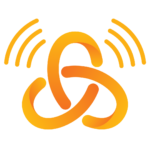
Description
Lucie and David discuss various forms of study, including opportunistic studies, surveys, questionnaires and experiments. They consider some best practices for good research, using anthropology and agroecology as examples. How might modern data science be able to employ existing data for new opportunistic studies? And who might typically be excluded from data as it is often collected?
[00:00:00] Lucie: Hi and welcome to the IDEMS podcast. I am Lucie Hazelgrove Planel, an anthropologist and social impact scientist, and I’m here today with David Stern, a founding director of IDEMS. Hi David.
[00:00:18] David: Hi Lucie, looking forward to another discussion. What’s it going to be about?
[00:00:23] Lucie: So, I wanted to dig into really what research is or how one can do research. When I joined IDEMS, I think several people, both from IDEMS and our partner, Stats4SD, have corrected me about whether I use the word survey or study or questionnaire. So, this has got me wondering
[00:00:46] David: A questionnaire is a tool to collect data. It can be used in many different contexts as part of many different types of study. And so, it’s not in its own right as, study type. So you could use this as part of an experiment where your data is collected using a questionnaire but you are doing an experiment because you have different treatments.
[00:01:12] Lucie: Right, so I’ve heard of this a lot now that I’ve started working with agroecologists, that many of our research colleagues do agricultural experiments.
[00:01:21] David: Yes.
[00:01:22] Lucie: Now in anthropology I wasn’t really used to people doing experiments and it means a lot in agriculture, doesn’t it?
[00:01:28] David: Absolutely. I mean experimental methods, these go back a long way. My understanding is the very first experiment was related to scurvy on ships.
[00:01:40] Lucie: Ah, interesting.
[00:01:41] David: That basically they gave different treatments to different sailors and saw who got ill, give or take, and you know, at that point, there wasn’t much which was understood about this in different ways, and so this was a first attempt at actually formalising that and keeping everything else the same, but just having one thing which changed, and that’s the treatment. That’s the experimental method, which has now been really developed out and it can be used in all sorts of contexts.
[00:02:12] Lucie: And it’s really to identify the cause of something, it’s to identify what creates the change.
[00:02:18] David: Absolutely. You can only establish causation through experimentation. You can hypothesize it in many other ways, with many other types of study. But to get rigorous scientific evidence for causation, the only method we currently have is experimentation.
[00:02:38] Lucie: Do you always need a control in an experiment?
[00:02:42] David: That’s a really loaded question, because of course, randomised control trials have become very popular, this led to a Nobel Prize, Esther Duflo is the second female economist to win the Nobel Prize, and she got it for her work on randomised control trials, which was to take broadly the experimental method in a very, precise form, which is coming out of, if you want, medical research, and applying that into the development context and into a more social context. And of course, control is central to that. But of course, control is really rather misunderstood.
[00:03:25] Lucie: Well, my follow up question would have been, well, what is a control? What ways, like, are there different ways of having a control?
[00:03:30] David: Of course, you can have a positive control and you can have a negative control. In many cases, for example, when I’ve been working with agriculturalists in looking at elements of fertilization, they tend to say, okay, so for the control, I’ll just use nothing. I say, well, wait a second, your farmers don’t like using nothing. So if you do this in a farmer’s field and you just use nothing, then maybe that’s not the right control for you to be using.
What’s their best current practice? If their best current practice is to use fertiliser, and you’re wanting to say, well, you could actually save money by replacing that bought fertiliser with sort of some form of homemade fertiliser then using nothing as your control isn’t useful because you’re not comparing the right things.
So you could consider the standard recommended dose of bought fertilizer as a positive control, nothing would be essentially a negative control, and you could then compare. And there are experiments which have two controls, a positive and a negative control, and those can be very powerful.
So the concept of control is really misleading, and I’ve seen this in too many cases, with, if you want, public health type studies, where the expectation is that the control is nothing, and therefore you’re not actually doing what I would consider a really useful comparison.
The heart of control is about comparison. What are you comparing to? And so you want to compare to something which has meaning to you. In the medical context, your control would be your best current treatment. That would be the right control. And it’s not necessarily easy to define in all contexts.
[00:05:26] Lucie: No.
[00:05:26] David: While control is a really powerful and important concept and very important to experimentation, sometimes people misunderstand it for nothing and then it’s not a useful concept. So I would rather people don’t think about having a control rather than to have nothing. If they think control is nothing, then it’s not useful, they shouldn’t be having controls.
