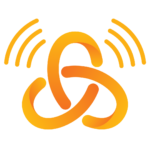
Description
Continuing their ongoing discussions on data, Lily and David consider data literacy, its importance, and the different skills required to interpret and work with data effectively. They explore the challenges of demystifying data science when teaching it to non-specialists. They consider the example of New Zealand’s innovative approach to embedding data literacy in school curriculums.
[00:00:00] Lily: Hello and welcome to the IDEMS podcast. I’m Lily Clements, a Data Scientist, and I’m here with David Stern, a founding director of IDEMS. Hi David.
[00:00:15] David: Hi Lily, great to talk again. What’s our topic today?
[00:00:18] Lily: I thought we could talk about data literacy today, and these kind of different skills. There’s a lot of courses that we work on at IDEMS, as you definitely know. And I thought that we could dig into these courses and, I suppose that there’s a lot of courses out there already on data.
[00:00:37] David: But data literacy is such a hard nut to crack because it’s really ill defined. We work with people who work in statistical literacy, we work with other people who are big data science people, there’s all this stuff about responsible AI. And data literacy really means different things to different people.
And, I think the courses that you’re referring to that we’ve been working on relatively recently, these are for non specialists. So these are getting people who are, maybe postgraduate students, but in other disciplines, and really exposing them to data in different ways, getting them comfortable working with data, understanding data, using data in their work. That’s the sort of course that you’ve been working on and that we recognize there’s still a big gap there.
[00:01:25] Lily: Yeah. Yeah, absolutely. And kind of demystifying data, I know all too often that people see data or see kind of statistics and data as this kind of really scary, difficult thing when actually it doesn’t need to be.
[00:01:41] David: It is challenging for people, but it’s not challenging for people for the reasons they think. And this is actually where presenting it in the right way is so important. Most people think it’s challenging because they don’t like maths, they weren’t good at maths, and they are scared therefore that working with data is very mathematical, it’s very numbers focused.
But actually, it doesn’t need to challenge your mathematical skills to work with data. But what it does do is it really, done well, you realise that what you’re looking at is often much more complicated than you first imagined. And this is what we often find. The reason it’s hard to work with data is because it’s variable. It doesn’t give you nice, clean answers. Mathematics is all clean. It’s black and white. There’s certainty.
[00:02:38] Lily: Yeah.
[00:02:39] David: Whereas what makes data so hard is the variability. That’s the heart of why it’s so hard to work with it.
[00:02:47] Lily: Yeah. But then I suppose, with data can still be a kind of, I guess, wrong way.
[00:02:52] David: Absolutely.
[00:02:54] Lily: And so while it’s not as obvious as it is with maths where it’s yes or no, at most levels, or at some levels that you are in. But there’s this kind of, as you say, this variability to it, but, there’s so many different ways you can interpret something. But that’s not disordinary to like other subjects that people do. I’d say that maths is a unique thing for being right or wrong, but actually in other subjects a lot of it’s down to interpretation.
[00:03:23] David: And you use frameworks to understand, where of course data has a mathematical, if you want, foundation in many ways, is there are certain things where you can tell the difference between things which are demonstrably right or wrong in certain ways. And some of that then relates to the nature of the data you’re looking at.
And one of the things which is really challenging, of course, and which people really struggle with, is that, especially when you’ve got lots of data, there’s lots it can tell you, it’s very rich very often. But that doesn’t mean that all the questions you have on it can be answered.
We had this very recently when we were working with an agricultural researcher where, he wanted to find out something and the interns we had working on it tried to do the analysis and I kept tearing their analysis apart because they were trying to answer a question where the data they had couldn’t answer that question. And so we went back to the researcher and indeed he did want an answer to that question, but he recognized that the fact that the data couldn’t answer that question didn’t mean it wasn’t useful, that it could provide other insights.
And so it could reformulate the question, and most importantly in that particular case, it could enable him to actually get information which could be conveyed back to the people who gave the data, where the data originated from, to ask them questions, to interact with them, to have qualitative follow ups, because he knew there were these rich stories behind the anomalies that we were observing in the data, where the information we had couldn’t possibly capture that.
