can be misled by data.
[00:11:51] Lily: Yeah, and I think that this is often what’s missing with AI or in these big scandals that we see it’s our interpretation at the other end. And if we go back to our demystifying podcast, we talked about those two examples, about the post office and about birdsong. And the thing that worries you with birdsong is that you don’t have something to catch when people are being misled, but with the post office, there is something to catch there.
[00:12:17] David: Exactly. Exactly. This is about understanding how to use tools. So recognizing, if you recognize, and there’s a wonderful, very, very old book about, you know…
[00:12:29] Lily: Literally I’ve got it here, right now.
[00:12:31] David: You have it there.
[00:12:33] Lily: How to lie with Statistics by Daryl Huff. Was it 1956, or is it older?
[00:12:38] David: No, I think it’s a bit younger than that. I think it’s in the 60s, is my memory. Yeah, it’s a sprint chicken, a bear, you know. It’s in the 60s, is that right?
[00:12:49] Lily: Copyright 1954.
[00:12:51] David: 1954! I’m wrong! Wow! It’s sort of almost going to have its 70th anniversary next year.
[00:13:00] Lily: And it’s still relevant. It’s still a book that I’m holding right now that this discussion on how to lie with data… I was like, well, I know what I need, I need my, my book.
[00:13:10] David: Yeah, but it’s not how to lie with data, this is how data lies. And this is the key point, that that book, actually it was very much, at the time, it was just showing how, oh look, you can do these fun things where you can misrepresent data if you do it in the wrong ways, and it was showing this as a warning to help people.
But really, it’s so relevant nowadays because so many people are looking for data driven decision making. I don’t want data driven decision making. I want intelligent decision making, which actually uses data in constructive ways. But it’s not that the data has the answers. This is where we need to learn how to interrogate the data.
And that’s so important. So, I’m really keen that as people embrace, and I want them to embrace AI, I want them to embrace these new tools, but as we embrace them, we have to think about and be very conscious about the limitations of just trusting what comes out. Nine times out of ten, if you get a result coming out of your data, your first reaction can be great, I might understand you or something.
Your second question should be, hmm, how can I challenge my understanding? You know, this is it.
[00:14:36] Lily: Yeah, and, I was going to say, I don’t even know if Simpson’s Paradox is covered in this book. There’s, there’s so many other ways of how data can lie and, and misinterpret, and I think it’s so important to always assume that the output can be interpreted in a different way.
[00:14:56] David: There’s a whole domains that look into this, you know, academic domains. This is what I would argue is the difference between science and pseudoscience. And that’s maybe going to be a whole another podcast at some point. But the whole scientific process is about you never get proof of something as a scientist. This is why I love being a mathematician. You could actually know stuff.
As a scientist the best you can do is you can say with the available information I have, I don’t yet know how this is wrong. That’s essentially what the scientific process gives you. It can give you the fact that you don’t yet know how what you’re describing is wrong. And I think, let’s think about that very constructively with, sort of science that most people are aware of.
Newton, just thinking about how gravity works, how the world works in terms of gravity and so on. As a scientific theory it was fantastic, it was world changing and its implications were massive, but it was of course wrong. But at that period in time, they didn’t know how it was wrong, so it was the best theory available at that point in time. And over time…
[00:16:17] Lily: What was the theory, sorry?
[00:16:18] David: Newton’s gravity, you know, apples fall on your head because of gravity.
[00:16:24] Lily: But gravity is true.
[00:16:26] David: Well, but it doesn’t work as Newton described it if you get very small or if you go very fast, and there are all sorts of these edge cases. Einstein, everything he had came about because he realized that Newtonian mechanics doesn’t hold when you start looking at stars, and you look at light, and you look at these other scenarios. You have to think about space and time together. And all of that came out of Einstein, and Einstein’s theories were fantastic, and where he got to with generalized relativity was amazing.
And Einstein believed that God did not play dice. And his theories all fell down because of quantum mechanics and where actually when you got really, really small, and then the laws changed again, and you needed to think about things differently. And so much more has been learned, but it’s always about understanding. It’s not that Newton was wrong. It’s that Newton’s theories are not universally right. They’re not right in all contexts. And they’re not right when you get very, very small. They’re not right when you go very, very fast, and so on. And so, there’s elements of those edge cases where the scientific process is so powerful.