They should be thinking much more about what are they wanting to compare and why. And that’s where, often, if you do that well, you get to a sensible control, something you’re comparing to. But it’s rather difficult in different ways if you just think of your control as nothing.
[00:06:13] Lucie: That’s really interesting. Okay. Now, so experiments are the most complicated type of study, right?
[00:06:18] David: Complicated? Not necessarily. I mean, in many ways they’re the simplest, because in an experiment you need to have your treatments. A good experiment, your treatments are such that the comparison between things is very simple.
So everything else stays the same, you’re removing as much complexity as you can, and you’re just comparing things which are comparable. I’ll give a very simple example of where this can get very complex. There’s some wonderful work around, let’s say, if you have multiple crops, where you’re intercropping them.
[00:06:53] Lucie: Yeah?
[00:06:53] David: You’re wanting to think about the intercroppings.
[00:06:57] Lucie: And intercropping is where you’re growing two things side by side, isn’t it?
[00:07:00] David: For example, that’s one way to intercrop, but actually there are some places where the intercropping happens in the same pocket. So you put seeds of multiple things in the same pocket.
[00:07:08] Lucie: Ok.
[00:07:09] David: And then the different plants grow from the same place.
[00:07:11] Lucie: Like I think sort of maize coming up and then green beans growing up the maize plants.
[00:07:15] David: For example green beans was not what I had in mind from the West African context we work in, but cowpea would be an example which works there. [Laughs]. But anyway, so the point would be, you know, you have multiple ways of intercropping and you have multiple varieties of maize and you have multiple varieties of cowpea, let’s say.
And now of course you get to this really interesting thing where, if you look at these and you look at them in certain ways, then you kind of get what you set up to get. There are many varieties of cowpea and maize, which aren’t used to being put in the same pocket together. And they’re used to be grown separately, and so if you do those in line, they grow very nicely, and they outperform varieties which are used to being put into the same pocket together.
And whereas if you put them all in the same pocket together, then it’s a different combination of varieties that work well together in that context, because they’ve been selected for that. So now normally I would say, if you want to understand something, only change one thing at once. Either compare your varieties, or compare the intercropping method, or compare the variety of cowpea, or whatever it might be, or the way of fertilizing it.
But in this particular case, of course, that doesn’t work very well, because if you take, let’s call them local varieties and bred varieties, you know, made in other ways, through breeding programs. Breeding programs don’t tend to be bred using intercropping and they tend to work better when you intercrop in lines and this sort of thing because then they have the space to grow. Whereas a lot of the farmer’s seeds, in certain places, they do this intercropping in the same pocket. And so, in that context, they grow really well together. They build a symbiotic relationship. They’ve developed that over time because of their selection process.
So, you can’t just do single comparisons. But if you can’t do single comparisons, I don’t know how to do a good experiment. And really, it becomes very complicated. What are you actually comparing? So these sorts of things where, in theory, actually, experiments don’t do well when they’re complicated.
Experiments do well when you can get to something simple. So in that particular context, it’s really hard to do a good experiment to compare those two things, because there’s so many different things, which is sort of symbiotic to each other, that complexity makes experimentation almost impossible to ask good questions.
So really experimentation is in some ways the most rigorous, but it works best in simplicity when you have simple comparison questions.
[00:10:10] Lucie: And that takes a lot of thought and it takes a lot of design then.
[00:10:13] David: Exactly, yes, it’s the most designed, you know, you can’t really do good experimentation without good design. These two go hand in hand, and even with good design, you can actually often get, if you take that example of the intercropping, you know, broadly what you learn is that, well, the things that particular varieties were bred for is where they do better. Well, you kind of knew that beforehand.
So what are you learning from the experiment? What comparison are you making which is useful there? That would be a really good example where I don’t know what the right questions are to ask. Is the question how should you be doing the intercropping?
But that depends on which varieties you’re using. You could ask a question which would be simpler for these varieties, how are they best adapted to intercropping? That would be a question which I could design an experiment to answer. But if you start adding the layers of complexity which farmers have, it becomes really hard.
[00:11:15] Lucie: That’s absolutely fascinating and a good example of the complexity of designing a simple experiment perhaps.
[00:11:22] David: Yes, exactly. And this is the thing, so experimental design, people put experimentation on a pedestal, because this is the only thing you can do to actually get sort of real attribution of cause to say, yes, the only thing different between these two is this one treatment effect, everything else I controlled for, or I measured, it was the same. And that one difference led to this difference in observed effect. And that, therefore, is evidence, scientific evidence, of a causal relationship.