I’ll give you a, couple of them. There were these anomalies where they were looking at animals which were being fattened up to be sold off.
[00:05:20] Lily: Okay.
[00:05:21] David: And there were some animals that were fattened up a lot, but they made no profit and there were other animals where they didn’t fatten up much at all, but they made really large profit.
And if you just have that data, it really doesn’t make much sense. But if you ask him, he said, oh yeah, I know we talked with some of the farmers, and some of the farmers they sold the animal back to the household because they then wanted to eat it. Of course, there was no money exchange, there was no profit in that sense, but the animal that had now been fattened up was now consumed by the household and sold back to the household.
[00:06:03] Lily: I see.
[00:06:04] David: In another case, oh yes, some of the farmers, they sold it just near this big festival. The prices were sky high.
[00:06:14] Lily: Ah.
[00:06:15] David: And of course, these differences, that information wasn’t in the data, there’s no way to exactly know that in the data that was there. We only had this sort of data on how much weight the animal had gained over a certain period, over a number of days, and the price at which it was bought, and the price at which it was sold, and therefore the price difference.
And so, these real world contexts within which they’re living, understanding that, and when you start telling those stories these things are obvious. This is life. This is exactly what you’d expect from life in whatever environments you’re living in different ways, and so on. The data is not wrong, it’s just you can’t always interpret it without additional information.
And the more data you have, I do this whenever I’m at the supermarket and I don’t have a reward card. I love messing with the data and asking the person in the queue next to me, Do you have a reward card? Oh yes, great. Why don’t you take the rewards for my shopping? And I know that’s going to mess up the data. I wonder why they’re buying these things suddenly.
These anomalies in the data, which can happen for all sorts of reasons, whatever data you’re looking at, however you’re doing it. Actually, if you think about it, once you bring your common sense to bear, there’s a lot that you know is beyond the numbers you have. And so it’s always hard to work with data, but not because it needs to be hard to do the data analytics, but because the data analytics on their own are almost never enough.
[00:08:07] Lily: Yeah. And you need to have that kind of level of context or go back to the, I love data, but I’m not an expert in agriculture or in climate or in anything that you’re faced with in terms of data, you need to have those experts to actually speak to that can provide that context.
[00:08:26] David: Exactly. The example of the supermarket data, which I think is so great, because I don’t know if people realise this, but, people have had reward cards for a long time in the UK, and other parts of the world as well, but the UK, but actually this supermarket data, this was one of the really big areas of advances in terms of data science, going beyond statistics.
[00:08:48] Lily: Ah.
[00:08:49] David: Having these really big data sets and doing data analytics on these big data sets of trends in different ways so you could figure out what you needed to stop, what were people buying and why and where. It’s a fantastic, as somebody who’s interested in data science, this is fantastic data. It’s so exciting in all sorts of different ways. Why do you think you get reductions if you actually give a coupon? Because you’re giving information, you’re giving data, which is really valuable to help supermarkets run more effectively and more efficiently.
It does make me slightly perverse to then love messing up the data. As a data analyst, I’d be really frustrated to have my data messed up, as I like to mess it up. But there is something about the fact that, this has moved forward. There have been a lot of advances in terms of data science because of these sources of data.
It’s not the only one, but it is one which did push the boundaries, traditional statistics didn’t apply. And so data science really was moved forward by the analytics of things like big supermarket data.
[00:09:53] Lily: I was just going to say, and this is my understanding anyway is where that kind of big difference between statistics and data science comes in is if it’s been designed and here it’s data, which there’s no study behind it in a way.
[00:10:05] David: Exactly. It’s data which, you know, it’s interesting, you can put design on afterwards if you want to try and answer certain questions because there’s so much data. And there are scenarios where that makes sense. But the whole point is that this is not what traditional statisticians would consider, a design study. It’s routinely collected data, which then becomes big data quite quickly and where there’s a wealth of information which has been shown to have value.
And this is the sort of thing that actually you can reduce waste and you can observe patterns and predict behaviours by analysing this data in different ways. And it’s then led to advances in marketing and all sorts of other things. And that’s why many supermarkets provide such great incentives to make sure that you’re the cardholder, because it’s so different to have that data.