It’s able to say, to the best of our knowledge, we don’t know yet why this is wrong. We only know in the cases that we’re looking at, it seems to be right for everything. We can’t find why it’s wrong. But that scientific process is what we need to bring to data. You know, whenever you’re looking at data, if you find that, coming back to our simple example, if you have that result, that as people get older, they become less digitally literate. And you, that is your hypothesis because that’s what your data is telling you. But then you interrogate your data in many different ways, and you find that, oh, wait a second, I need to change my hypothesis. My hypothesis now has a double hypothesis. Younger people tend to be more digitally literate than older people, but as you get older, you get more digitally literate. This is a really important progression as you’ve taken that next step. Now those hypotheses, we don’t know if they’re right, but we do know that they are better than the original hypothesis.
So those two distinct hypotheses together explain the data better than the original hypothesis. And that’s the scientific process. It’s not to say, okay, we now have the right answer. Anyone who tells you they’re using science to get the right answer, they’re only really doing pseudoscience. Pseudoscience tries to prove what you know to be true using data or using evidence. No!
Real science is all about trying to find out how, why, what you think is true is actually wrong. And if you can’t find out why it’s wrong, then it’s the best hypothesis you have for now. But maybe in the future you’ll be able to get a better hypothesis.
[00:19:42] Lily: But then if we link to responsible AI again, okay well this is the best answer we have for now, that then doesn’t justify things like the benefit scandal that doesn’t…
[00:19:55] David: No, it doesn’t, because they used it wrong. So the key point is, how do you use AI? When you get a script out of ChatGPT, because you’re wanting to save time on doing an analysis I’ve given you, what do you do?
[00:20:09] Lily: Okay, so I read it, I check it, I amend it, I engage with the output. And I have that human element come in there.
[00:20:20] David: And basically you then take responsibility that what you end up doing is to the best of your knowledge. You’re not just taking the output of ChatGPT and saying this is the best I can do. That would not be responsible. And in general, in AI processes, this is where… I don’t, it’s a whole other podcast to go into.
[00:20:42] Lily: Yeah.
[00:20:43] David: What’s happening with, let’s say, the self driving cars. But, this is another thing where actually this element of yes, we should be able to make the road safer using self driving cars, but at the moment there’s all sorts of reasons why we don’t know how to do that. And some of these are technical, some of these are issues with the algorithms, and some of these are legal and ethical.
We don’t know how to do the ethics of it. And so that’s a whole other podcast. But I’d argue that it really does come back to this same point. The reason we can’t do that is because we know that data lies. Data will lie. As I understand it, to finish on this instance of the self driving cars, one of the first accidents that was understood as to what happened, my understanding is that because of the complicated nature of a bike with things on its handlebars and somebody pushing it, which was unusual and therefore not well recognized by the AI system for whatever reason, that led to one of the very first automated car deaths, self deriving deaths, because the system was not trained, it didn’t know how to interpret that data.
And therefore, the data was lying, it was being interpreted in an incorrect way. So, how do we build systems which are resilient to that, which recognize how data lies and how you will always have to include that because any data you have is going to be imperfect.
There are going to be misrepresentations where we have to train humans and trust humans to then make good decisions based on the best evidence that can come and to use the data they have constructively. So just to finish with the scandal, what should have happened? Well, what should have happened is recognising that someone is at risk of fraudulent behaviour, you probably need to recognise that this is also somebody who is at risk in general.
And so the right step should have been a human to reach out and understand the situation, the context, in a friendly, supportive way, to understand if they could help. First of all, to try and really dig in, to understand that situation. So that, if it was a fraudulent case, that would have been identified.
But if it was somebody who was simply at risk and being misidentified, they would be supported. And so that correct interpretation of the output, and therefore putting in place the human structures, that would then correctly identify and differentiate the fraudulent cases from the cases in need of human support, that would have been a good use of the AI system. Recognizing how data lies.
[00:23:51] Lily: Excellent. I think that that’s probably a great place for us to finish today. But thank you very much. I obviously love data. That’s what I’m trained in. So I found it a very interesting discussion. Thank you very much, David.
[00:24:02] David: No, thank you.