But often it’s only useful if you actually already have a pretty good hypothesis of what that causal relationship is, and an open question about the fact that, is it really there or not? And this is where, in the medical world, this is so effective, because you’ve done a lot of research before you get to the experiment to check, does it actually have the effect you’re expecting in the way you’re expecting it in the real world. And that’s where these randomized control trials are extremely powerful at that. And, of course, in the medical context, they have to be double blinded.
[00:12:35] Lucie: Yes, I was thinking of that.
[00:12:38] David: If you don’t double blind them, then you get the placebo effect on one hand, and you get an effect because if the doctor believes that what they’ve given you works, actually, that has an effect as well. So, double blinding is essential. Now, you can’t get double blinding in almost any other context that I know, except red pill blue pill type contexts.
[00:12:57] Lucie: Yeah.
[00:12:58] David: And so, the importance of double blinding cannot be overstated and there’s been such fantastic studies demonstrating the power of the placebo effect that this is something where we can’t underestimate the value of the blinding within a good randomized controlled trial.
So, in the context where you can’t have double blinding, it does lead to questions, well what other elements of experimentation, what other design methodologies might help? Because it’s not the only way, there’s crossover designs which are really powerful, where you give the same treatments to both but you do it in a different order.
And then you get some really interesting other data because you actually get some of the longer term effect or the comparisons in another way. Crossover designs are great fun and really can be very powerful as well.
[00:13:51] Lucie: But that makes the analysis and interpretation a lot harder?
[00:13:54] David: Well, it means it’s more expensive, because everybody needs to get the treatment eventually, and in some cases it’s not ethical. I mean, it’s not ethical to take someone off an effective medical treatment, so there’s all sorts of ethical issues with these in different contexts.
In the educational context, crossover designs are well established and they are recognised as being very powerful. And often in that context, the additional cost is not that much. And so very often I would argue that in an educational context, a good crossover design is very cost effective.
You’ve actually got all the setup to do it and there’s many ethical advantages with that approach in that context. So, it really does depend, we shouldn’t be putting methodologies on a pedestal as being the best. Different methodologies work in different contexts, in different situations for different studies. And I suppose we’ve discussed experimentation quite extensively, but experimentation is, in my mind, no better than other studies.
You’ve actually helped me to get the language on this. So when we discussed this and put this into a course recently, we were able to call this distinction of really three levels of: observations, opportunistic studies…
[00:15:13] Lucie: Yeah.
[00:15:14] David: Surveys, if you want, and experiments. And so experiments, we’ve discussed at length, they are the only methodology that can lead to actual scientific evidence of causation. Whereas both survey and opportunistic studies can only hypothesize causation. And this is something where, really, as a language, this is not well established. This is really your language, which I love, and so I’m repeating and stealing.
[00:15:53] Lucie: It was a collaboration!
[00:15:55] David: It was a collaboration.
[00:15:56] Lucie: I wasn’t aware of these different types. I hadn’t thought about different types of studies across disciplines too. This is what I find so interesting about this, is that it works across disciplines and it can be applicable too. As you said, I think, before the different study types aren’t hierarchically different and they’re not necessarily independent. They can be added to each other. They are complementary sometimes.
[00:16:19] David: They can be complementary to one another. Yes, absolutely. Good research would often involve elements of different study types. I absolutely agree. And I would not say that one study type is better than another, but I would say that there are sufficient differences between these three universally across areas that it’s a useful distinction to have in your armoury of thinking about evidence.
So we’ve got the distinction between experimentation, and opportunistic versus survey.
[00:16:49] Lucie: Yeah.
[00:16:51] David: The boundary between opportunistic and survey, I’d argue is a little bit more fluid.
[00:16:56] Lucie: Yeah.
[00:16:56] David: But I think it’s hard enough. There is a hard boundary in some sense, which I would really attribute to being able to design your data, design the data you are working with in some sense. So really have rigorous design principles for the data.
[00:17:17] Lucie: Sorry, can I just ask, there you’re saying designing the data as opposed to designing how you’re going to get that data?
[00:17:25] David: Good question. I… I don’t know. I mean, broadly, yes, designing how you’re going to get the data. Many opportunistic studies include all the amazing work which is now happening in data science, where you have big data, where you have lots of data which is coming in and you’re almost flooded by the data, and you’re now trying to find ways to use that data to make conclusions, to understand, to be able to get knowledge out of it, to build understanding from it.