In some sense, you have the data at the level of a shop anyway. That data is going through the system. But having that data associated to individuals is so much more valuable. And that’s also an important element of understanding.
These are interesting elements of data literacy. We’ve gone away a little bit from data literacy in some sense. But I suppose the point is that actually understanding that the data you have, its value changes depending on the nature of that data. This is a really good example to understand that. If you only know what happens at the level of the shop, then that data is useful and it can help you in certain ways.
But if you can now track that to individuals, so you understand the individual behaviour within that data, then you can also understand patterns about which shops are they going to? Would it matter if, let’s say, you shut down one of your convenience stores, or if you opened another one? These are sort of things where unless you have the individual data, it’s much harder to have some of those things because you don’t have those individual patterns in the same way.
There’s all sorts of advantages to being able to actually get the individual behaviour. And actually in some of the other work, I mean, you know George well, he did his first episode.
[00:12:34] Lily: He told me.
[00:12:35] David: Yeah. His work is really also related to this element that you have these complex systems and being able to model individual insects or individuals within a system is really important. So this is also about that from the flip side of understanding the data. If you have the data on the individuals, more than just at a higher level, your understanding is totally different and you can then modify behaviour in ways which are much better.
When I say better, it’s a difficult word because better for who? This is a difficult question. But the sort of modelling he does is really related to this sort of multi level aspect of that.
[00:13:15] Lily: Yeah, interesting. And so there’s this power of having the data at this individual level, and then we can also have it at the kind of, shop level.
[00:13:25] David: Yeah.
[00:13:25] Lily: And then I guess across all the shops, or the region level?
[00:13:30] David: Yeah, I mean you might have all the shops that relate to a particular distribution point, that might be a sensible next level up. And then you might have a national level, of course, and you know some of the supermarkets are international, but that multiple level data where you’re getting data at these different levels, that’s so much more valuable than data at just a single level.
[00:13:48] Lily: So going back to data literacy in these courses, multi level data when I was taught it, five years ago or whatever, maybe a little bit more, in the last 10 years, was not something that was taught in a second or third year of an undergrad. It was something that’s kind of a…
[00:14:03] David: Advanced topic.
[00:14:05] Lily: Very advanced, whereas you and Roger are both very keen for this to become more in the introductory side, like more in our introduction to data. And I guess my question is firstly, why is that? But secondly, why isn’t it? Why is it not currently taught as…?
[00:14:22] David: If you think about when you talk to statisticians or data scientists about multi level data, they consider it as something advanced, because the mathematics behind it does get pretty hairy, it does get trickier to actually understand, to model variability which is happening at multiple levels, and to recognize that you could have variability which is emanating from different sources.
Let me just explain what that means. I’ll explain it in another context which is similar, but schools. With schools, pupils are different from each other, teachers are different from each other. You know, there’s a pupil effect, there’s a teacher effect, there’s a school effect, because each environment the school provides are different from each other, and that will affect the performance.
And so if you are looking at student performance, you’ve got variability coming in from these multiple layers. The differences between students, the differences between teachers, the differences between the school environment they’re in and so on. And what do you need to change? Do you need to change the pupils? Do you need to do something about the teachers? Do you need to do something about the schools? What are you trying to do? What can you affect? What’s within your control and what’s not within your control? If you’re working at, let’s say, a governmental level, trying to improve performance in your country, then this is a complex problem.
And the mathematics behind modelling that to try and disentangle these sort of sources of variability, mathematically this is a challenging problem. However, if on the other hand, we’re not thinking about the mathematics behind it, we’re just thinking about the fact that actually we want people to be engaging with data, it’s very natural to think pupils are different, teachers are different, schools are different. This is obvious.
So if we start our whole education suppressing what people already know as being obvious, because it’s mathematically challenging, we’re in some sense creating these sorts of barriers that people are getting. We’re presenting everything as if there was only one source of variability. I still remember this very recently, I was talking to mathematicians that are deeply involved in cutting edge advances in data science, in machine learning, and one of them asked me, you do a lot of work actually using data, we’ve had this discussion recently about error terms. We broadly agreed that because of the maths of what we’re doing, we’re just going to have one error term. But is that sensible?