It’s extremely powerful. You don’t control that influx of data in that case. There’s other opportunistic studies. You know this as well from an anthropological side, where there’s this idea of actually really being very careful at designing what you’re trying to find out before you go into a community for study, or going in with a much more open mind and being more opportunistic with who you follow, what you’re looking for, what you’re doing.
And so that distinction is there as well. And I think in the anthropological setting, which you know well, it’s not clear to me where that line would be in the same way as it is where I’m thinking of a well defined survey with a sampling method, which means that you know what population is represented by the data you have versus data which is coming up at scale, which maybe represents more people, but you don’t actually know exactly who it represents because of the nature of the data and the way it’s come in.
And so in some ways, in a survey, you may know better who the survey represents. It may be better if you know exactly who you’re trying to represent. Whereas you may gain more insights from having the big data which comes in, but there may be more biases in that. And I would argue that in many ways, the protection against bias is a big part of what you get from the design aspect of surveys.
[00:19:27] Lucie: Yep.
[00:19:28] David: So that’s really a lot of what you’re trying to do. And this is where I find it very interesting that in contexts where people have fallen in love with data science and the power of big data, I’m surprised that people aren’t more scared by the potential biases. That this is something that I absolutely see the value of big data but I’m always worried about, well, whose voice is not being heard? Whose data is not there? Who’s not represented? Is somebody’s voice over represented? Are there certain perspectives or views which are over represented in that data? And because of the nature of where the data’s come from, whose voice is being heard? And often we don’t know, there’s elements of this which I feel are very exciting because this is sort of early days. I mean, a century ago, the concept of big data was totally non existent. Even in the 90s, when a lot of the methods that are now being used really quite powerfully on this, the orders of magnitude of data that was available was such a tiny fraction of what we have nowadays. Data is omnipresent in our current world. And to be able to use that constructively What an exciting opportunity!
[00:20:53] Lucie: And that’s sort of an open challenge to the agroecological researchers that we work with. Can they use existing data in an opportunistic manner, perhaps?
[00:21:02] David: Absolutely. And can we change the way we think about data and collect data and work with data so that we can gain learning in other ways.
[00:21:16] Lucie: Yeah.
[00:21:17] David: There’s going to be, I hope, over the next, I don’t know, I guess it’ll take longer than I expect so I’ll say 20 years. It might be less, but over the next 20 years or so, I would expect that the people who are currently excited about the power of big data and what it can do may find that they become a bit more cautious. And the people who are currently too cautious and believe everything should be designed and so on, my hope is that they will embrace the big data and what could be learned from it, and learn how to bring their expertise on that. Because I feel that at the moment, we’ve got elements of opportunity which are not being seized, and where people are running away in ways which are in some cases irresponsible.
Lily and I have been discussing a number of these in the Responsible AI podcasts, and there’s real issues around this. People using big data irresponsibly, but the opportunity that comes from the data that’s available… I want to come back to the fact that opportunistic studies are not just big data.
[00:22:30] Lucie: No, exactly. I was going to mention, for example, the MICS surveys the Multiple Index Cluster Surveys, is that right, by UNICEF, for example? In terms of people working in agriculture, the benefits of understanding households and how they’re composed, can be really interesting to look at.
[00:22:46] David: Absolutely. And this is a fantastic example of survey data. So this is real survey data. But it could also be used opportunistically for other things.
[00:22:58] Lucie: And that sounds confusing.
[00:23:00] David: It is confusing, I’m sorry.
[00:23:01] Lucie: We’ve just said that there’s three types of study. One’s opportunistic, two’s survey, and three’s experiments. But now you’re saying that surveys can be used opportunistically.
[00:23:10] David: The data from surveys.
[00:23:11] Lucie: Right.
[00:23:12] David: So this is the key thing. It was a well designed survey. Census data, maybe that’s another example.
[00:23:19] Lucie: Yeah.
[00:23:19] David: You know census data, really well designed, comprehensive, but you could use it opportunistically in an opportunistic study. So this is the key thing, that it’s types of study, not types of data. If you get into types of data, then there’s other types of data as well, and so, you know, people talk about routinely collected data, I don’t want to get lost into that, because I think types of study is enough, that actually if you think about designing your study, really a lot of this is, well, are you going to be opportunistic, using data which is either already there, or where you have an opportunity to get information? Another example of an opportunistic study could be observation studies.