These are the latest cutting edge advances happening in machine learning, making decisions about something which, from a real world perspective, is demonstrably that’s putting you into a really narrow source of applications. Most applications have multiple variability at multiple levels and therefore multiple error terms. And yet some of these people at the forefront of data science and machine learning, they are making decisions to just focus on the mathematics of single error term systems, because that’s what’s doable, that’s what’s achievable for them.
[00:17:33] David: But it’s just, it’s only applicable in very small, very narrow contexts. This is not about those advances. We don’t need the most advanced stuff for most people. Now don’t get me wrong, I’m really excited by the advances happening, and how could I not be? They’re going into algebraic geometry, they’re into my fields.
But most people engaging with data don’t need to worry about the cutting edge stuff. They need to come back and they need to understand that actually data is not a big scary thing. It’s everything around us. It’s something where variability and understanding where variability is coming from, where there’s, in some sense variability which can be accounted for, and therefore maybe even affected by interventions or by doing things differently, and where there’s variability which is just natural and that’s something you just have to accept and live with.
And so being able to distinguish between these and to actually build our knowledge up not trying to live in certainty because that’s another thing which leads to sometimes quite extreme views. Because we’re very confident and certain about what we know, whereas the more you see data in the word, the more you recognize that there’s a whole range of different things, there’s natural variability alongside variability which can be affected by what interventions we put in place.
I don’t feel I’m explaining this very well, but the key is, if we could get elements of, I believe, this data literacy more widely, to a much wider population, then I believe it would really help people in their decision making, in understanding the sort of difficult things they are studying, or if they’re in research, or just living with and part of, just in their daily lives.
I feel it is something which could really help. I believe data literacy is one of the biggest challenges of our time, to get it so that people can understand the difference between what we can know and what we can’t know because almost all our knowledge in the world today is determined by data in some form or another. And so this is central to our societies. And yet the levels of data literacy generally, even specialists in other areas, tend to be relatively low. I’ll just give a few simple examples.
[00:20:05] Lily: Yeah, please.
[00:20:06] David: You know, we work with expert researchers in agriculture and in the social sciences. And they are often very comfortable using what are considered some quite advanced statistical techniques. But when it comes to interpreting them, their interpretation is often limited to just whether or not this is something which has a proven effect or not, it’s statistically significant or not.
That’s a level of literacy which is already, compared to the sort of general population, pretty advanced. But it’s really interesting that amongst those researchers, when digging in and discussing with them what does statistical literacy actually mean to you? Statistical significance, sorry. What does statistical significance actually mean to you? Statistical significance is what means that the effect that they’ve observed is real. And that’s true. But another way of framing it, which I think they don’t recognize, is that the data that you have is sufficient to show that the effect that you have observed is real.
And so really, fundamentally, the only question it’s really answering is whether or not you have enough data to show that this difference is likely to be real. And why is that important? That’s really important because in many of these contexts, they’re now entering into situations where they have access to lots of data where they can do things at a bigger scale.
And the question of whether it’s statistically significant or not becomes less important if you have a big study, if you have lots of data. There’s other questions which become more important than statistical significance. And what you’re wanting to then do is you want to then dig into understanding the data that you’re looking at. And so data visualisation becomes a much more important tool in your arsenal.
And this is something where understanding that because so much of the training has been about scenarios where you have very little data, a lot of the research we look at is born, the methods are born in contexts where we don’t have enough data. I like to talk about data scarcity. The era of data scarcity is where a lot of the research methods that are being used for research all over the world, and this is what’s expected by journals and academic publishers. That’s absolutely valid, but it’s putting so much emphasis on asking the question, do we have enough data?
Whereas actually in the world we live in now, we’re entering this amazing world of data abundance. And what that does for the research questions, what that could do for research is immense. But it would require a whole set of literacy, data literacy, to be shared at all levels of our academic sort of infrastructure from the publishers all the way down.