[00:24:12] Lucie: Well, exactly. So to me as an anthropologist, being opportunistic is the sort of taking the opportunity to go to an event that you didn’t know was going to happen, that you couldn’t have planned for but you can have planned. So most anthropological fieldwork is a survey in type, because it’s understanding the general situation, the overall sort of, whether it’s a very small situation of one household or whether it’s the biggest situation of a village or a community in a city. But then you can design, by design you can be opportunistic.
[00:24:47] David: Well, exactly. And I would argue that part of the question is in that design, are you trying to be representative of something? If you’re not, then I would argue that’s just opportunistic. You’re wanting to learn about this particular family, not because this family represents families of this particular type, or this particular genre, you know, or this community, but because it’s interesting and you can learn from observing them, and that’s an opportunistic study.
A survey is almost always trying to be representative of something more than you are collecting.
[00:25:22] Lucie: Okay.
[00:25:23] David: So if you’re thinking about doing an anthropological study of a particular culture or a particular community, then there’s almost certainly elements of survey within it where you’re trying to make sure that you’ve got representation within that community to understand the structures of the community to be able to get that. That’s part of how you design your anthropological study.
But, you know, there are other opportunities where you’re not trying to actually represent anything more than the people you studied. They are not saying these people represent anything more. And that would be to me the real difference between a well designed opportunistic study and a well designed survey. A well designed survey should be representative of something more.
And that’s why you’re doing the design. And sampling is key, randomized sampling is key to being able to be representative of something more. And so that sort of, you know, if you think about your randomized control trial, your treatment and your control, that’s part of the experimentation. Your randomization, that’s already there in a survey because that’s what allows you to represent something more in those individuals.
[00:26:39] Lucie: So I’m just coming back to the anthropological side of this. So I think often if you’re a person going and trying to communicate with other people, the family, if you’re going to work with the family, the family you work with, it’s not necessarily you who chooses them, it’s them who choose you.
So that side is perhaps opportunistic, but then when you’re writing it up, the anthropologist will always sort of try and give an understanding of how that family is perhaps different to other families in order for the reader or whoever is engaging with the research to try and understand what biases might be present.
[00:27:11] David: So I would argue what you’ve described there is very much an opportunistic study. And what you’re trying to do is, in an opportunistic study, you need to try and recognize and understand the potential biases. And that’s exactly what you’re describing needs to be done, just as if you were to get big data, you should try and understand the biases, and there are tools to try and do this.
And by trying to understand the biases, you can then hopefully remove some of them from the results that you project and describe. But if you are looking at instead of studying a family, studying a community, that’s when my guess is that you’d actually, as an anthropologist, the methods you’d use would actually be probably closer to what I would define as a survey, because within that you would have choices about who you’re interacting and who you’re spending time with within that.
In a sort of survey language, you’d be doing the equivalent of stratification in terms of your sampling. You’d try to make sure you talk to people who are different, who have different roles within the society. That’s stratification within a survey design process. And so that idea of stratifying for your sampling, you know, that’s the sort of thing where if you’re trying to understand a community, am I correct as an anthropologist, you might not call it the same thing, but you’re doing that as well, you’re identifying who are the sub communities?
[00:28:34] Lucie: But exactly, and this was one of the problem of anthropologists in the 19th century, no 18th, 20th century, sorry, where generally it was men doing research and generally they only interviewed men, even if it was about women’s issues or women’s work, which is an interesting thing to do…
[00:28:52] David: Yeah, and there’s all sorts of issues that come up about that in surveys in general. And, you know, you have all sorts of different methods. You know, a focus group. Focus group could be part of experimental data, or it could be part of your opportunistic study, or it could be part of a survey. Actually, that’s just a data collection method.
Let me take another one. Very simply if you think about a lab analyses. You can do lab analyses as part of an experiment, or you can do lab analyses as part of a survey, or you can do lab analyses as part of an opportunistic study.
[00:29:27] Lucie: If someone just happens to give you some soil to analyse, then yep, then that’s being opportunistic, but you might have decided to actually analyse that soil right from the beginning, that might be what your study is about.
[00:29:36] David: Exactly. It’s not just if they give you some soil to analyse, it’s for example, if you have a soil analysis system which is set up so that people can bring their soils in for analyses, that data could then be used as an opportunistic study data.
[00:29:52] Lucie: Yes.