Because if the publishers are only publishing things which relate to statistical significance, then they’ve not understood, of course, if you’ve got lots of data, then you’re going to get statistical significance, but that’s not what’s important. And so actually being able to have deep data literacy, where you’re understanding what the different, or what you can learn from data in different ways, in different contexts, when you have more data, when you have less data, when you’re able to design a study, when you’re not able to design a study, this is what’s so important.
And it’s not that difficult because very simply, there’s only one way to actually get evidence of causation, and that is to have an experimental design where you can be confident the only source that it will explain the variability observed is the treatment, and therefore the difference that you put in. And then you can genuinely say yes, we have evidence that this difference causes this effect. And so you can actually determine causation.
If you don’t have experimental design to support that and to enable that, then you can hypothesise causation. You can do all sorts of other things, but you can’t actually determine it. And this is where, if we actually dig into this, and it’s not so difficult, there’s some simple principles. Instead of focusing on the calculations behind it, those aren’t important. What we need to think about and what we need to get towards is what are the principles?
And this is where I think thinking about data literacy in terms of building understanding of some of the principles of how we work with data, some of the skills you can gain when you’re observing data, this is something I believe this can be done at a much bigger scale than it’s currently being done. And I would love it if we could actually build from the work you’ve already started. And I know this is something you’re passionate about, so I’m preaching to the choir here. I’m conscious that you’ve started trying to build these. And I think you’ve been surprised when you’ve actually got into sort of building some of these courses at what’s needed, what’s useful and, how people are reacting to it.
[00:25:45] Lily: Yeah, no, absolutely. Yeah, very surprised at what’s… I guess just surprised. And this kind of goes back to the stuff when I said about it being, I didn’t say simple, I didn’t say simple, but what I said at the start about it not being hard, not being as hard as you think it’s going to be. Because actually when you take away, when you strip away all of this kind of P values and calculations and okay which kind of ANOVA do I, you know, when you strip away all of that, it’s actually just a simple question.
When it’s about, okay well, I’ve got this data, so let’s just go through it. Then you can… I’m not phrasing this very well. I suppose it’s like with these p values, as you were saying, and just seeing, oh, actually significance testing, as you say, it’s just about, do I have enough data? Is there enough data to show that there is an effect? And when you just realise that’s all it is, that’s all that’s happening here, that’s when it becomes quite liberating in a way.
[00:26:49] David: Let me try and rephrase what I think you’re saying, because I think what you’re trying to say is really powerful. What you’re saying is, if you think about, if you want to get the p value, if that’s your aim and that’s your goal, then it is hard, because what assumptions are behind the p value you’re getting?
[00:27:06] Lily: Yes.
[00:27:06] David: Are you using a parametric test? If you’re using a parametric test, what are the assumptions that need to be satisfied? Are they satisfied? If they’re not satisfied, what should I do about it? In all these different cases, can I be confident in the result? And it is hard.
[00:27:21] Lily: And even there saying, parametric test, that, that sounds so scary and confusing, but that’s just saying, okay, can we assume, does it follow a distribution? That’s it.
[00:27:28] David: Exactly. And if you can’t, then you need to take a non parametric test, and that’s weaker in different ways, but there’s so much to know, and so confusing, all these different things. Am I doing the right thing? Am I doing the wrong thing?
[00:27:39] Lily: Yes.
[00:27:39] David: But if you know that all that’s going to tell you, after you’ve done all that, is whether you have enough data or not, then you can make a value judgement and say, okay, I seem to have enough data to start with, so let me actually understand what it’s telling me to know if it’s worth getting some help to know if I need to check whether I’ve got enough data or not.
And then you can bring in a statistician who’s a specialist at doing that. That’s a very simple example if you’re worried about having enough data or not. But of course, many people are now not worried about having enough data because they’re in a context where they’ve got lots of data.
And there again, do you need to get in someone who’s going to do the machine learning on it? And yes, you might need to, to be able to sort of identify things you haven’t been able to capture. But most things you should be able to visualise. The importance of looking at your data, getting a feel for your data. I had some students a while back, PhD students, really good, really competent people, who were given some data, and they, that was the dog, wasn’t it?