[00:29:52] David: Because, you know, it’s not being sampled and there’s no survey sampling process. But there is a large amount of data coming in, which actually together you might notice, whoa, everybody’s soil is really acid here, maybe there’s a problem of soil acidity in this region. Maybe it’s just the people who brought their soils in, who brought it in because there was soil acidity. I’m using that as a random example. But, you know, this is the sort of thing where all three of these sorts of methods, there’s really powerful ways to think about this.
Let me go back to that opportunistic related to something which is coming from a farmer, let’s say. If we think about disease identification in plants, let’s say.
[00:30:37] Lucie: Plants, yeah, exactly, not of humans, plants here.
[00:30:39] David: It could be humans as well, but that’s a bit more complicated. So I’ll do with plants for now, because then you might be able to take a photo. So let’s say you got photos of people doing this. Now this isn’t a sort of a survey, because you’re not designing what this represents. But this could be incredibly powerful in terms of the data that’s collected, because this done right could then feed your AI algorithm, which could then be used in the field to help identify it on the fly, and so on.
And so these different ways of thinking about these different studies and how we want to design studies to be able to serve and build our knowledge base in different ways is, I think, really exciting. It’s something where I don’t think there’s enough recognition. I certainly didn’t have the vocabulary until you helped me get it.
I certainly had this distinction of these three things, but I didn’t have a way of describing them as sort of opportunistic, survey, experiment in the past. And I think that description is really helpful because it could allow people to value all three. Even scientifically, academically.
And I don’t think there’s enough value given to opportunistic studies. And actually that’s where being able to set up rigorous methodologies related to opportunistic studies where we worry about, if you’ve got an opportunistic study, you need to worry about biases. This is like in your anthropology, where you then think about, well, you try to observe what are the potential biases, and you try to sort of manage them, and you actually have to report on that, and how you’ve reported on them.
So that would be part of what would be needed if we established how to do opportunistic studies, rigorously, scientific, and I think there’s incredible opportunity around opportunistic studies, but more generally around thinking about these three types of studies and the value that each one brings.
[00:32:48] Lucie: Individually and how they can add to another study.
[00:32:52] David: Exactly. I gave a talk on responsible AI not so long ago to a corporate group. And one of the things that was discussed a bit is the value of polls and polling surveys, where actually you determine the mood of the nation.
[00:33:09] Lucie: Okay, that sort of poll, yep.
[00:33:10] David: Using a poll for, you know, who are you going to vote for? Using a poll which is a very small number of people that represents pretty accurately the view of a large number of people, compared to a sentiment analysis, where you have lots and lots of information, but it’s maybe not of a representative sample of the population.
The sentiment analysis, let’s say, is used to analyse tweets. Sorry, X’s. I don’t know what they’re called anymore.
[00:33:42] Lucie: X’s sounds like xisses!
[00:33:43] David: Maybe that’s what they’re supposed to be called now. Things that used to be on the platform formerly known as Twitter.
[00:33:52] Lucie: There we go.
[00:33:58] David: But, you know, if you analyse them, you can do a sentiment analysis and you can use other social media networks to do that as well, and you get a feeling for what are people feeling at this point in time towards a particular topic or in relation to something. And there’s some really good work which has been done on that. Who’s it representative of?
[00:34:18] Lucie: Yep.
[00:34:18] David: What I love about the polling methodology and how that’s got really good over the years is that they do use stratification in very powerful ways to be able to ensure that it’s actually representative of the population they want to represent.
[00:34:33] Lucie: Interesting.
[00:34:33] David: Whereas you can’t do that as easily with a sentiment analysis based on social media because some people’s voices are simply not heard, whole sections of the population. And other people’s voices are heard very loudly. It’s not that one is better than the other, they serve different purposes.
[00:34:52] Lucie: Exactly.
[00:34:53] David: And you have advantages and disadvantages of either of them. One of them is an emerging field coming up very fast, but I feel often not recognised in enough scientific disciplines the value these approaches could bring. You know, in agriculture, I almost never hear people talking about the value of opportunistic studies to be able to get large scale amounts of information and learn about systems in interesting ways, which is, I think, a real possibility.
But it’s different. And I think there’s a… Well, it’ll be interesting to see what happens as these fields develop and as the methods develop as well.
[00:35:37] Lucie: That’s a really nice place to end. So I’ve enjoyed this discussion, David. And yeah, we look forward to seeing what the future brings in terms of the study types and usage of them.
[00:35:48] David: Thank you.