[00:28:44] Lily: That was the dog and he’s still going, so I’ll put myself on mute.
[00:28:47] David: So basically they had this data, they got given this data, and it was quite a lot of data in different ways, and it was quite difficult, and they said, oh, we could use this machine learning algorithm to try and classify it, and identify the categories.
And I said, you can try if you want. And they then spent days and days working on it. And after that, they then came out and said, we’ve done it. And as it turned out they had managed to find out, this was looking at data on lots of different varieties. They had managed to find out that short season varieties were different from long season varieties. And that’s all they’d found out. And we already knew that. They’d used really powerful techniques, saying for a long time, trying to do it right in different ways. And all they’d found out is what was already known about the data.
So it’s not about the techniques. Now, of course, this is something where I actually know really nice instances where, going back in time, some of the very early data scientists were working with people on this and they had a similar sort of thing where the first time they went back to them, they found out something where they already knew it. The second time, the same thing. And the third time they went back with something and the scientists said, really? No. Ooh, that’s interesting. We didn’t know that. And it led to huge advances.
And so the fact that you’re finding things which are known that’s good. That’s reassuring.
[00:30:08] Lily: Yeah.
[00:30:08] David: And then the fact that actually when you dig in, you might find something which isn’t known, this is exactly where the power of big data comes. This wasn’t surprising to me at all. It’s just, it puts into perspective, we need the importance of the methods and the fact that these aren’t magic. They’re just identifying trends in the data or features in the data, patterns in the data. And we need people to recognize and value them for what they are. Which are extremely powerful tools, which can really help, but where we should have non specialists able to interact and understand what the data is actually able to tell us or not, even if they don’t know which test do I actually have to use to be confident that I have enough data to be sure that what I’m observing is really happening?
That you don’t need, that can get hard.
[00:31:04] Lily: Yeah.
[00:31:05] David: And so that can be passed on. Similarly, elements of the deep machine learning with big amounts of data can be passed on when you’re doing it with lots of data. But the understanding that what all you’re doing there really, is trying to find the patterns and the structure in the data, and so actually looking for patterns and structure in smaller data sets, even in subsets of big data, and being able to see, is this sensible? Is this what we’re looking for? That’s the data literacy everybody should be having at their fingertips.
And data is so abundant nowadays. This should be almost a universal skill. And I guess the thing I’d like to finish on is I want to shout out to New Zealand.
[00:31:48] Lily: I was wondering when New Zealand was going to come in. I jotted this one down.
[00:31:51] David: Of course, New Zealand is the one country that I know have now for, I believe it’s almost 20 years, it’s certainly over 15 years, they’ve had from the first year of primary right the way through school, a whole separate curriculum on data. And don’t get me wrong, implementation has not been perfect and they’ve learnt from this, but they are way ahead of anyone else I know on trying to get this embedded in the population at scale.
And in doing that, they’ve made decisions, which, when I first heard them, I thought were ridiculous. And the more I’ve learned, and the more I’ve seen, I’ve come to appreciate them, I still don’t agree necessarily with all of them, but I see why they’ve taken the decisions they’ve done, and I think it’s inspirational. It’s so exciting. I really want to learn more about that and I wish more countries would be looking to New Zealand to see how can we change our curriculums at school to make data literacy its own thing, right the way through schooling.
[00:32:57] Lily: I was gonna ask it as a question, but then I thought this probably warrants its own podcast, is data maths still how things are going?
[00:33:06] David: That has to be another episode.
[00:33:08] Lily: Yes.
[00:33:08] David: We can’t do that in this one, because if you get me started on that, that’s going to be a whole nother episode, but let’s do that, let’s do that episode. That episode will come out shortly. I look forward to that, because it’s one that I have really, I’ve got a really ambitious idea on this. So I’m going to present that in another episode.
[00:33:26] Lily: Perfect.
[00:33:29] David: I’m itching to do that now, but we’re out of time.
[00:33:31] Lily: We’re out of time. Thank you very much, David.
[00:33:35] David: Thank you.